Better Model, Worse Predictions: The Dangers in Student Model Comparisons
ARTIFICIAL INTELLIGENCE IN EDUCATION (AIED 2021), PT I(2021)
摘要
The additive factor model is a widely used tool for analyzing educational data, yet it is often used as an off-the-shelf solution without considering implementation details. A common practice is to compare multiple additive factor models, choose the one with the best predictive accuracy, and interpret the parameters of the model as evidence of student learning. In this work, we use simulated data to show that in certain situations, this approach can lead to misleading results. Specifically, we show how student skill distribution affects estimates of other model parameters.
更多查看译文
关键词
Additive factor model, Student modeling, Simulation, Model comparison
AI 理解论文
溯源树
样例
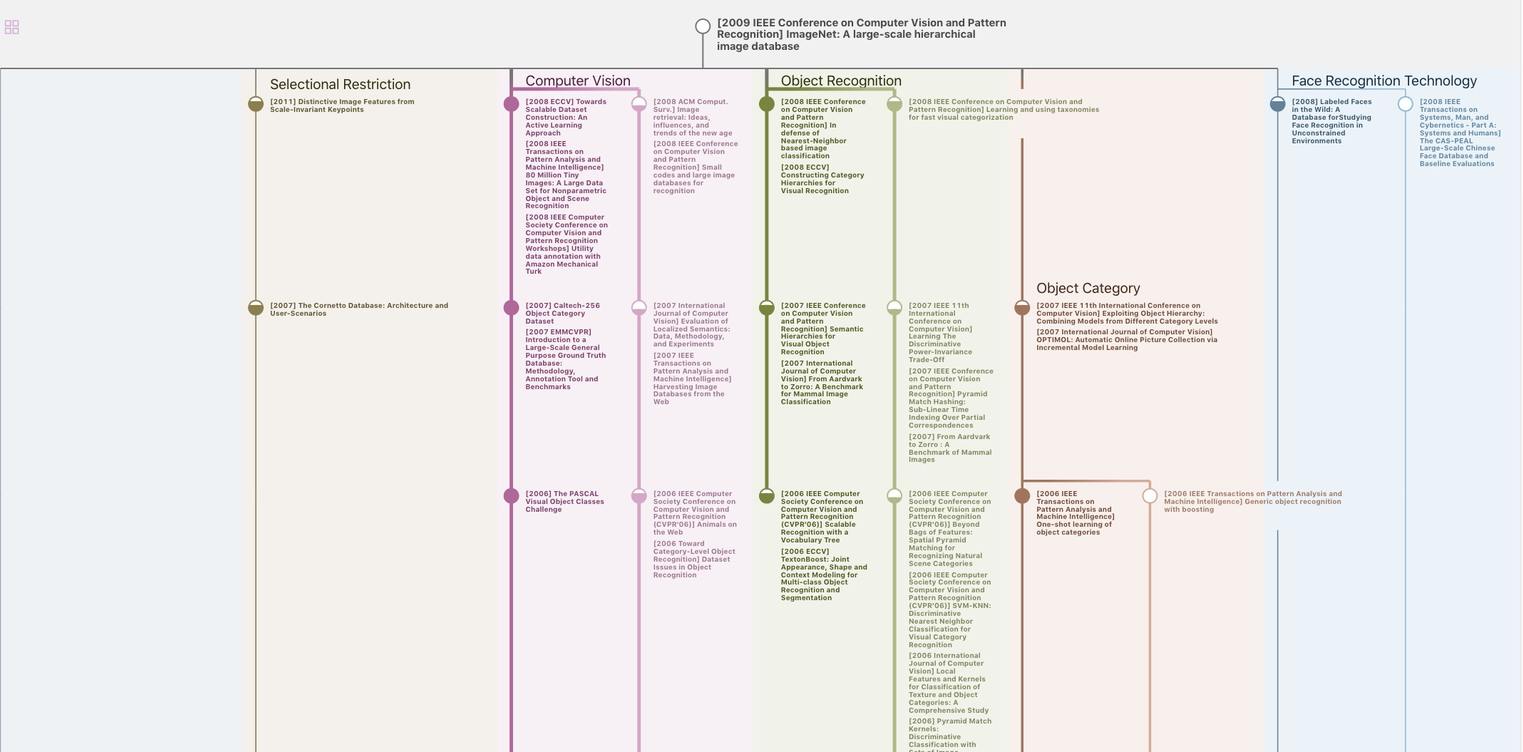
生成溯源树,研究论文发展脉络
Chat Paper
正在生成论文摘要