Transfer Learning assisted PodNet for Stimulation Frequency Detection in Steady state visually evoked potential-based BCI Spellers
BRAIN-COMPUTER INTERFACES(2023)
Abstract
Convolutional Neural Networks (DCNNs) can be a useful tool for detecting the stimulus frequency of the SSVEP signal. The transfer learning approach is used to improve the performance of the PodNet in the presence of a low amount of training data. In this research, two publicly available, 35-subject Benchmark and 70-subject BETA databases are used. In the rendered method, information is transferred from a model trained on the large BETA database to a secondary model which has been designed to identify target stimulus frequency in single-participant of the Benchmark database. The results show that the accuracy (95.00 %) and ITR (143.13 bpm) of the proposed approach in single-participant are significantly higher than the CCA (p < 0.05) and the PodNet (p < 0.00001). This study illustrates that using the transfer learning approach can improve the performance of the PodNet in the case of limited training data.
MoreTranslated text
Key words
Brain-Computer interface speller systems,deep convolutional neural network,transfer learning approach,electroencephalography,steady state visual evoked potential
AI Read Science
Must-Reading Tree
Example
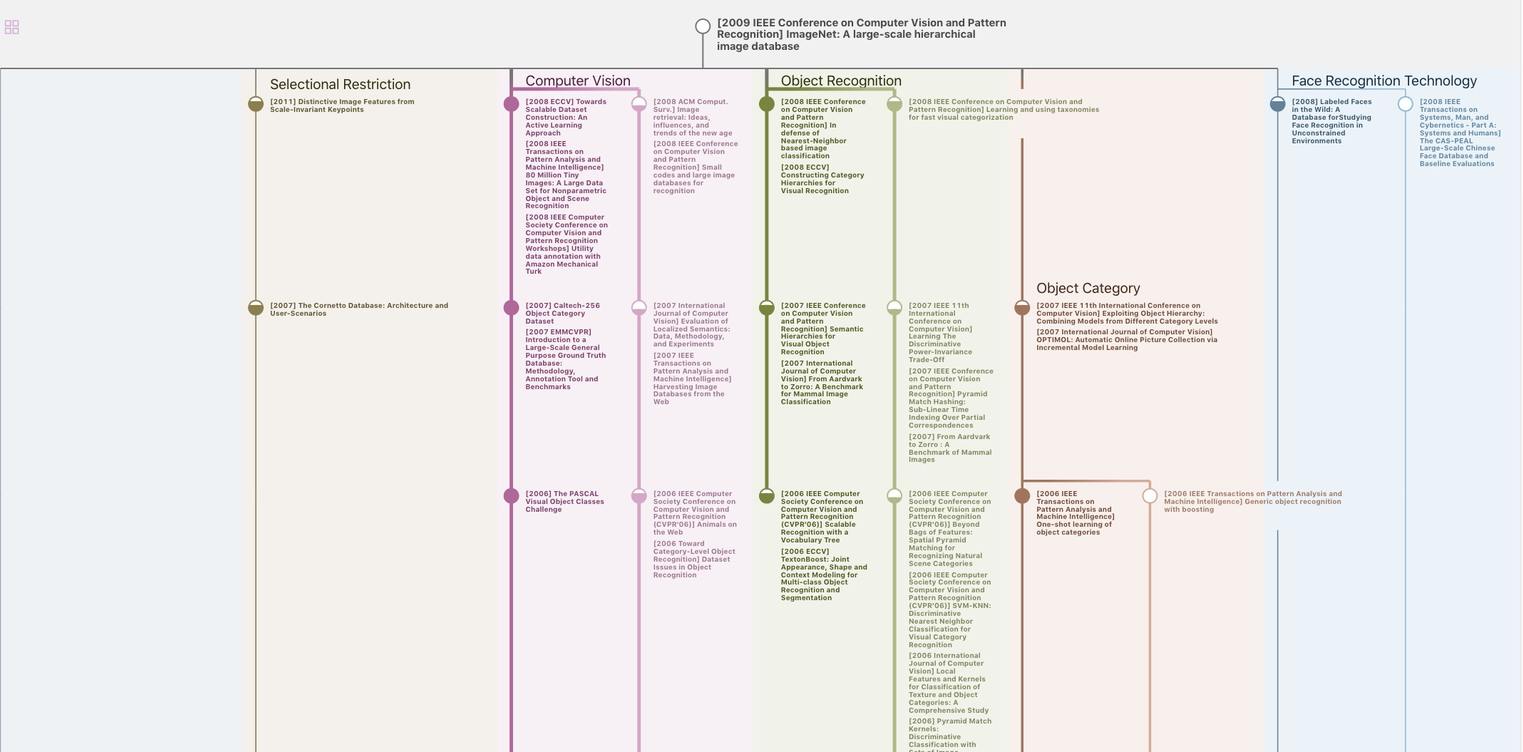
Generate MRT to find the research sequence of this paper
Chat Paper
Summary is being generated by the instructions you defined