Three-Phase Fault Arc Phase Selection Based on Global Attention Temporal Convolutional Neural Network
APPLIED SCIENCES-BASEL(2022)
Abstract
For low-voltage three-phase systems, the deep fault arc features are difficult to extract, and the phase information has strong timing. This phenomenon leads to the problem of low accuracy of fault phase selection. This paper proposes a three-phase fault arc phase selection method based on a global temporal convolutional network. First, this method builds a low-voltage three-phase arc fault data acquisition platform and establishes a dataset. Second, the experimental data were decomposed by variational mode decomposition and analyzed in the time-frequency domain. The decomposed data are reconstructed and used as input to the model. Finally, in order to reduce the fault features lost during the causal convolution operation, the global attention mechanism is used to extract deep fault characterization to identify faults and their differences. The experimental results show that the accuracy of the three-phase arc fault arc phase selection of the model can reach 98.62%, and the accuracy of single-phase fault detection can reach 99.39%. This model can effectively extract three-phase arc fault and phase characteristics. This paper provides a new idea for series fault arc detection and three-phase fault arc phase selection research.
MoreTranslated text
Key words
global attention mechanism, arc fault, deep learning, fault phase selection
AI Read Science
Must-Reading Tree
Example
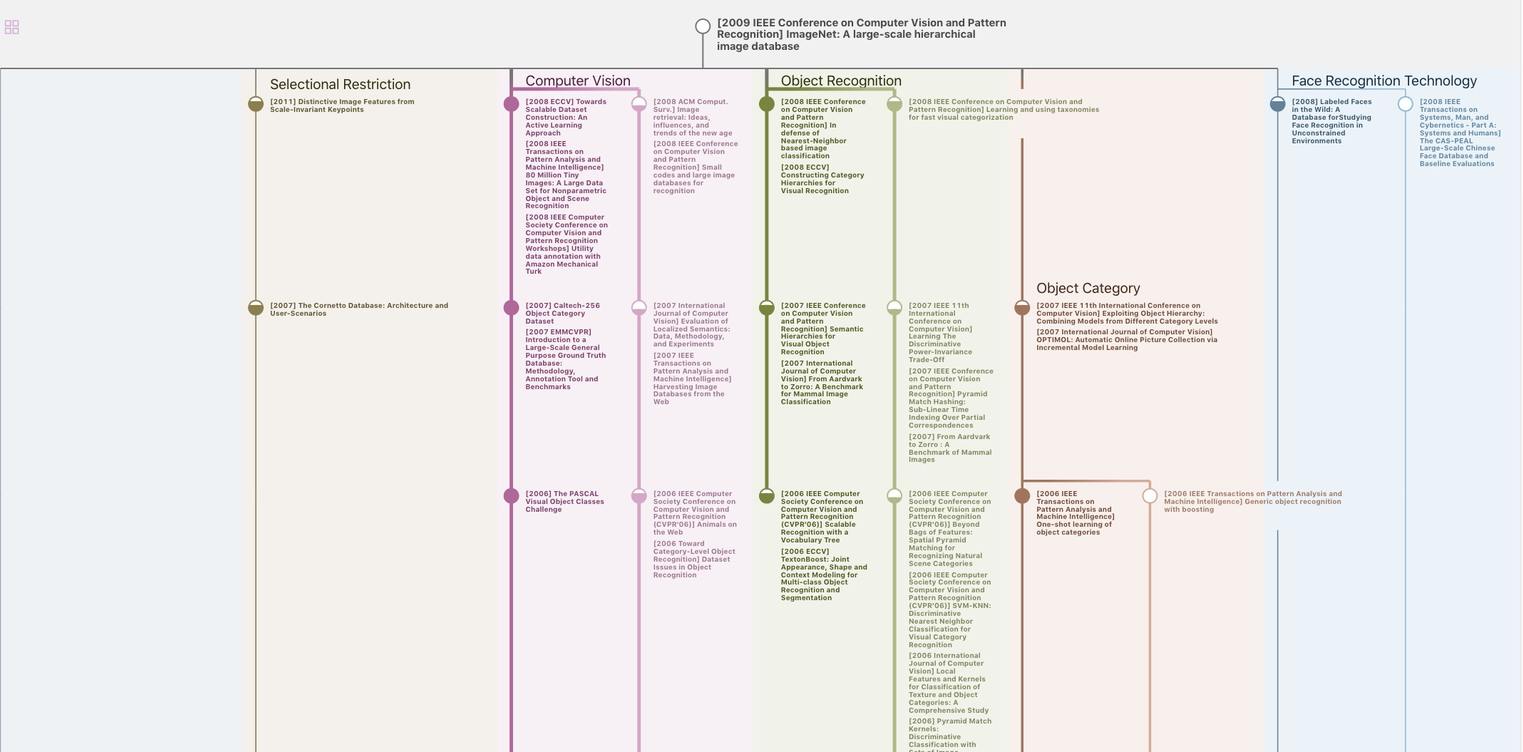
Generate MRT to find the research sequence of this paper
Chat Paper
Summary is being generated by the instructions you defined