Filling Missing Surface Roughness Data for Grinding Process Using Physics-Guided Neural Network
MANUFACTURING LETTERS(2022)
摘要
Missing data is often unavoidable in real-world datasets which may lead to poor data-driven analysis and unreliable prediction. Filling the missing data presents a nontrivial challenge in existing techniques. Current naive methods for filling the missing data often lead to biased approximations or make false assumptions about the data and correlations of the data. This paper presents a physics-guided neural network (PGNN) method that integrates the deep learning method with the guidance of a physics-based model to effectively fill the missing data that caused by limitations in information collection. The PGNN method first determines the coefficients of a physics-based model of a certain process by using machine learning (ML)-based estimations instead of experiment-based calibration. The outputs of this estimated physics-based model will serve as additional inputs for the next-level deep learning model. A numerical case study is performed to demonstrate the effectiveness of the proposed method by comparing it with other standard data-driven methods. The simulation results show that the PGNN method can more accurately fill the missing information and capture the uncertainty in the environment. (C) 2022 Society of Manufacturing Engineers (SME). Published by Elsevier Ltd. All rights reserved. Peer-review under responsibility of the Scientific Committee of the NAMRI/SME.
更多查看译文
关键词
Missing data filling, Smart manufacturing, Machine learning, Big data analytics, Integration of intelligent manufacturing processes with smart systems
AI 理解论文
溯源树
样例
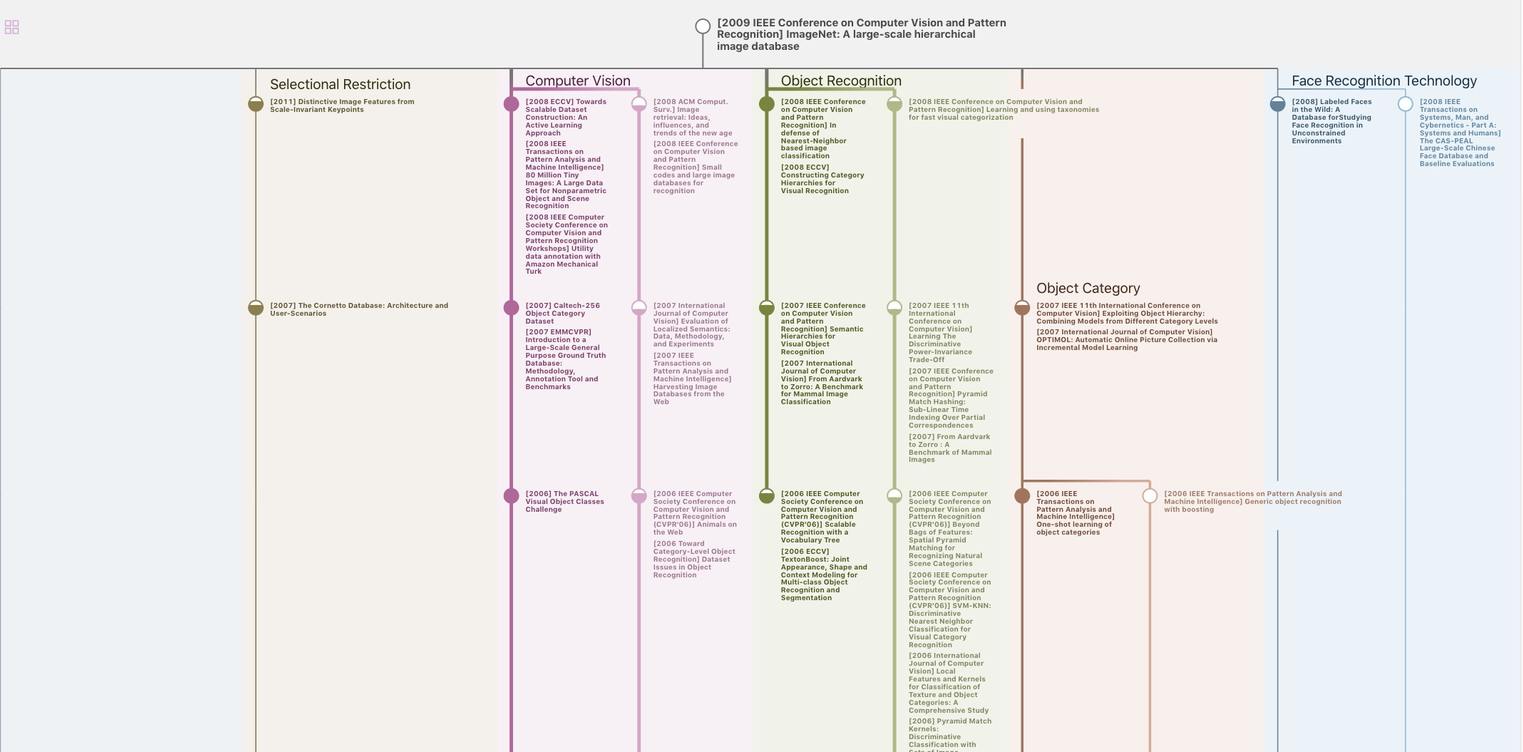
生成溯源树,研究论文发展脉络
Chat Paper
正在生成论文摘要