Joint Paraspinal Muscle Segmentation and Inter-rater Labeling Variability Prediction with Multi-task TransUNet
UNCERTAINTY FOR SAFE UTILIZATION OF MACHINE LEARNING IN MEDICAL IMAGING(2022)
摘要
Recent studies have associated morphological and composition changes in paraspinal muscles with low back pain (LBP), which is the most common, but poorly understood musculoskeletal disorder in adults. Accurate paraspinal muscle segmentation from MRI is crucial to enable new image-based biomarkers for the diagnosis and prognosis of LBP. Manual segmentation is laborious and time-consuming. In addition, high individual anatomical variations also pose challenges, resulting in inconsistent segmentation across different raters. While automatic segmentation algorithms can help mitigate the issues, techniques that predict and visualize inter-rater segmentation variability will be highly instrumental to help interpret reliability of automatic segmentation, but they are rarely attempted. In this paper, we propose a novel multi-task TransUNet model to accurately segment paraspinal muscles while predicting inter-rater labeling variability visualized using a variance map of three raters' annotations. Our technique is validated on MRIs of paraspinal muscles at four different disc levels from 118 LBP patients. Benefiting from the transformer mechanism and convolution neural networks, our algorithm is shown to perform better or similar to the state-of-the-art methods and a newly proposed multi-task U-Net model while predicting and visualizing multi-rater annotation variance per muscle group in an intuitive manner.
更多查看译文
关键词
Paraspinal muscle segmentation, TransUNet, Inter-rater variability, Uncertainty estimation, Multi-task learning, MRI
AI 理解论文
溯源树
样例
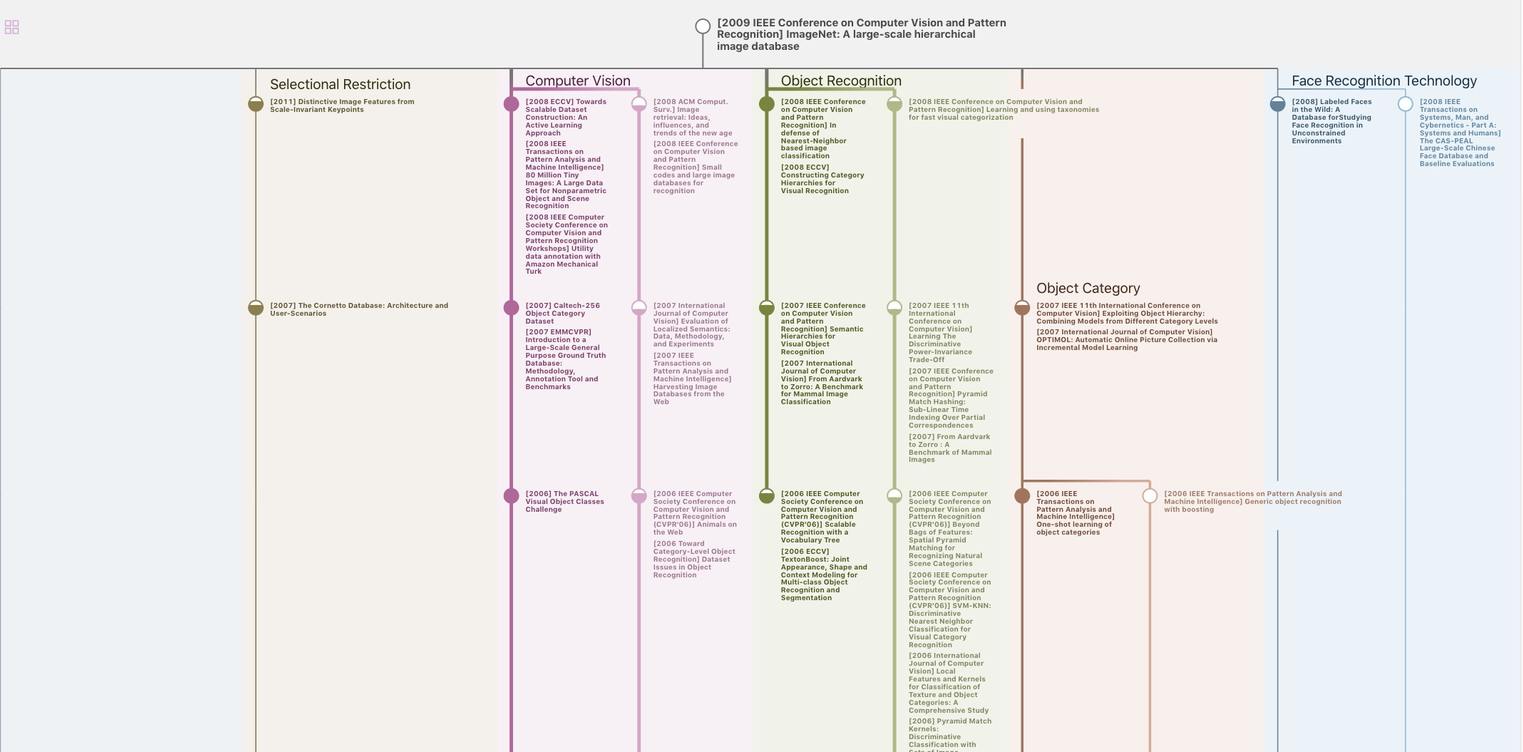
生成溯源树,研究论文发展脉络
Chat Paper
正在生成论文摘要