Sparse covariance matrix estimation for ultrahigh dimensional data
STAT(2022)
Abstract
We introduce a Covariance matrix Refitted Cross Validation (CovRCV) estimation procedure without requiring the Gaussian assumption. Specifically, we first use the modified Cholesky decomposition(MCD) to transform the problem of covariance matrix estimation to that of coefficient estimation in the regression setting. Then, we use an estimation method based on RCV to mitigate the prevalent spurious correlation in the ultrahigh dimensional regression. The proposed procedure is easy to implement. Furthermore, we establish the consistency of the proposed CovRCV estimation and show the rate of convergence without assuming the banded structure in Bickel and Levina (2008). The finite sample performance of our method is assessed via an intensive numerical study. Our numerical comparison shows that the CovRCV estimation outperforms the existing ones in various interesting scenarios. The advantages of our method are further illustrated by a real case study.
MoreTranslated text
Key words
covariance matrix,refitted cross validation,spurious correlation,ultrahigh dimensional data
AI Read Science
Must-Reading Tree
Example
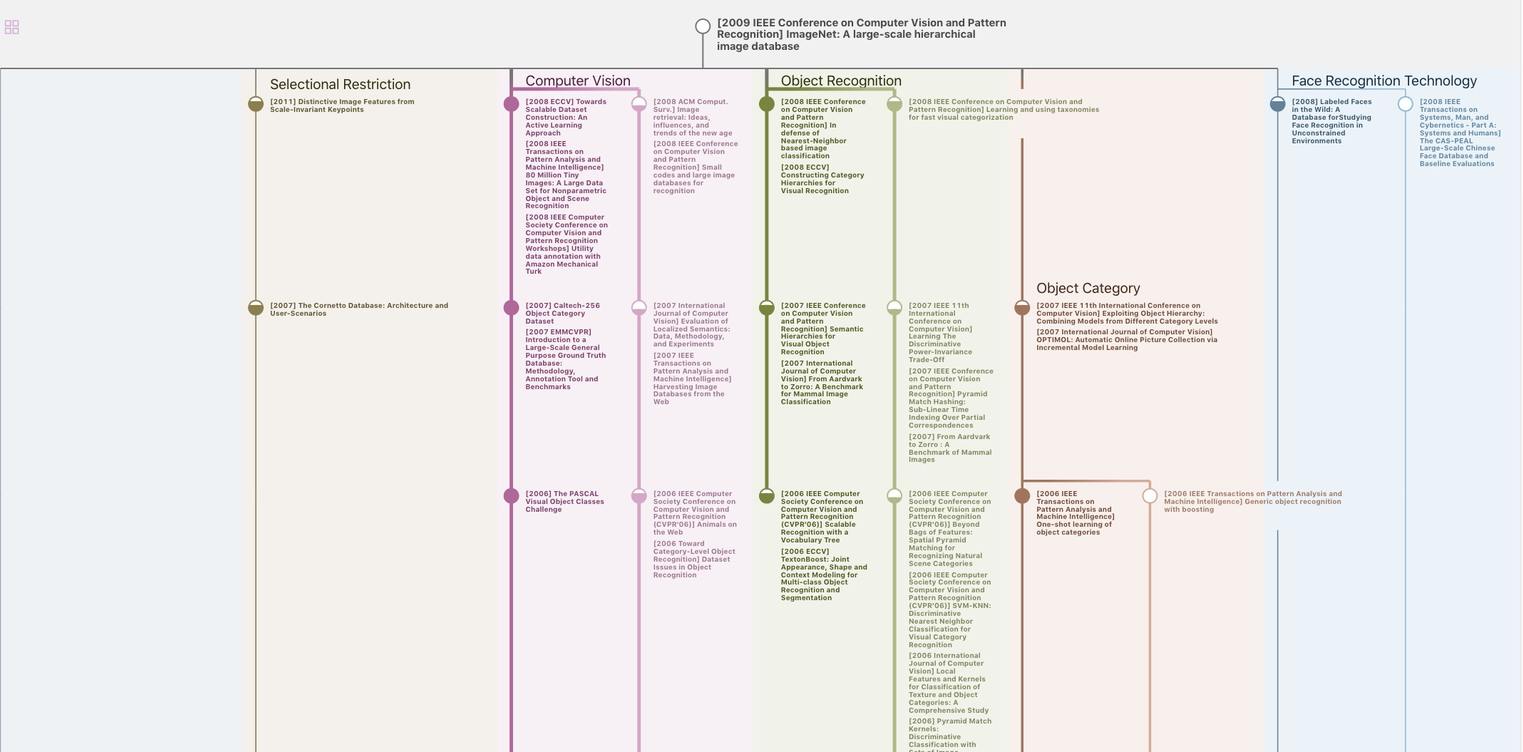
Generate MRT to find the research sequence of this paper
Chat Paper
Summary is being generated by the instructions you defined