A Generalization of Sigmoid Loss Function Using Tsallis Statistics for Binary Classification
NEURAL PROCESSING LETTERS(2022)
Abstract
In this paper, we present a generalization of sigmoid loss function by applying q -exponential ( q -exp) of Tsallis statistics. With this framework, we could relax and/or tighten-up the slopes of sigmoid loss which depend on the q values of q -exp. We called it q -sigmoid. Our derivation on q -sigmoid shows that the proposed loss function could give a way to explain learning rate factors, which in traditional gradient descent optimizations, are set heuristically and their values are selected empirically. Here, we relate it with Lipschitz constant and derive an adaptive way to determine q . We implement the proposed loss function on logistic regression for five datasets: MNIST, Cifar-10, Cifar-100, and two datasets for plant diseases detections. The experiments show that our method could outperform logistic regressions with sigmoid loss and fixed learning rate for some values of q .
MoreTranslated text
Key words
Surrogate loss functions, Sigmoid loss, q-exponential function, Tsallis statistics, Logistic regression
AI Read Science
Must-Reading Tree
Example
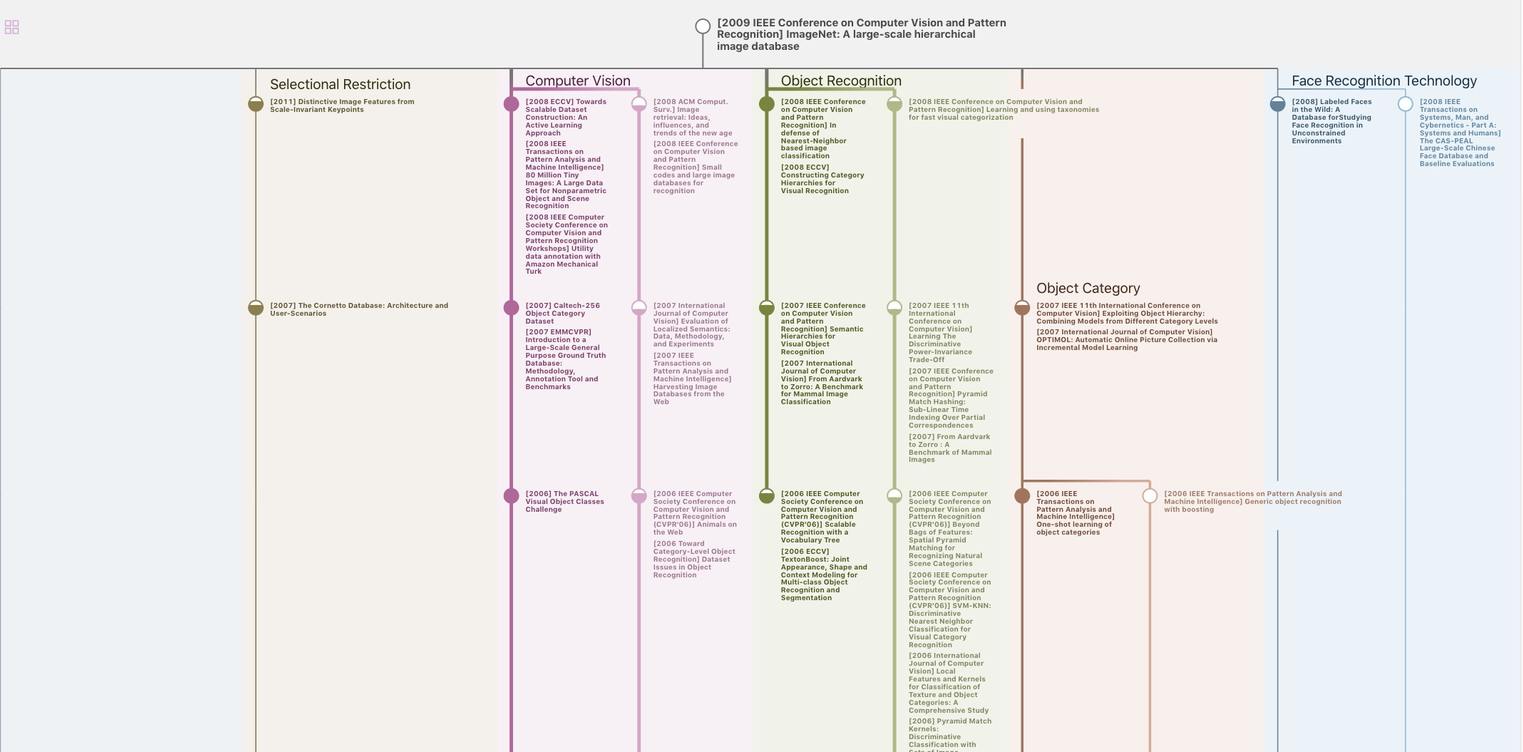
Generate MRT to find the research sequence of this paper
Chat Paper
Summary is being generated by the instructions you defined