Parameter and Hyperparameter Optimisation of Deep Neural Network Model for Personalised Predictions of Asthma
JOURNAL OF ADVANCES IN INFORMATION TECHNOLOGY(2022)
Abstract
Over the last couple of decades, numerous optimisation algorithms have been introduced to optimise machine learning models. However, until now, no evidence or framework can be found in the literature that adequately describes how to select the best algorithm for parameter and hyperparameter optimisation of the Deep Neural Network (DNN) model. In this paper, an enhanced Fragmented Grid Search (FGS) method has been introduced for tuning several hyperparameters and finding the optimal architecture of the DNN model using less computation power and time. Furthermore, several experimental models are trained on the asthma dataset using various optimisers to find the optimal parameters, which can help the DNN model converge towards the lowest loss value. The results show that the Adam optimiser provides the best accuracy rate (96%). Consequently, the optimised DNN model can be used for accurately providing personalised predictions of asthma exacerbations for effective asthma self-management.
MoreTranslated text
Key words
machine learning, deep neural networks, optimisation algorithm, personalization
AI Read Science
Must-Reading Tree
Example
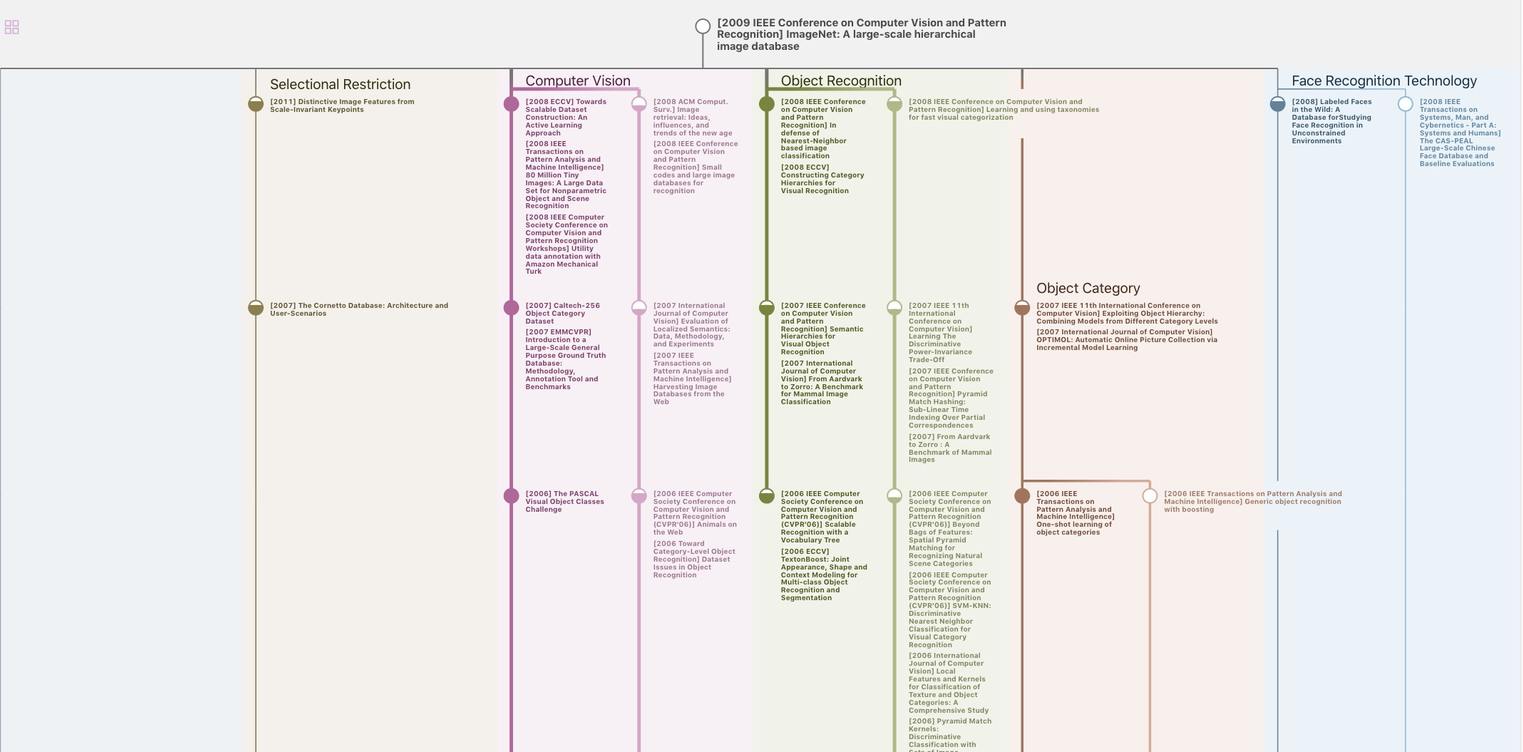
Generate MRT to find the research sequence of this paper
Chat Paper
Summary is being generated by the instructions you defined