A Machine Learning-Based Predictive Model to Identify Patients Who Failed to Attend a Follow-up Visit for Diabetes Care After Recommendations From a National Screening Program
DIABETES CARE(2022)
摘要
OBJECTIVE Reportedly, two-thirds of the patients who were positive for diabetes during screening failed to attend a follow-up visit for diabetes care in Japan. We aimed to develop a machine-learning model for predicting people's failure to attend a follow-up visit. RESEARCH DESIGN AND METHODS We conducted a retrospective cohort study of adults with newly screened diabetes at a national screening program using a large Japanese insurance claims database (JMDC, Tokyo, Japan). We defined failure to attend a follow-up visit for diabetes care as no physician consultation during the 6 months after the screening. The candidate predictors were patient demographics, comorbidities, and medication history. In the training set (randomly selected 80% of the sample), we developed two models (previously reported logistic regression model and Lasso regression model). In the test set (remaining 20%), prediction performance was examined. RESULTS We identified 10,645 patients, including 5,450 patients who failed to attend follow-up visits for diabetes care. The Lasso regression model using four predictors had a better discrimination ability than the previously reported logistic regression model using 13 predictors (C-statistic: 0.71 [95% CI 0.69-0.73] vs. 0.67 [0.65-0.69]; P<0.001). The four selected predictors in the Lasso regression model were lower frequency of physician visits in the previous year, lower HbA(1c) levels, and negative history of antidyslipidemic or antihypertensive treatment. CONCLUSIONS The developed machine-learning model using four predictors had a good predictive ability to identify patients who failed to attend a follow-up visit for diabetes care after a screening program.
更多查看译文
AI 理解论文
溯源树
样例
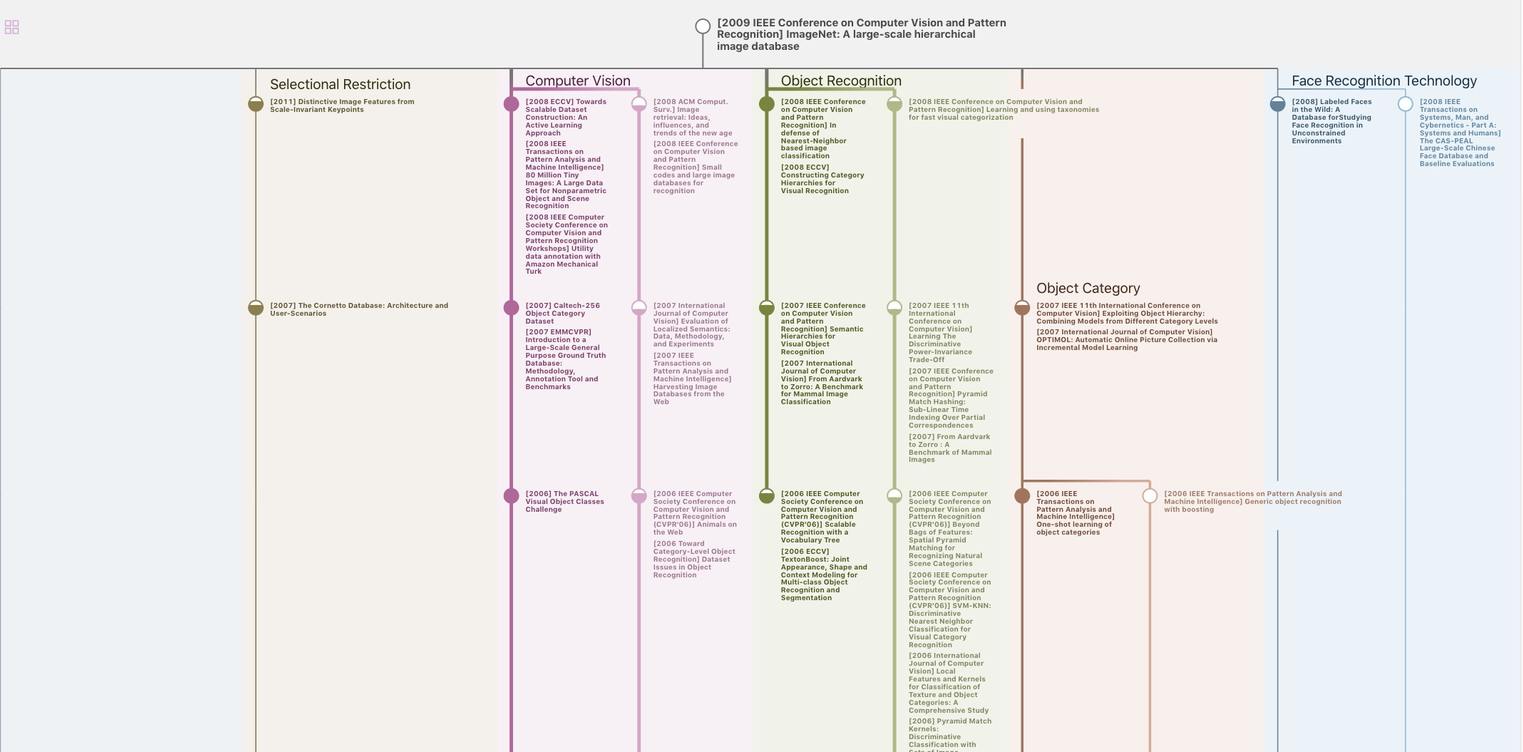
生成溯源树,研究论文发展脉络
Chat Paper
正在生成论文摘要