Machine learning-based inverse design for single-phase high entropy alloys
APL MATERIALS(2022)
摘要
In this work, we develop an inverse design framework to search for single-phase high entropy alloys (HEAs) subjected to specified phase targets and constraints. This framework is based on the fast grid search in the composition-temperature space, enabled by a highly accurate and efficient machine learning model trained by a huge amount of data. Using the framework, we search through the entire quaternary, quinary, and senary alloy systems, formed by Al, Co, Cr, Cu, Fe, Mn, Ni, and Ti, to identify three types of HEAs: (1) the single-phase FCC HEA with the highest Al content; (2) the single-phase FCC HEA with lower equilibrium temperatures; and (3) single-phase BCC HEAs with Al as the principal element. For the first time, we reveal that the highest Al content in single-phase FCC HEAs is 0.15 in mole fraction, which is higher than the Al contents in all reported single-phase FCC HEAs. The identified HEAs for the quaternary, quinary, and senary groups are Al0.15Co0.34Cr0.16Ni0.35, Al0.15Co0.35Cr0.1Fe0.05Ni0.35, and Al0.15Co0.36Cr0.06Fe0.06Mn0.01Ni0.36, respectively. All the designed HEAs are verified by the equilibrium calculations with Thermo-Calc software and the TCHEA3 database. We further conduct Scheil-Gulliver calculations and experimental fabrications and characterizations for the designed HEAs, to verify the formation of the targeted phases at non-equilibrium conditions. This work demonstrates a viable approach to design HEAs with specified phase targets and constraints. (C) 2022 Author(s).
更多查看译文
关键词
alloys,entropy,inverse design,learning-based,single-phase
AI 理解论文
溯源树
样例
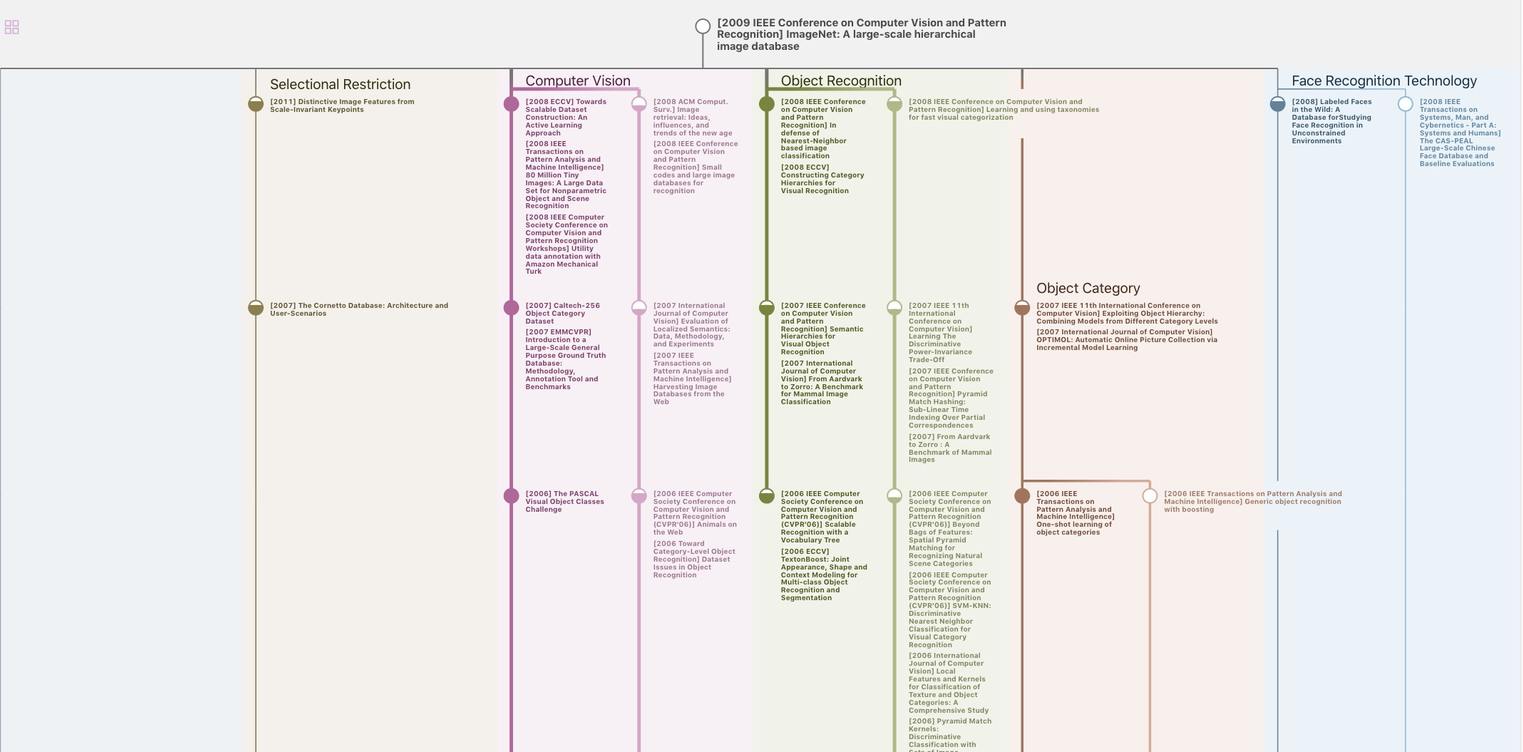
生成溯源树,研究论文发展脉络
Chat Paper
正在生成论文摘要