Mining Diversified Top-r Lasting Cohesive Subgraphs on Temporal Networks
IEEE TRANSACTIONS ON BIG DATA(2022)
摘要
Temporal subgraph mining is recently ubiquitous. Identifying diversified and lasting ingredients is a fundamental problem in analyzing temporal networks. In this paper, we investigate the problem of finding diversified lasting cohesive subgraphs from temporal networks. Specifically, we first introduce a new model, called maximal lasting (k, sigma)-core, for characterizing lasting cohesive subgraphs on temporal networks so as to the nodes in the subgraph are connected densely and also the subgraph's structure remains unchanged for a period of time. To enhance the diversity of results, we then formulate a diversified lasting cohesive subgraphs problem, which finds r maximal lasting (k, sigma)-cores with maximum coverage regarding the number of vertices and timestamps. Unfortunately, we show that the optimization problem is NP-hard. To tackle this issue, we first devise a greedy algorithm named GreLC with (1-1/e) approximation ratio. However, GreLC has prohibitively high time and space complexity, resulting in poor scalability. Then, an improved DFS-based search algorithm called TopLC with 1/4 approximation ratio is proposed to lower the computational cost. Finally, empirical studies on six real-world temporal networks demonstrate that the proposed solutions perform efficiently and accurately, and our model is better than temporal cohesive subgraphs detected by existing approaches.
更多查看译文
关键词
Cohesive subgraphs,temporal networks,lasting pattern mining,diversified top-r search
AI 理解论文
溯源树
样例
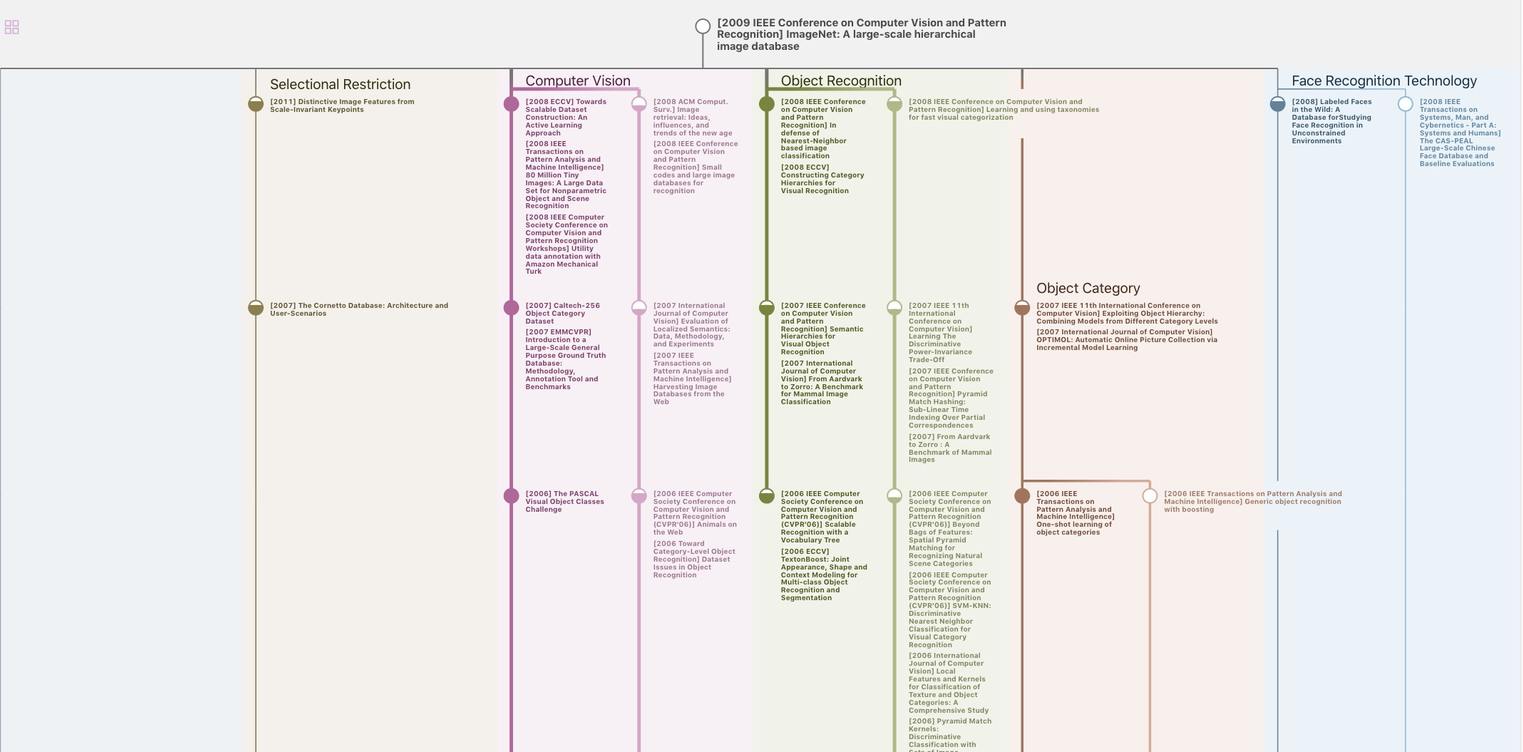
生成溯源树,研究论文发展脉络
Chat Paper
正在生成论文摘要