A Method of Named Entity Recognition for Tigrinya
APPLIED COMPUTING REVIEW(2022)
摘要
This paper proposes a method for Named-Entity Recognition (NER) for a low-resource language, Tigrinya, using a pre-trained language model. Tigrinya is a morphologically rich, although one of the underrepresented in the field of NLP. This is mainly due to the limited amount of annotated data available. To address this problem, we present the first publicly available datasets of NER for Tigrinya containing two versions, namely, (V1 and V2) annotated manually. The V1 and V2 datasets contain 69,309 and 40,627 tokens, respectively, where the annotations are based on the CoNLL 2003 Beginning, Inside, and Outside (BIO) tagging schema. Specifically, we develop a new pre-trained language model for Tigrinya based on RoBERTa, which we refer to as TigRoBERTa. Our model is then fine-tuned on downstream tasks on a more specific target NER and POS tasks with limited data. Finally, we further enhance the model performance by applying semi-supervised self-training using unlabeled data. The experimental results show that the method achieved 84% F1-score for NER and 92% accuracy for POS tagging, which is better than or comparable to the baseline method based on the CNN-BiLSTM-CRF.
更多查看译文
关键词
Named entity recognition,POS tagging,pre-trained language model,low-resource language,semi-supervised learning
AI 理解论文
溯源树
样例
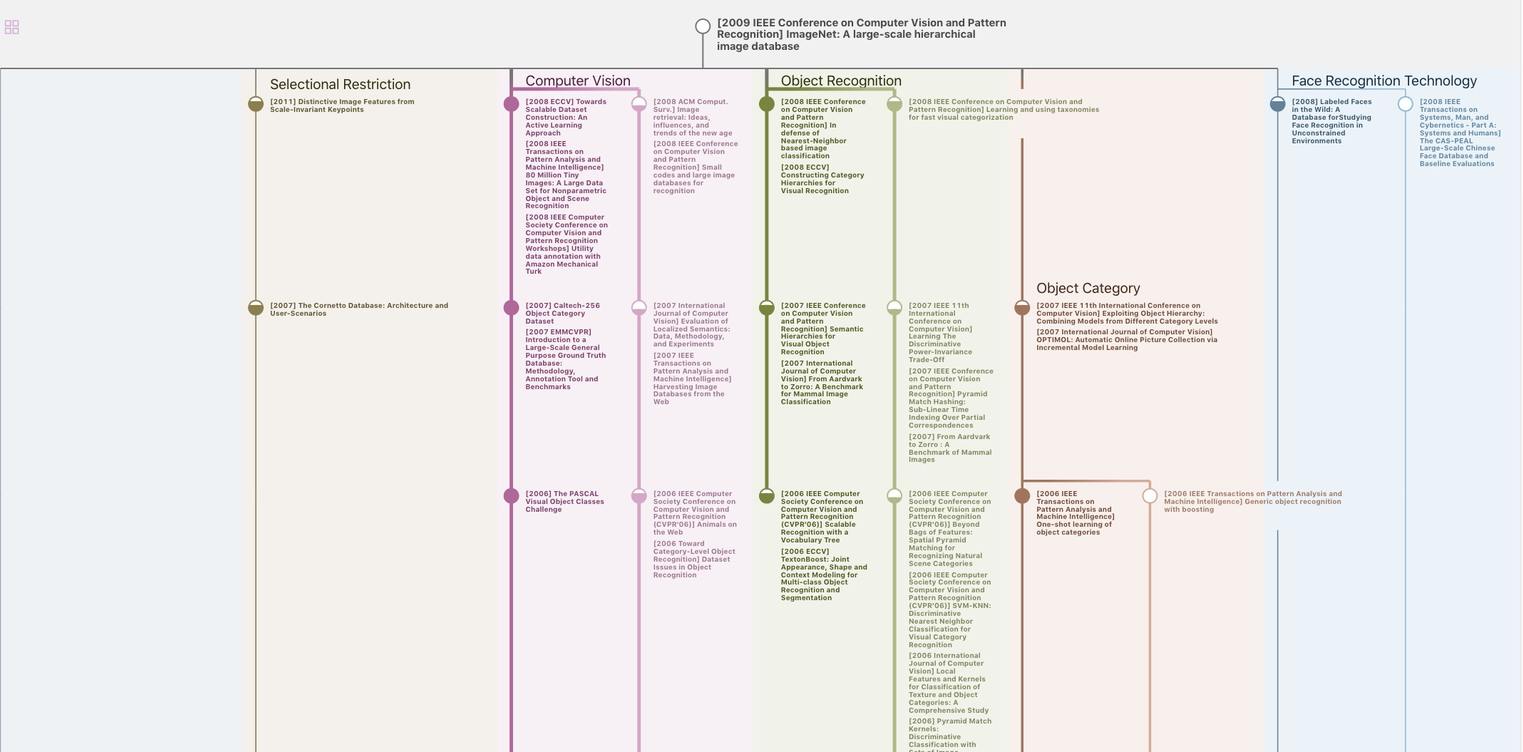
生成溯源树,研究论文发展脉络
Chat Paper
正在生成论文摘要