Crack detection based on deep learning: a method for evaluating the object detection networks considering the random fractal of crack
STRUCTURAL HEALTH MONITORING-AN INTERNATIONAL JOURNAL(2023)
摘要
The visual test for cracks is a tough work for human eyes. With the help of cameras, methods for object detection based on deep learning can detect the cracks automatically from digital images. However, an anomaly is observed that the models for crack detection are obviously underestimated by the widely used mean Average Precision (mAP) standard. In this study, by theoretical analyses about the topology of cracks, we attribute the failure of mAP standard to the random fractal feature. More deeply, this issue is due to the strict box matching during the calculation. To solve this problem, we construct a practical fractal-available evaluation method adopting the idea of covering box matching. Several metrics including Extended Recall (XR), Extended Precision (XP), and Extended F-score (F-ext) are defined for scoring the crack detectors. Then, we conduct experiments on several mainstream models for object detection. Results show two advantages of the proposed evaluation method: (1) the proposed metrics evaluate cases better; (2) the proposed evaluation is non-maximum suppression-unrelated. Moreover, we present a case study to optimize a faster region-based convolutional neural networks model for crack detection adopting the proposed metrics. Recall (represented by the XR) of our best model achieves an industrial-level at 95.8%. These results denote that the proposed evaluation method removes the obstacle in evaluating the methods for object detection for cracks, which show great potential for automatic industrial inspections.
更多查看译文
关键词
Crack detection,convolutional neural networks,object detection based on deep learning,automatic visual test,random fractal
AI 理解论文
溯源树
样例
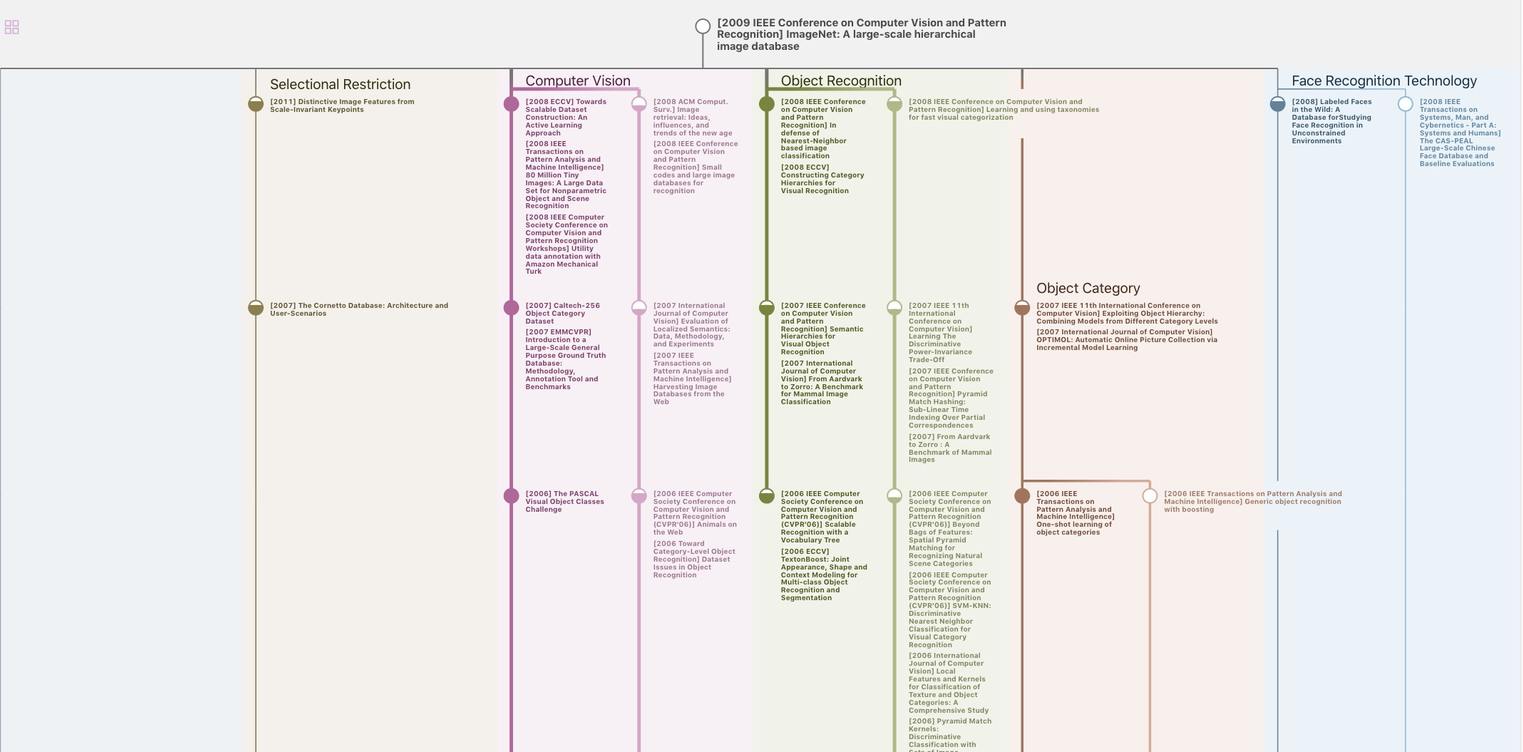
生成溯源树,研究论文发展脉络
Chat Paper
正在生成论文摘要