Group event recommendation based on a heterogeneous attribute graph considering long- and short- term preferences
JOURNAL OF INTELLIGENT INFORMATION SYSTEMS(2022)
摘要
Recently, a new type of social network, the event-based social network (EBSN), has become popular. Typical EBSN platforms include Meetup, Plancast, Doublan, etc. In an EBSN platform, users usually participate in events not as a single person, but as a member of a group. An issue with these networks is that the amount of events posted in them makes it difficult for users to find the events they are interested in. One effective way to solve this problem for the EBSN is to utilize a group event recommendation system. However, though existing group event recommendation methods have achieved good performance, they still suffer from two challenges: 1) many existing methods only use the explicit attribute information in EBSN and fail to exploit implicit social information and high-order structure information, resulting in the problem of data sparsity and 2) most of the existing methods treat user preferences as static rather than considering their dynamic variability of user preferences. In this paper, we propose to build a novel group event recommendation model based on a Heterogeneous Attribute Graph (HAG) considering users' long-term and short-term preferences. Specifically, considering that the group event scoring matrix in EBSN is a sparse matrix, we make full use of a variety of information in EBSN, including explicit attribute information, implicit social information and high-order structure information in the social network. We then make use of graph attention networks (GAN), which encode that information by propagating embedding over the graph, to learn the representations of users and events. We conduct in-depth learning on the recent interactions between users and events, and the obtained implicit vector is represented as the user's short-term preference. In the same way, we also conduct in-depth learning on the complete history of interactions between users and events, except for recent interactions, and regard the learned implicit vector as the user's long-term preference. Finally, we fuse the users' long- and short-term preference representations using a multi-layer perceptron and attention mechanism to generate group preference representation. Experimental results on real-world datasets demonstrate that our proposed HAG model provides performance improvement over the baseline methods on group event recommendation.
更多查看译文
关键词
Group event recommendation,EBSN,Heterogeneous attribute graph,Attention
AI 理解论文
溯源树
样例
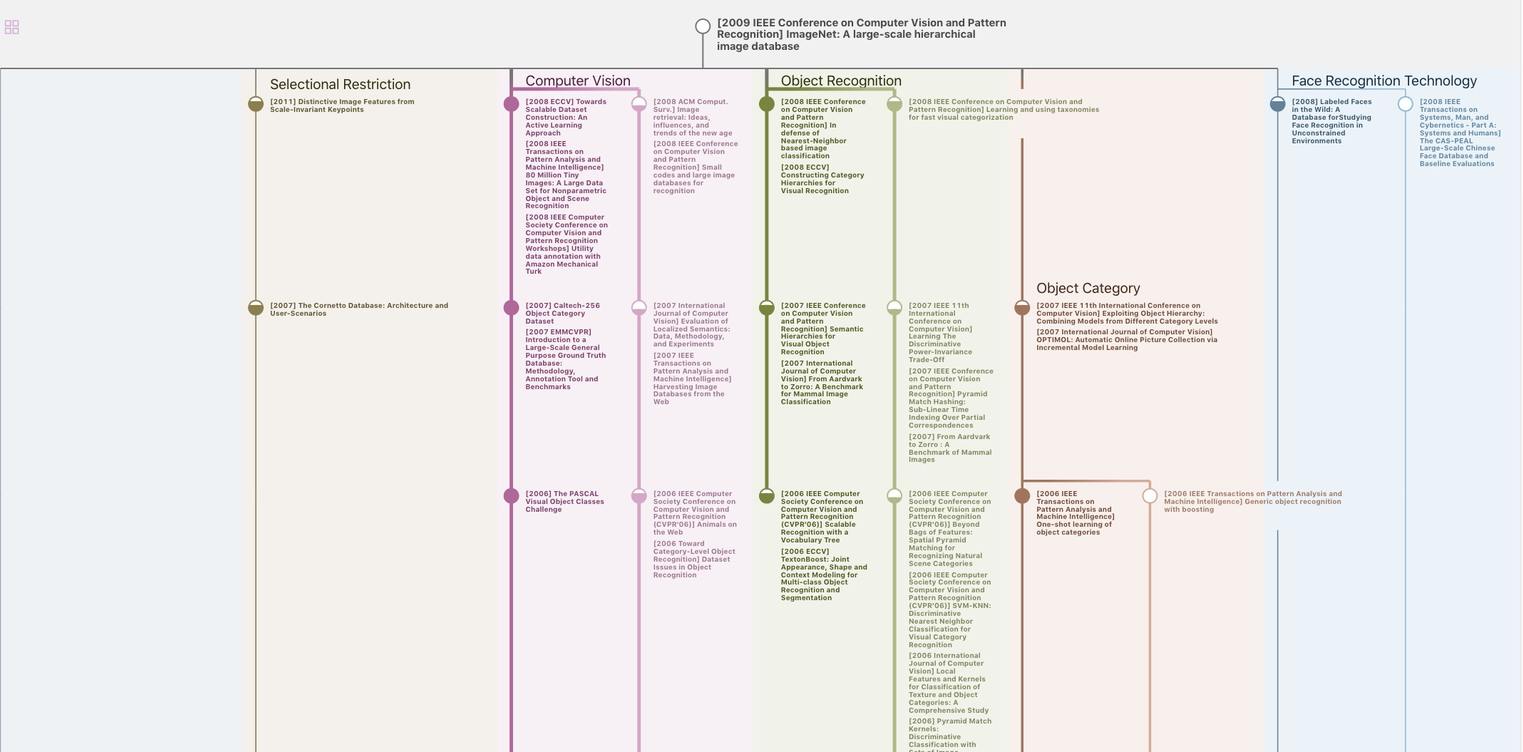
生成溯源树,研究论文发展脉络
Chat Paper
正在生成论文摘要