A hybrid forecasting model based on the group method of data handling and wavelet decomposition for monthly rivers streamflow data sets
OPEN PHYSICS(2022)
摘要
The natural streamflow of the River is encouraged to forecast through multiple methods. The impartiality of this study is the comparison of the forecast accuracy rates of the time-series (TS) hybrid model with the conventional model. The behavior of the natural monthly statistical chaotic streamflow to use in the forecasting models has been compiled by projecting two distinguished rivers, the Indus and Chenab of Pakistan. Therefore, this article is based on the monthly streamflow forecast analysis that has been reported using the group method of data handling with wavelet decomposition (WGMDH) as a new forecasting attribute. Discrete wavelets decompose the perceived data into sub-series and forecast hydrological variables; these fittingly have been endorsed as inputs in the hybrid model. The forecast efficiency and estimations of the hybrid model are measured by the appropriate statistical techniques such as mean absolute error (RME), root mean square error (RMSE), and correlation coefficients (R) and compared to the group method of data handling (GMDH), least-square support vector machine and artificial neural network conventional models. The comparative analysis shows that the hybrid WGMDH model is more stable and more potent for forecasting river flow than other predictive models and significantly proved that the hybrid model is a robust alternate forecasting tool for TS data sets.
更多查看译文
关键词
monthly data sets, hybrid, group method and data handling, wavelet decomposition, statistical techniques
AI 理解论文
溯源树
样例
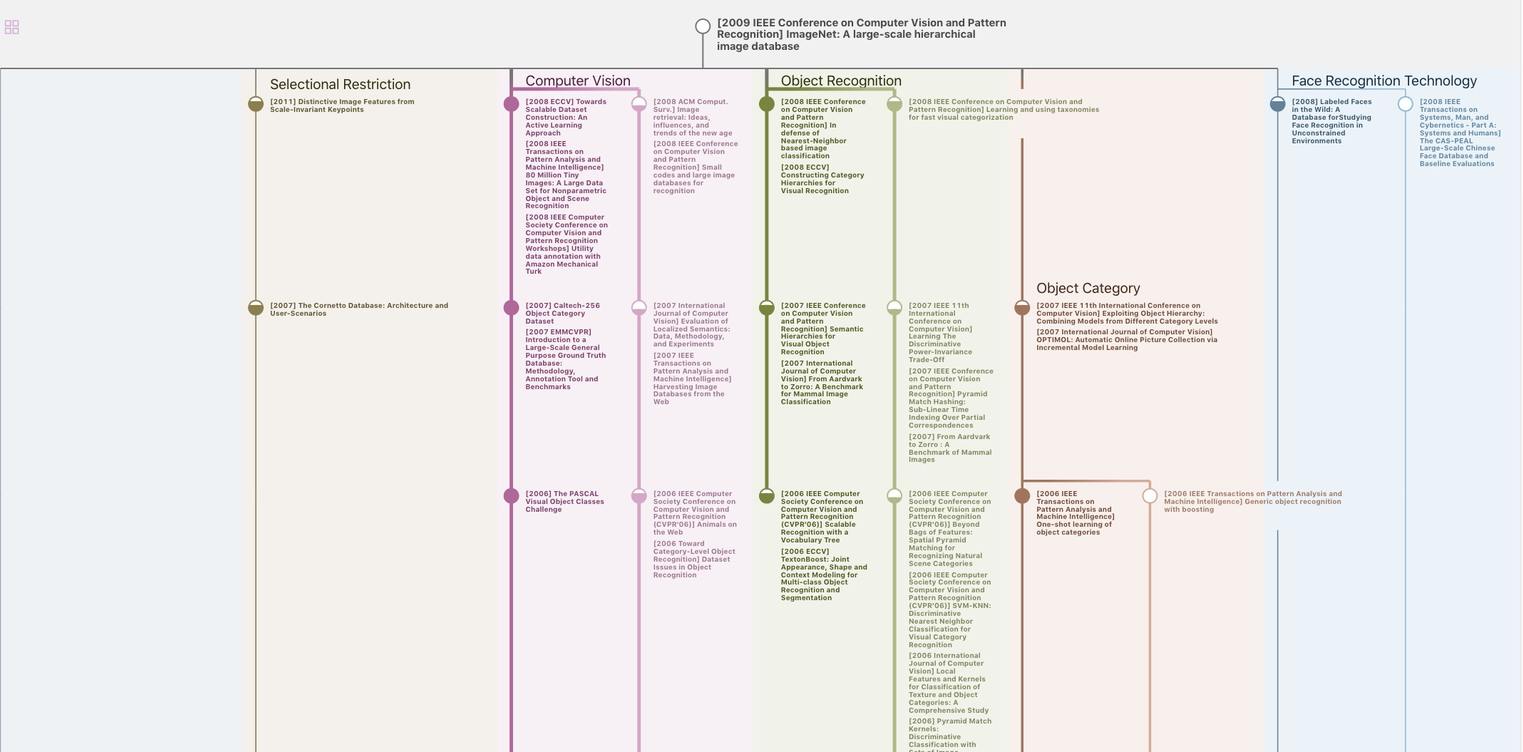
生成溯源树,研究论文发展脉络
Chat Paper
正在生成论文摘要