Machine learning for the real-time assessment of left ventricular ejection fraction in critically ill patients: a bedside evaluation by novices and experts in echocardiography
Critical care (London, England)(2022)
摘要
Background Machine learning algorithms have recently been developed to enable the automatic and real-time echocardiographic assessment of left ventricular ejection fraction (LVEF) and have not been evaluated in critically ill patients. Methods Real-time LVEF was prospectively measured in 95 ICU patients with a machine learning algorithm installed on a cart-based ultrasound system. Real-time measurements taken by novices (LVEF Nov ) and by experts (LVEF Exp ) were compared with LVEF reference measurements (LVEF Ref ) taken manually by echo experts. Results LVEF Ref ranged from 26 to 80% (mean 54 ± 12%), and the reproducibility of measurements was 9 ± 6%. Thirty patients (32%) had a LVEF Ref < 50% (left ventricular systolic dysfunction). Real-time LVEF Exp and LVEF Nov measurements ranged from 31 to 68% (mean 54 ± 10%) and from 28 to 70% (mean 54 ± 9%), respectively. The reproducibility of measurements was comparable for LVEF Exp (5 ± 4%) and for LVEF Nov (6 ± 5%) and significantly better than for reference measurements ( p < 0.001). We observed a strong relationship between LVEF Ref and both real-time LVEF Exp ( r = 0.86, p < 0.001) and LVEF Nov ( r = 0.81, p < 0.001). The average difference (bias) between real time and reference measurements was 0 ± 6% for LVEF Exp and 0 ± 7% for LVEF Nov . The sensitivity to detect systolic dysfunction was 70% for real-time LVEF Exp and 73% for LVEF Nov . The specificity to detect systolic dysfunction was 98% both for LVEF Exp and LVEF Nov . Conclusion Machine learning-enabled real-time measurements of LVEF were strongly correlated with manual measurements obtained by experts. The accuracy of real-time LVEF measurements was excellent, and the precision was fair. The reproducibility of LVEF measurements was better with the machine learning system. The specificity to detect left ventricular dysfunction was excellent both for experts and for novices, whereas the sensitivity could be improved. Trial registration : NCT05336448. Retrospectively registered on April 19, 2022.
更多查看译文
关键词
Artificial intelligence,Echocardiography,Left ventricular ejection fraction,Machine learning,Novice,Point of care ultrasound
AI 理解论文
溯源树
样例
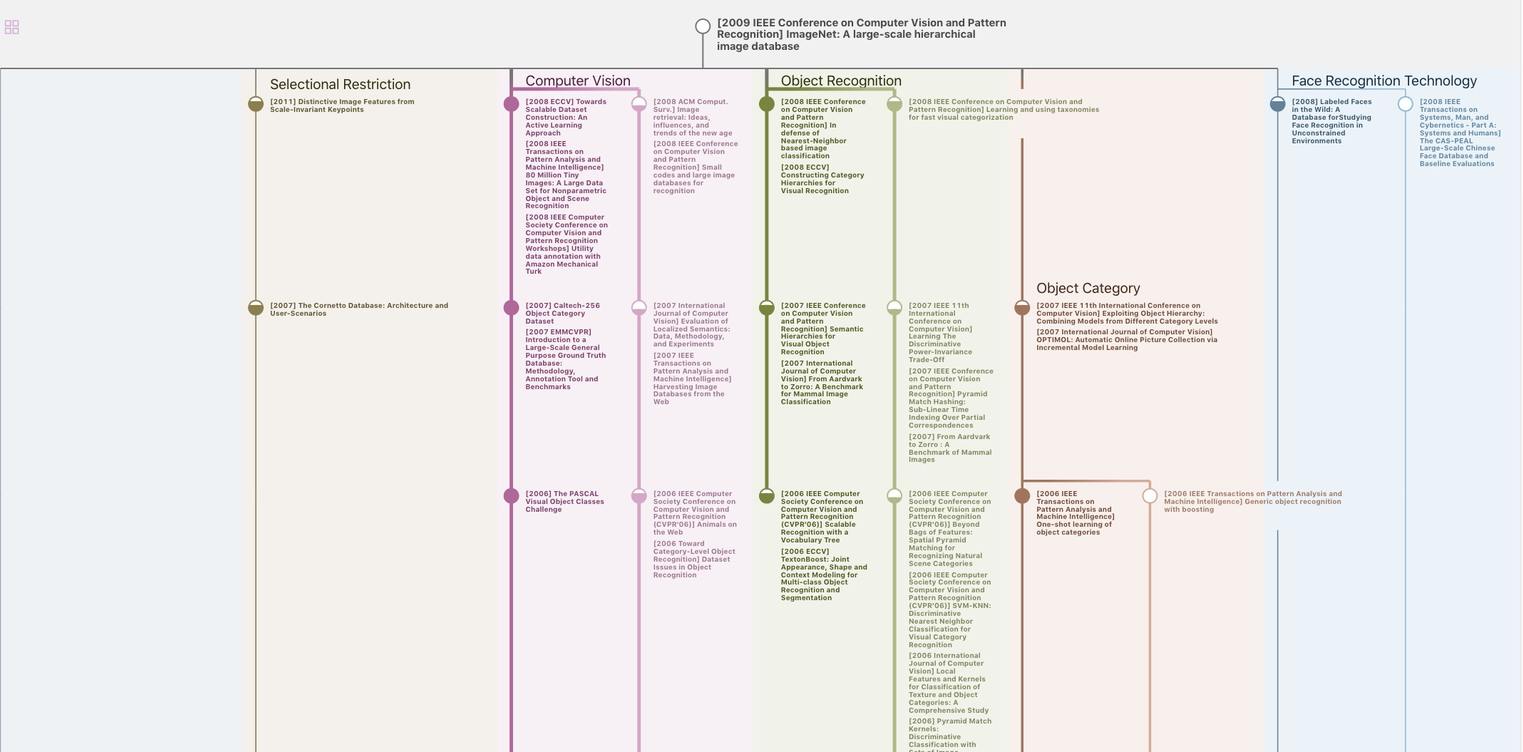
生成溯源树,研究论文发展脉络
Chat Paper
正在生成论文摘要