On Explicit Curvature Regularization in Deep Generative Models
CoRR(2023)
Abstract
We propose a family of curvature-based regularization terms for deep generative model learning. Explicit coordinate-invariant formulas for both intrinsic and extrinsic curvature measures are derived for the case of arbitrary data manifolds embedded in higher-dimensional Euclidean space. Because computing the curvature is a highly computation-intensive process involving the evaluation of second-order derivatives, efficient formulas are derived for approximately evaluating intrinsic and extrinsic curvatures. Comparative studies are conducted that compare the relative efficacy of intrinsic versus extrinsic curvature-based regularization measures, as well as performance comparisons against existing autoencoder training methods. Experiments involving noisy motion capture data confirm that curvature-based methods outperform existing autoencoder regularization methods, with intrinsic curvature measures slightly more effective than extrinsic curvature measures.
MoreTranslated text
Key words
explicit curvature regularization,models
AI Read Science
Must-Reading Tree
Example
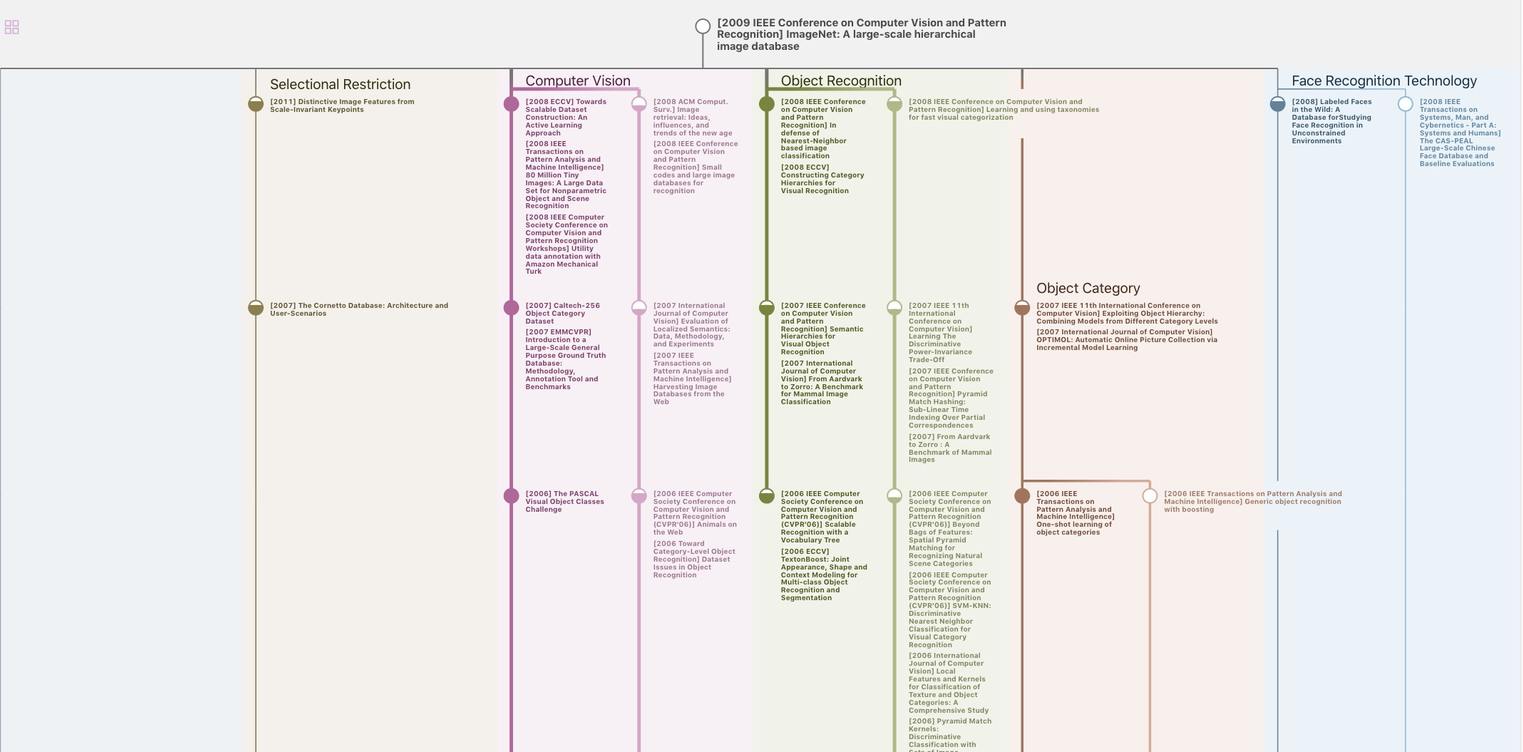
Generate MRT to find the research sequence of this paper
Chat Paper
Summary is being generated by the instructions you defined