Consistency tests of field level inference with the EFT likelihood
arxiv(2023)
摘要
Analyzing the clustering of galaxies at the field level in principle promises access to all the cosmological information available. Given this incentive, in this paper we investigate the performance of field-based forward modeling approach to galaxy clustering using the effective field theory (EFT) framework of large-scale structure (LSS). We do so by applying this formalism to a set of consistency and convergence tests on synthetic datasets. We explore the high-dimensional joint posterior of LSS initial conditions by combining Hamiltonian Monte Carlo sampling for the field of initial conditions, and slice sampling for cosmology and model parameters. We adopt the Lagrangian perturbation theory forward model from [1], up to second order, for the forward model of biased tracers. We specifically include model mis-specifications in our synthetic datasets within the EFT framework. We achieve this by generating synthetic data at a higher cutoff scale Lambda(0), which controls which Fourier modes enter the EFT likelihood evaluation, than the cutoff Lambda used in the inference. In the presence of model mis-specifications, we find that the EFT framework still allows for robust, unbiased joint inference of a) cosmological parameters - specifically, the scaling amplitude of the initial conditions - b) the initial conditions themselves, and c) the bias and noise parameters. In addition, we show that in the purely linear case, where the posterior is analytically tractable, our samplers fully explore the posterior surface. We also demonstrate convergence in the cases of nonlinear forward models. Our findings serve as a confirmation of the EFT field-based forward model framework developed in [2-7], and as another step towards field-level cosmological analyses of real galaxy surveys.
更多查看译文
关键词
cosmological parameters from LSS, galaxy clustering, cosmological simulations
AI 理解论文
溯源树
样例
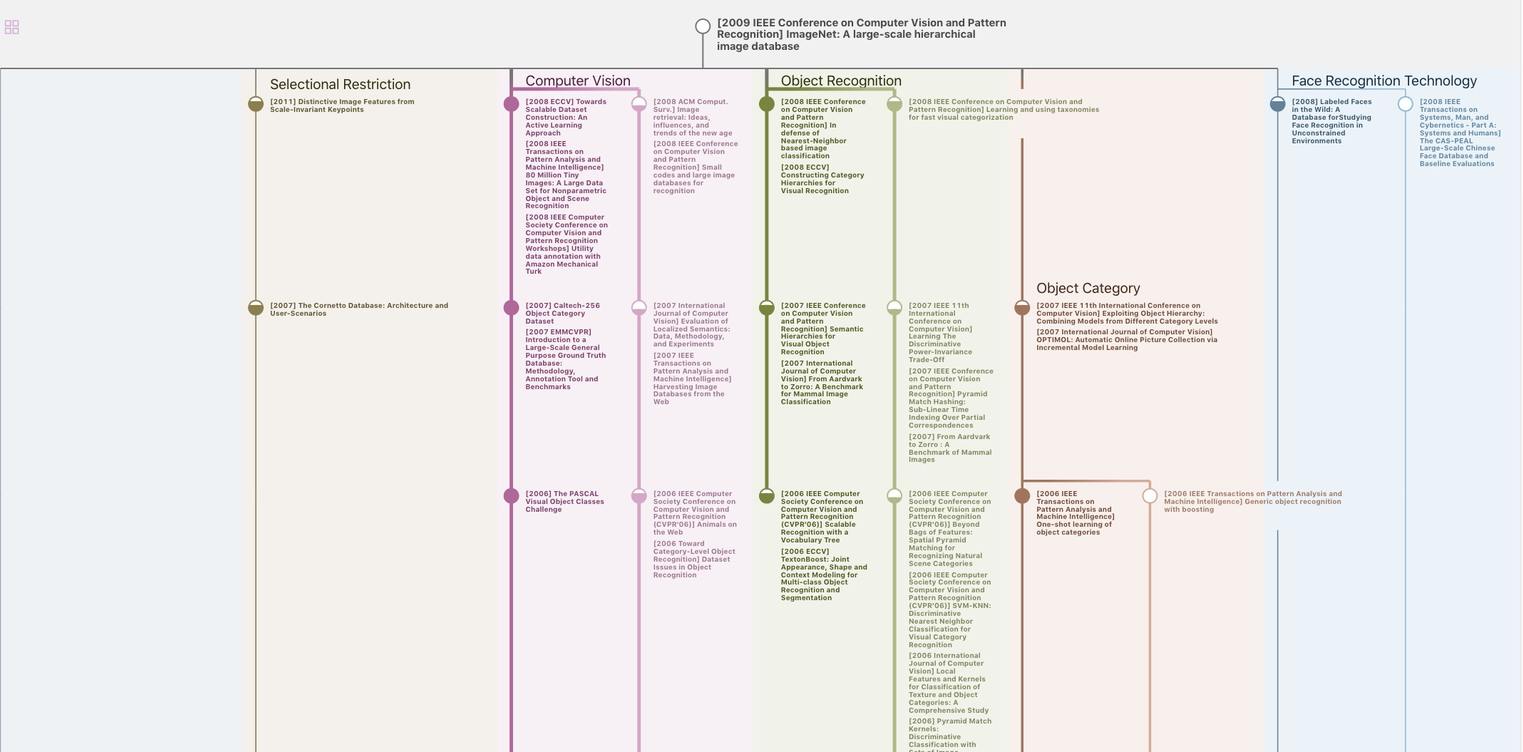
生成溯源树,研究论文发展脉络
Chat Paper
正在生成论文摘要