Solve the Puzzle of Instance Segmentation in Videos: A Weakly Supervised Framework With Spatio-Temporal Collaboration
IEEE Transactions on Circuits and Systems for Video Technology(2023)
摘要
Instance segmentation in videos, which aims to segment and track multiple objects in video frames, has garnered a flurry of research attention in recent years. In this paper, we present a novel weakly supervised framework with Spatio-Temporal Collaboration for instance Segmentation in videos, namely STC-Seg. Concretely, STC-Seg demonstrates four contributions. First, we leverage the complementary representations from unsupervised depth estimation and optical flow to produce effective pseudo-labels for training deep networks and predicting high-quality instance masks. Second, to enhance the mask generation, we devise a puzzle loss, which enables end-to-end training using box-level annotations. Third, our tracking module jointly utilizes bounding-box diagonal points with spatio-temporal discrepancy to model movements, which largely improves the robustness to different object appearances. Finally, our framework is flexible and enables image-level instance segmentation methods to operate the video-level task. We conduct an extensive set of experiments on the KITTI MOTS and YT-VIS datasets. Experimental results demonstrate that our method achieves strong performance and even outperforms fully supervised TrackR-CNN and MaskTrack R-CNN. We believe that STC-Seg can be a valuable addition to the community, as it reflects the tip of an iceberg about the innovative opportunities in the weakly supervised paradigm for instance segmentation in videos.
更多查看译文
关键词
Video instance segmentation,weakly supervised learning,multi-object tracking and segmentation
AI 理解论文
溯源树
样例
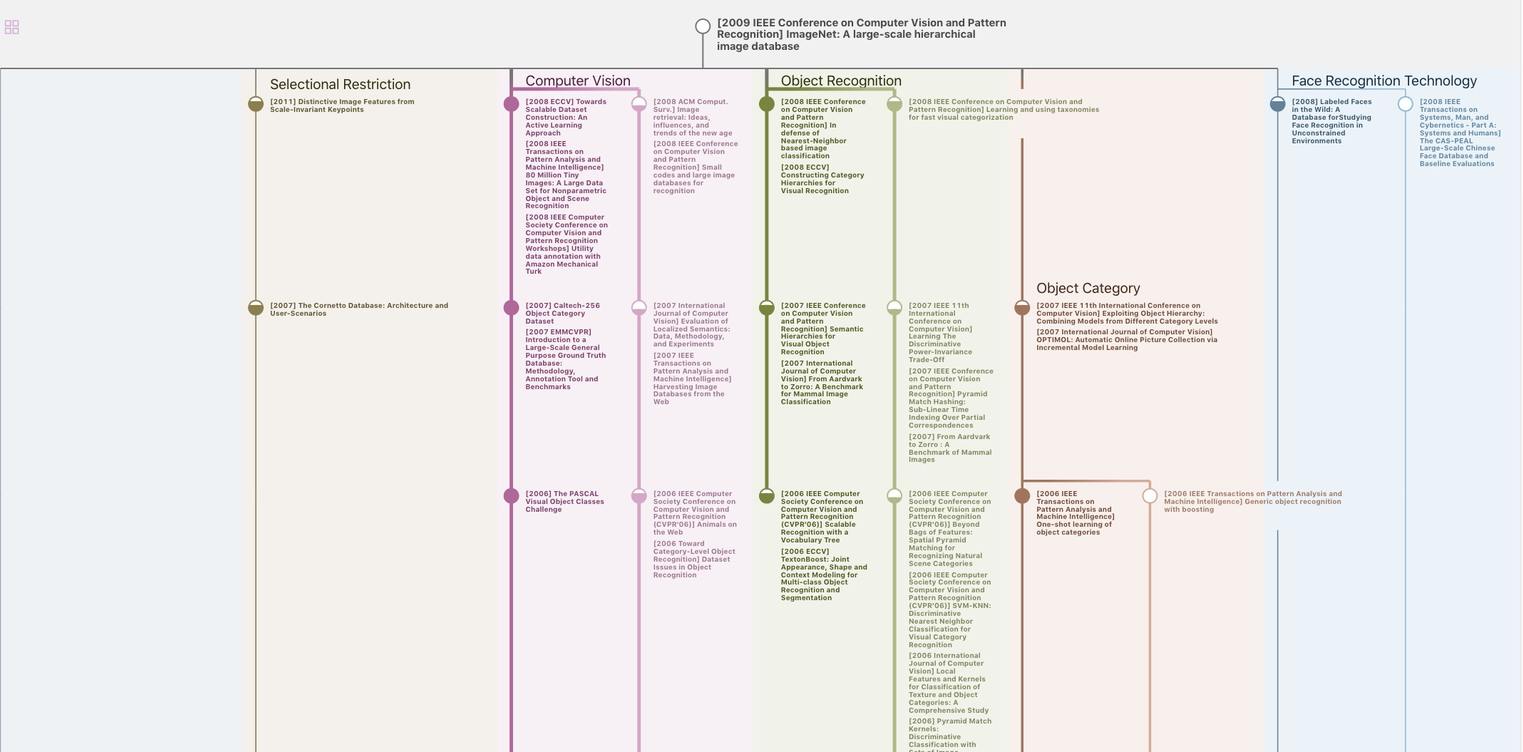
生成溯源树,研究论文发展脉络
Chat Paper
正在生成论文摘要