TESPDA-SEI: Tensor embedding substructure preserving domain adaptation for specific emitter identification.
Physical Communication(2023)
Abstract
For the specific emitter identification (SEI) with few or no labels, domain adaptation make the model respond quickly with the help of empirical information. However, the more extreme case is that there are so few labeled samples in the source domain that it is difficult to train an excellent recognition model. In fact, it is more valuable to make full use of these limited label information. This work aims at proposing an unsupervised domain adaptation (UDA)-based method to accommodate the typical case of no labels in the target domain and small samples in the source domain when new devices are first introduced. The basic principle is to learn tensor embedding shared feature space and preserving inter-class substructure, which perform feature space mapping under the joint source and target domain led by mapping error minimize in the source domain. Specifically, this tensor embedding substructure preserving domain adaptation (TESPDA) consist of three parts, tensor invariant subspace learning, substructure preserving feature space mapping and pseudo-label prediction, which are used to learn inter-class substructure after tensor space mapping and identify the predict labels for the target domain. Finally, experiments are conducted on the real-word ADS-B dataset to demonstrate the effectiveness of the TESPDA method.
MoreTranslated text
Key words
Unsupervised domain adaptation,Specific emitter identification,Tensor,Substructure preserving
AI Read Science
Must-Reading Tree
Example
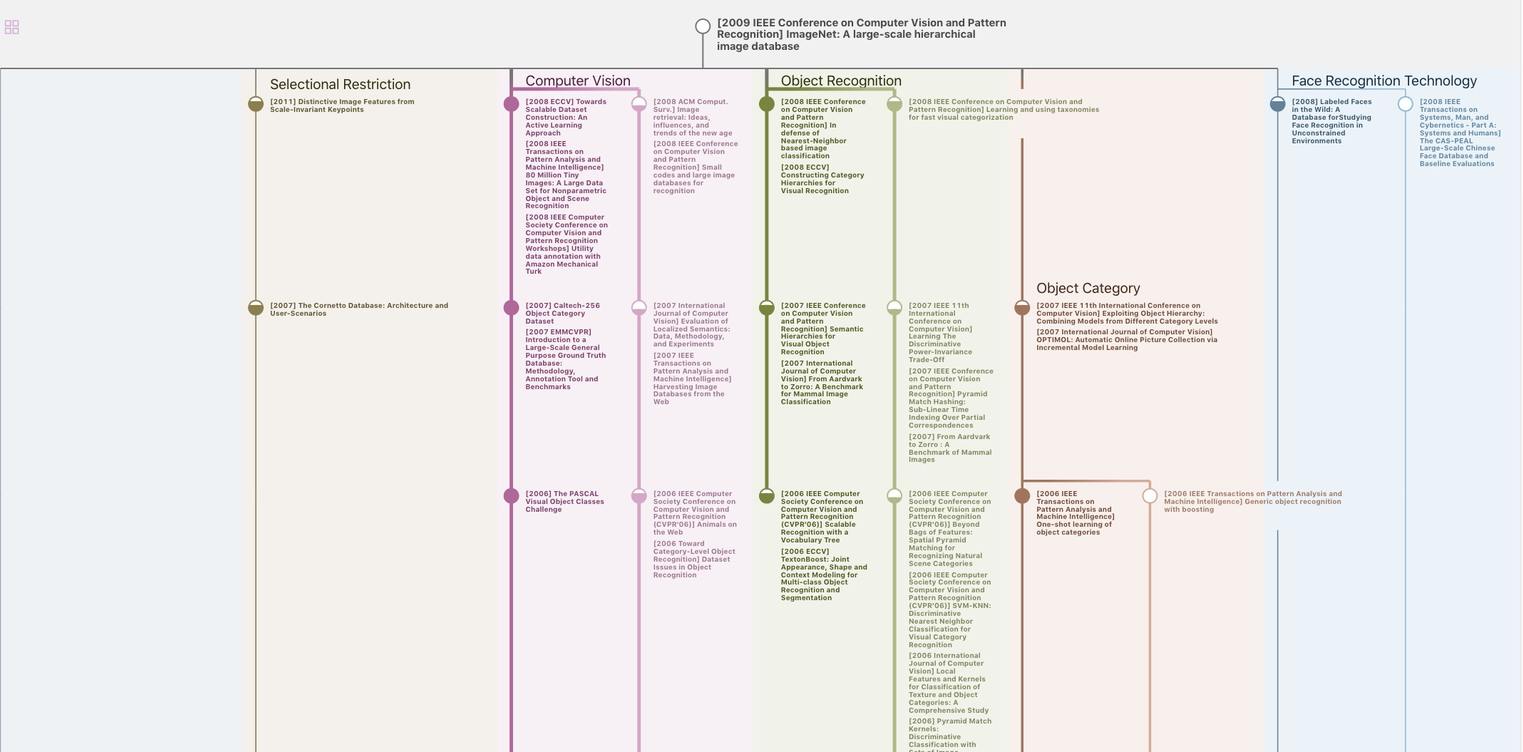
Generate MRT to find the research sequence of this paper
Chat Paper
Summary is being generated by the instructions you defined