Scheduling and Aggregation Design for Asynchronous Federated Learning over Wireless Networks
arxiv(2023)
摘要
Federated Learning (FL) is a collaborative machine learning (ML) framework that combines on-device training and server-based aggregation to train a common ML model among distributed agents. In this work, we propose an asynchronous FL design with periodic aggregation to tackle the straggler issue in FL systems. Considering limited wireless communication resources, we investigate the effect of different scheduling policies and aggregation designs on the convergence performance. Driven by the importance of reducing the bias and variance of the aggregated model updates, we propose a scheduling policy that jointly considers the channel quality and training data representation of user devices. The effectiveness of our channel-aware data-importance-based scheduling policy, compared with state-of-the-art methods proposed for synchronous FL, is validated through simulations. Moreover, we show that an ``age-aware'' aggregation weighting design can significantly improve the learning performance in an asynchronous FL setting.
更多查看译文
关键词
Federated Learning,asynchronous training,wireless networks,scheduling,aggregation
AI 理解论文
溯源树
样例
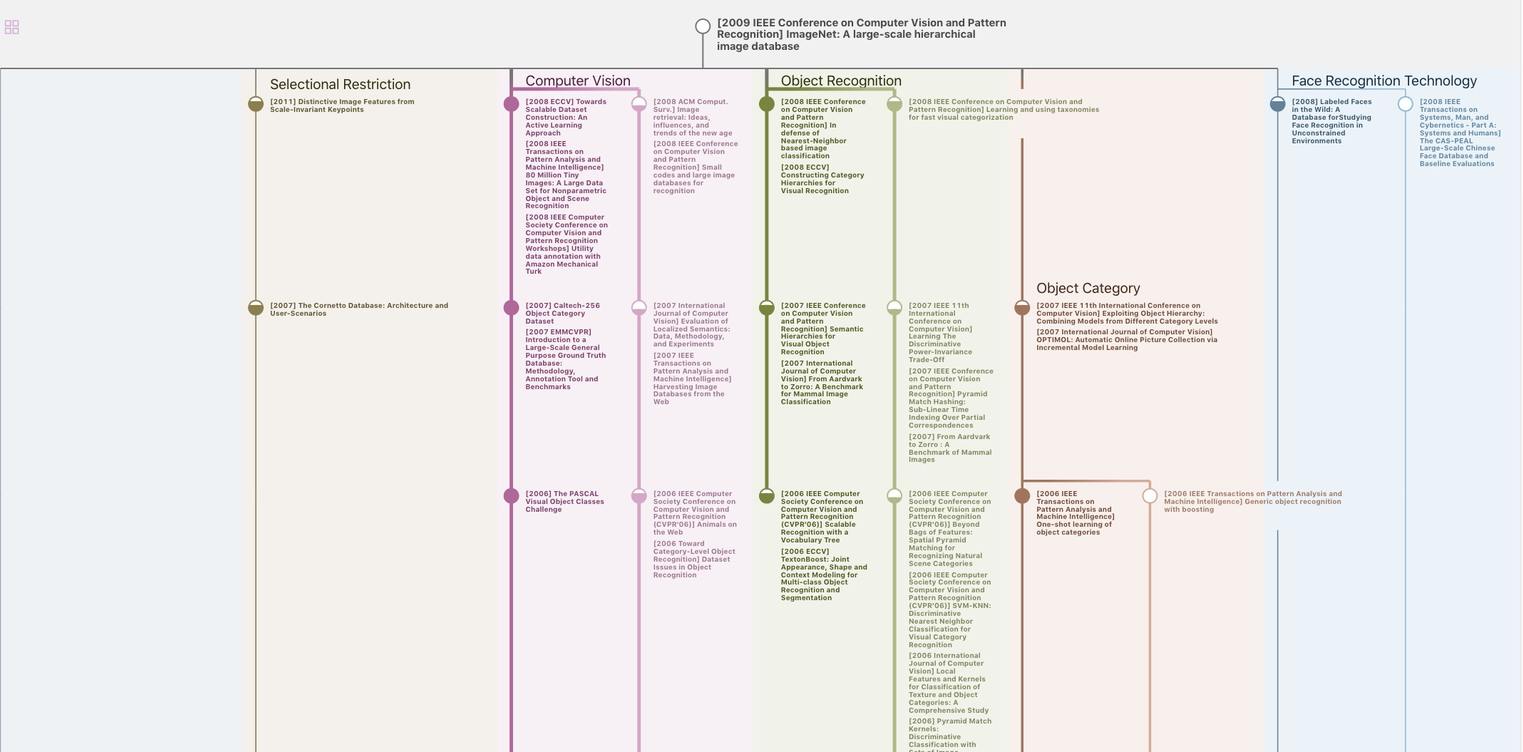
生成溯源树,研究论文发展脉络
Chat Paper
正在生成论文摘要