SECUREASTRAEA: A Self-balancing Privacy-preserving Federated Learning Framework
2022 IEEE Intl Conf on Dependable, Autonomic and Secure Computing, Intl Conf on Pervasive Intelligence and Computing, Intl Conf on Cloud and Big Data Computing, Intl Conf on Cyber Science and Technology Congress (DASC/PiCom/CBDCom/CyberSciTech)(2022)
摘要
Federated learning is an emerging fundamental AI technology originally used to solve the problem of updating models locally by Android cell phone end-users. As a distributed approach, its design goal is to carry out efficient and secure machine learning among multiple participants or multiple computing nodes. Specifically, federated learning can somewhat protect information security during data exchange, protect endpoint data and personal data privacy, and ensure legal compliance, which is also applicable to edge computing systems. However, unlike the common training dataset, the data distribution of the edge computing system is imbalanced which will introduce biases in the model training and cause a decrease in accuracy of federated learning applications. In this work, we analyze the privacy leakage problem of existing solutions. To address this problem, we construct a self-balancing federated learning framework by designing multiple protocols in the semi-honest and server collusion scenarios, respectively. The proposed framework enables privacy protection by transmitting data as ciphertext as well as computing to protect the privacy of the participants. Compared with FedAvg, the state-of-the-art FL algorithm, our scheme has a substantial improvement in accuracy on imbalanced MNIST dataset; and compared with the prior solutions, our scheme is able to avoid the privacy leakage of participants.
更多查看译文
关键词
Federated Learning,Privacy Protection,Class Imbalance,Homomorphic Encryption
AI 理解论文
溯源树
样例
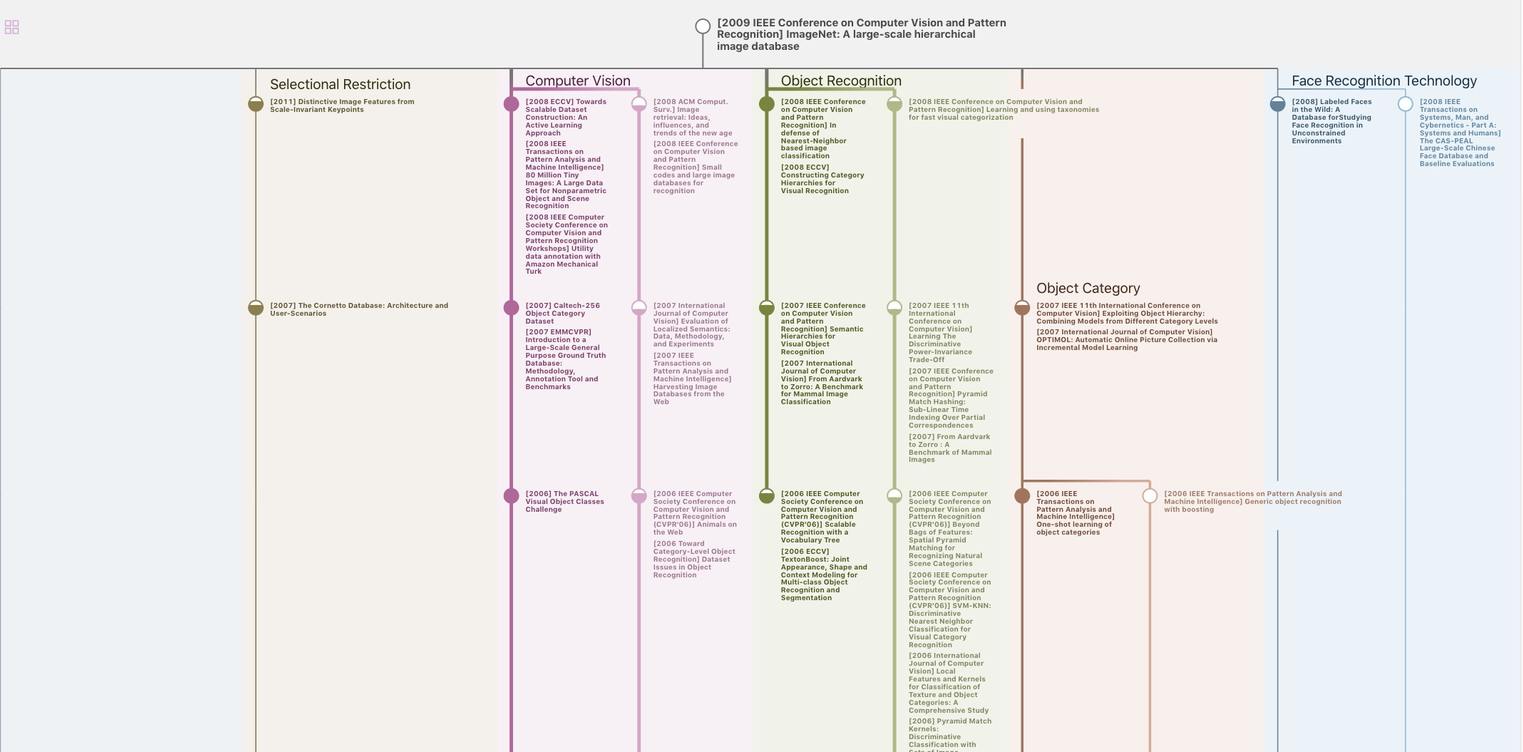
生成溯源树,研究论文发展脉络
Chat Paper
正在生成论文摘要