Contrastive Representation based Active Learning for Time Series
2022 IEEE Intl Conf on Dependable, Autonomic and Secure Computing, Intl Conf on Pervasive Intelligence and Computing, Intl Conf on Cloud and Big Data Computing, Intl Conf on Cyber Science and Technology Congress (DASC/PiCom/CBDCom/CyberSciTech)(2022)
摘要
Active Learning designs query strategies to select the most representative samples to be labeled by an oracle in an attempt to maximize the model’s performance while minimizing the labeling workload. We propose REAL, a new pooling-based active learning algorithm for time series data that learns the query strategy and optimizes the representation model in a contrastive manner. To initialize the process, a cluster module is employed to select the first sample set for labeling. Subsequent samples are selected through a contrastive loss function from three complementary perspectives, self-consistency, attraction to similar samples, and repulsion of disparate samples. Concurrently, the contrastive loss is also used to update the representation model. We evaluate our method on various time series classification tasks against state-of-the-art algorithms and demonstrate gains or comparable performance for an equal number of labeled samples.
更多查看译文
关键词
Contrastive learning,active learning,time series,representation,multi-view
AI 理解论文
溯源树
样例
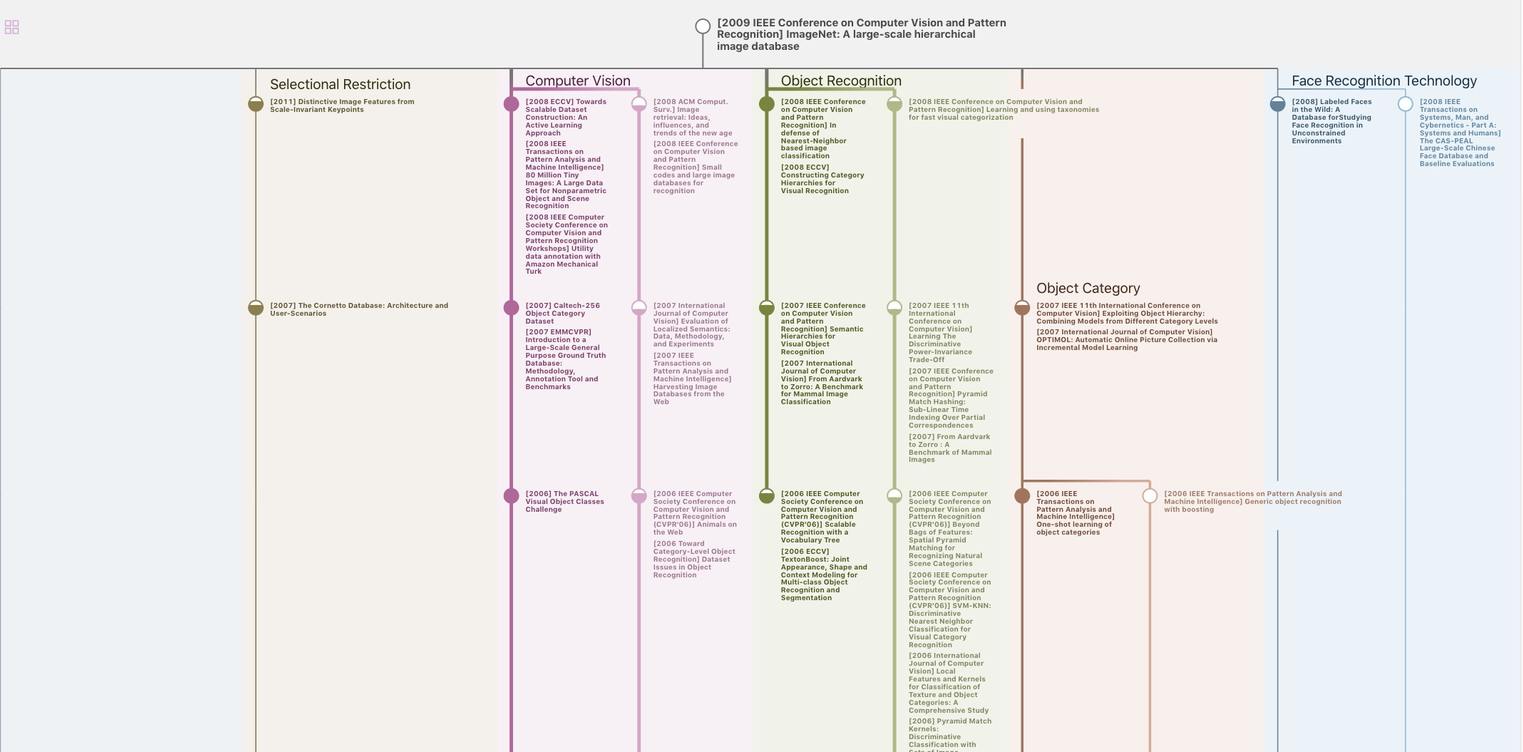
生成溯源树,研究论文发展脉络
Chat Paper
正在生成论文摘要