Motor Imagery EEG Classification with Self-attention-based Convolutional Neural Network
2022 7th International Conference on Intelligent Informatics and Biomedical Science (ICIIBMS)(2022)
摘要
Motor Imagery-based Brain-Computer Interfaces have been widely utilized in neuro-rehabilitation. Motor Imagery electroencephalogram (MI-EEG) refers to the EEG signals that people imagine their body moving without real action. People who have motor disorders can control the external devices through electroencephalogram (EEG)-decoding. However, there are still a variety of challenges in decoding due to the complexity and non-stationarity of EEG. How to improve the accuracy and robustness of EEG-decoding remains a key question to be studied. In this work, a self-attention-based convolutional neural network (CNN) combined with Frequency-Time Band Common Spatial Pattern (FTBCSP) is first introduced for the four-class MI-EEG classification. Self-attention-based CNN is employed on raw data to obtain the channel weights and intensify the spatial information. Common Spatial Pattern (CSP), an algorithm that is widely used in MI-EEG decoding, can extract discriminative features between two classes. Features after processing by the CSP algorithm are combined with the above spatial information to accomplish classifying. We validate this method on the publicly available multiclass MI datasets and yield a mean accuracy of 78.12% which performs better than other traditional methods. It proves that the proposed approach makes full use of the temporal and spatial information of EEG and acquires outstanding classification performance on public datasets.
更多查看译文
关键词
Motor Imagery,Brain-Computer Interface,Electroencephalgram (EEG),attention,Frequency-Time Band Common Spatial Pattern (FTBCSP )
AI 理解论文
溯源树
样例
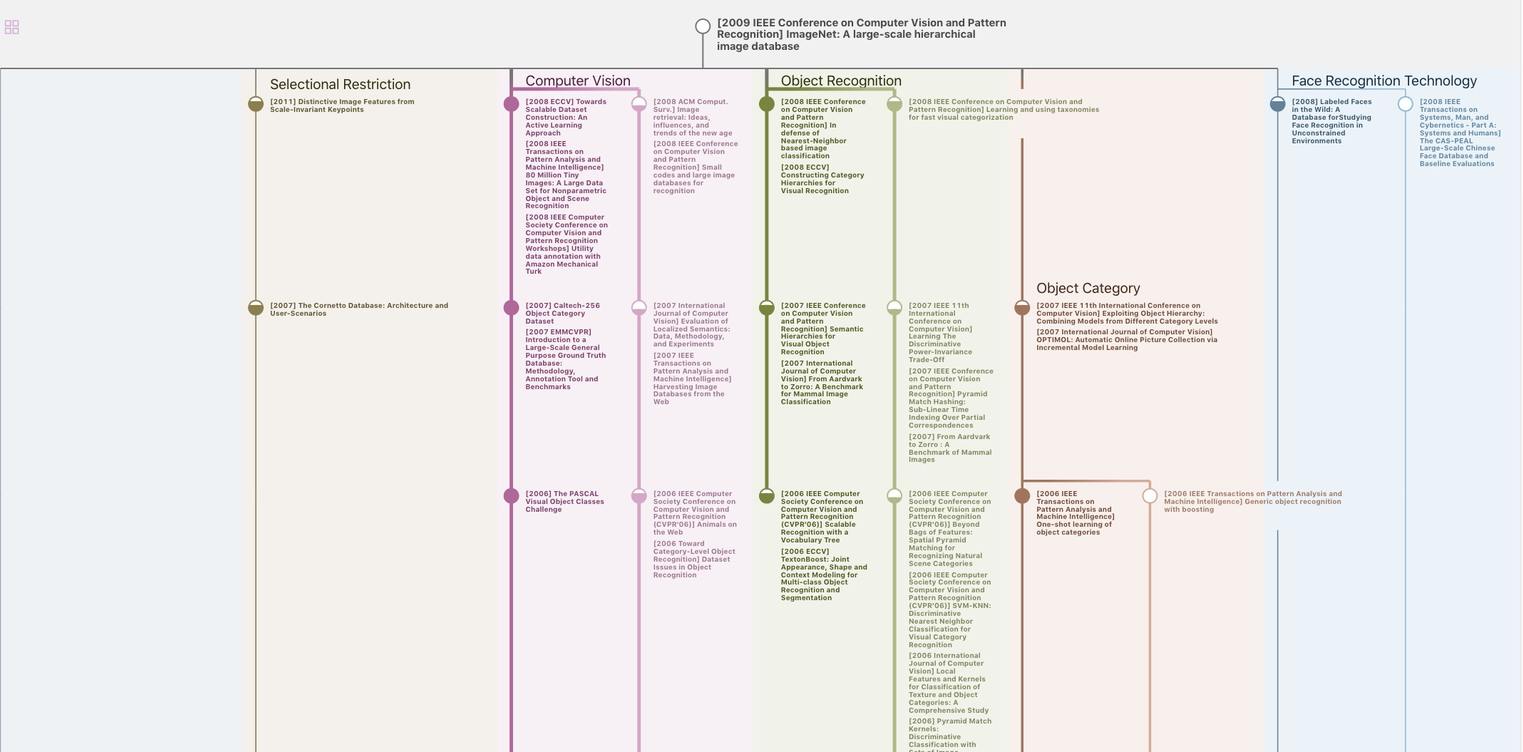
生成溯源树,研究论文发展脉络
Chat Paper
正在生成论文摘要