Nonparametric Independent Component Analysis for the Sources with Mixed Spectra
arxiv(2022)
摘要
Independent component analysis (ICA) is a blind source separation method to recover source signals of interest from their mixtures. Most existing ICA procedures assume independent sampling. Second-order-statistics-based source separation methods have been developed based on parametric time series models for the mixtures from the autocorrelated sources. However, the second-order-statistics-based methods cannot separate the sources accurately when the sources have temporal autocorrelations with mixed spectra. To address this issue, we propose a new ICA method by estimating spectral density functions and line spectra of the source signals using cubic splines and indicator functions, respectively. The mixed spectra and the mixing matrix are estimated by maximizing the Whittle likelihood function. We illustrate the performance of the proposed method through simulation experiments and an EEG data application. The numerical results indicate that our approach outperforms existing ICA methods, including SOBI algorithms. In addition, we investigate the asymptotic behavior of the proposed method.
更多查看译文
关键词
spectra
AI 理解论文
溯源树
样例
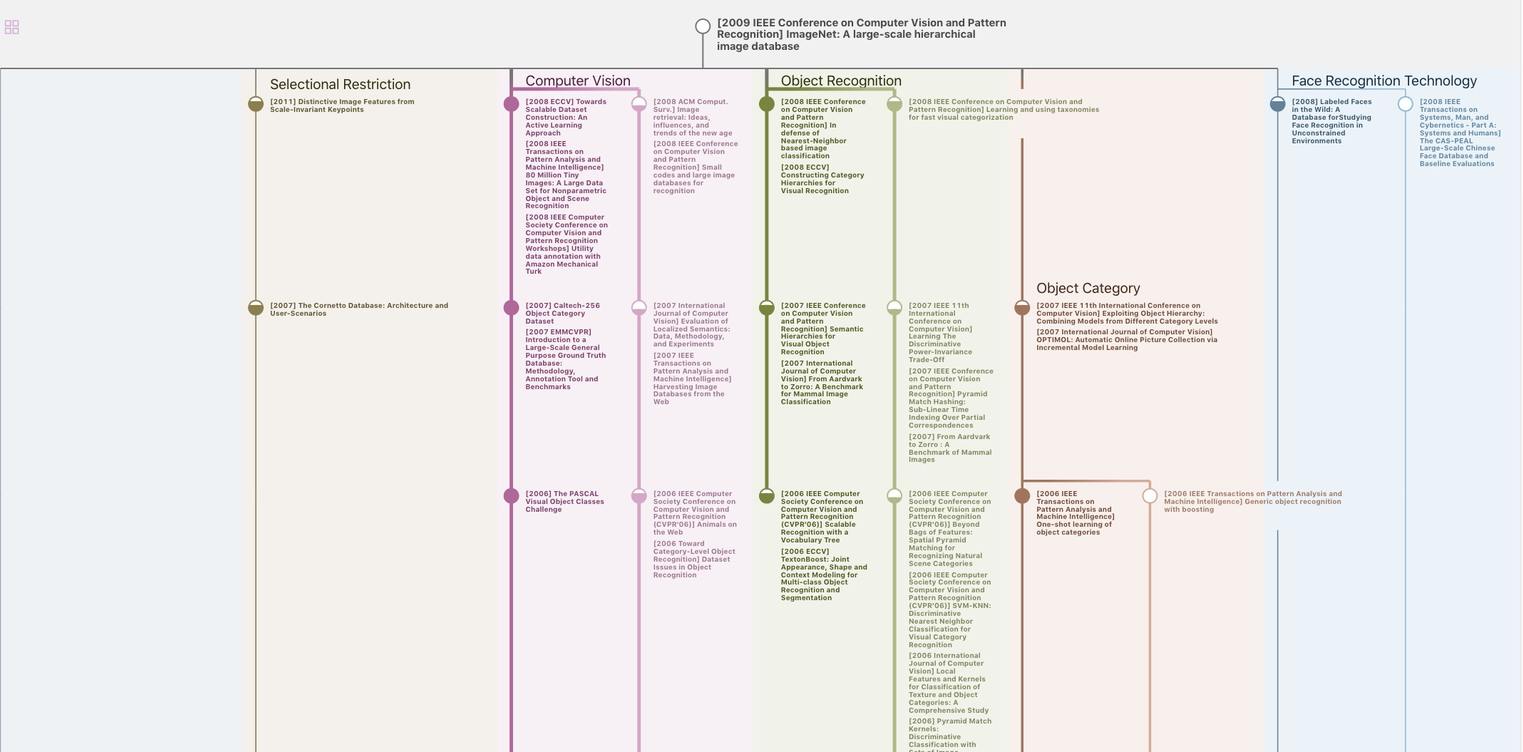
生成溯源树,研究论文发展脉络
Chat Paper
正在生成论文摘要