Breaking the "Object" in Video Object Segmentation
arxiv(2023)
摘要
The appearance of an object can be fleeting when it transforms. As eggs are broken or paper is torn, their color, shape and texture can change dramatically, preserving virtually nothing of the original except for the identity itself. Yet, this important phenomenon is largely absent from existing video object segmentation (VOS) benchmarks. In this work, we close the gap by collecting a new dataset for Video Object Segmentation under Transformations (VOST). It consists of more than 700 high-resolution videos, captured in diverse environments, which are 20 seconds long on average and densely labeled with instance masks. We adopt a careful, multi-step approach to ensure that these videos focus on complex object transformations, capturing their full temporal extent. We then extensively evaluate state-of-the-art VOS methods and make a number of important discoveries. In particular, we show that existing methods struggle when applied to this novel task and that their main limitation lies in over-reliance on static appearance cues. This motivates us to propose a few modifications for the topper-forming baseline that improve its capabilities by better modeling spatio-temporal information. More broadly, our work highlights the need for further research on learning more robust video object representations.
更多查看译文
关键词
Video: Low-level analysis,motion,tracking
AI 理解论文
溯源树
样例
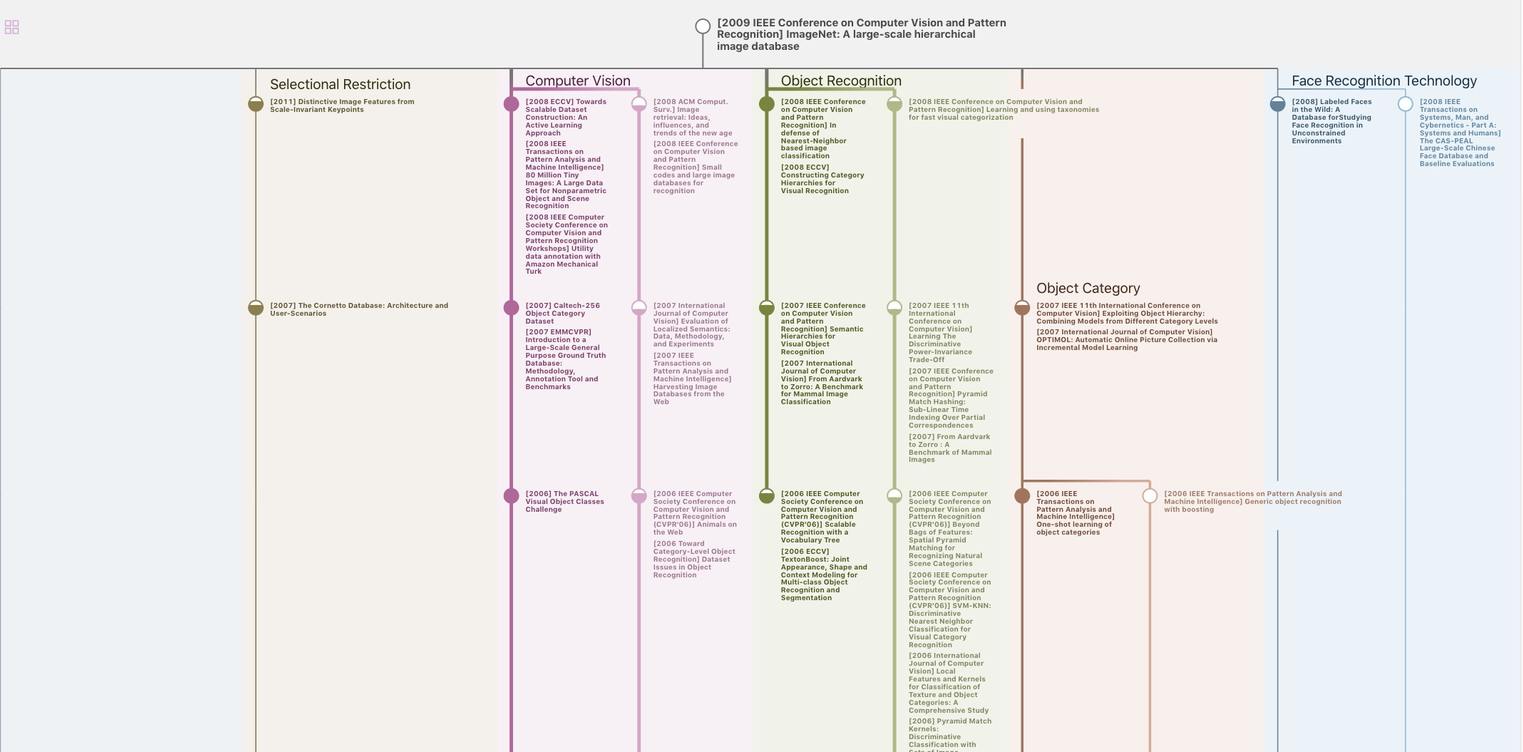
生成溯源树,研究论文发展脉络
Chat Paper
正在生成论文摘要