LRFFNet: Large Receptive Field Feature Fusion Network for Semantic Segmentation of SAR Images in Building Areas
Remote Sensing(2022)
摘要
There are limited studies on the semantic segmentation of high-resolution synthetic aperture radar (SAR) images in building areas due to speckle noise and geometric distortion. For this challenge, we propose the large receptive field feature fusion network (LRFFNet), which contains a feature extractor, a cascade feature pyramid module (CFP), a large receptive field channel attention module (LFCA), and an auxiliary branch. SAR images only contain single-channel information and have a low signal-to-noise ratio. Using only one level of features extracted by the feature extractor will result in poor segmentation results. Therefore, we design the CFP module; it can integrate different levels of features through multi-path connection. Due to the problem of geometric distortion in SAR images, the structural and semantic information is not obvious. In order to pick out feature channels that are useful for segmentation, we design the LFCA module, which can reassign the weight of channels through the channel attention mechanism with a large receptive field to help the network focus on more effective channels. SAR images do not include color information, and the identification of ground object categories is prone to errors, so we design the auxiliary branch. The branch uses the full convolution structure to optimize training results and reduces the phenomenon of recognizing objects outside the building area as buildings. Compared with state-of-the-art (SOTA) methods, our proposed network achieves higher scores in evaluation indicators and shows excellent competitiveness.
更多查看译文
关键词
synthetic aperture radar images,semantic segmentation,cascade feature pyramid module,large receptive field channel attention module,auxiliary branch
AI 理解论文
溯源树
样例
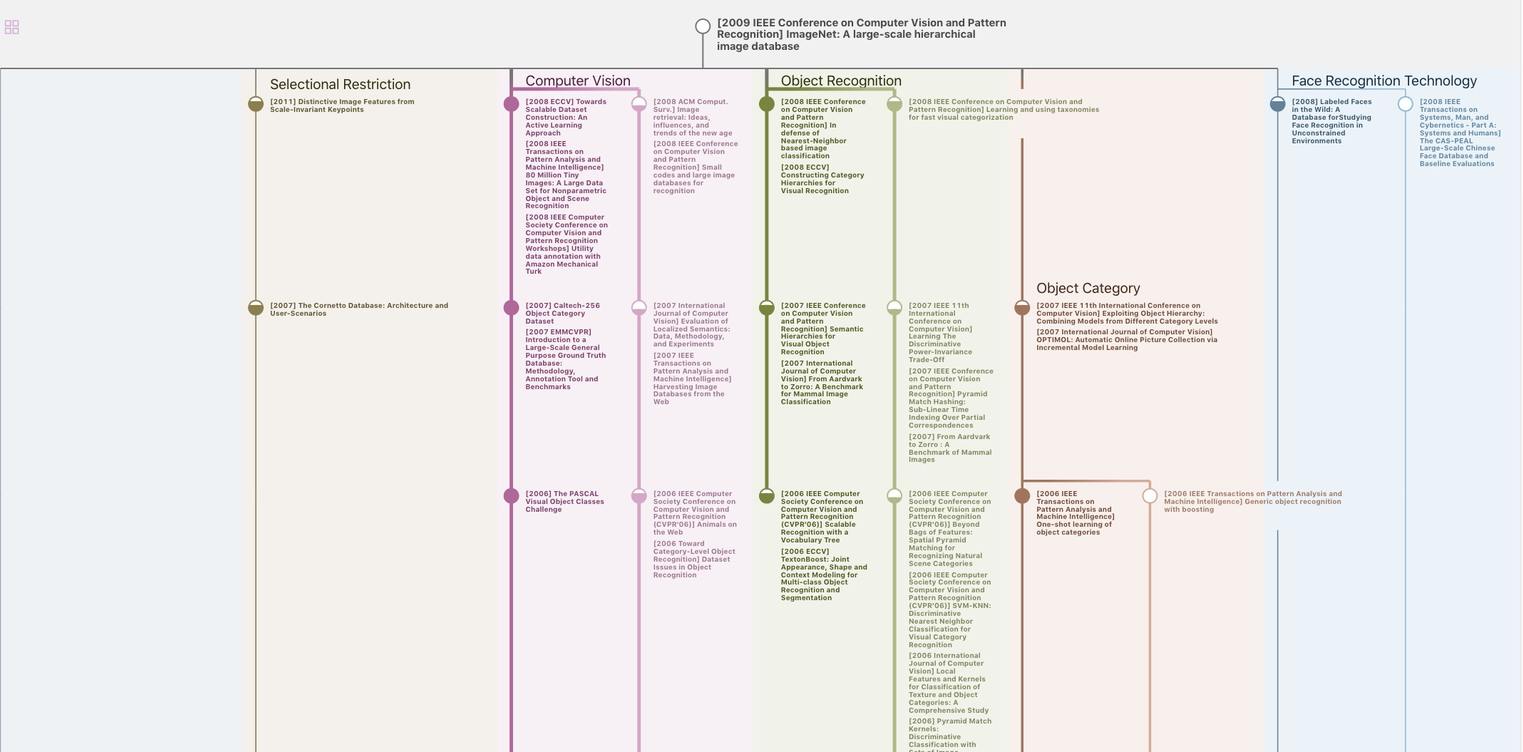
生成溯源树,研究论文发展脉络
Chat Paper
正在生成论文摘要