Generating Structurally Realistic Models With Deep Autoregressive Networks
IEEE Transactions on Software Engineering(2023)
摘要
Model generators are important tools in model-based systems engineering to automate the creation of software models for tasks like testing and benchmarking. Previous works have established four properties that a generator should satisfy: consistency, diversity, scalability, and structural realism. Although several generators have been proposed, none of them is focused on realism. As a result, automatically generated models are typically simple and appear synthetic. This work proposes a new architecture for model generators which is specifically designed to be structurally realistic. Given a dataset consisting of several models deemed as real models, this type of generators is able to produce new models which are structurally similar to the models in the dataset, but are fundamentally novel models. Our implementation, named ModelMime (M2), is based on a deep autoregressive model which combines a Graph Neural Network with a Recurrent Neural Network. We decompose each model into a sequence of edit operations, and the neural network is trained in the task of predicting the next edit operation given a partial model. At inference time, the system produces new models by sampling edit operations and iteratively completing the model. We have evaluated M2 with respect to three state-of-the-art generators, showing that 1) our generator outperforms the others in terms of the structurally realistic property 2) the models generated by M2 are most of the time consistent, 3) the diversity of the generated models is at least the same as the real ones and, 4) the generation process is scalable once the generator is trained.
更多查看译文
关键词
Generators,Object oriented modeling,Computational modeling,Task analysis,Predictive models,Measurement,Scalability,Model generators,realistic models,graph neural networks,recurrent neural networks,generative models
AI 理解论文
溯源树
样例
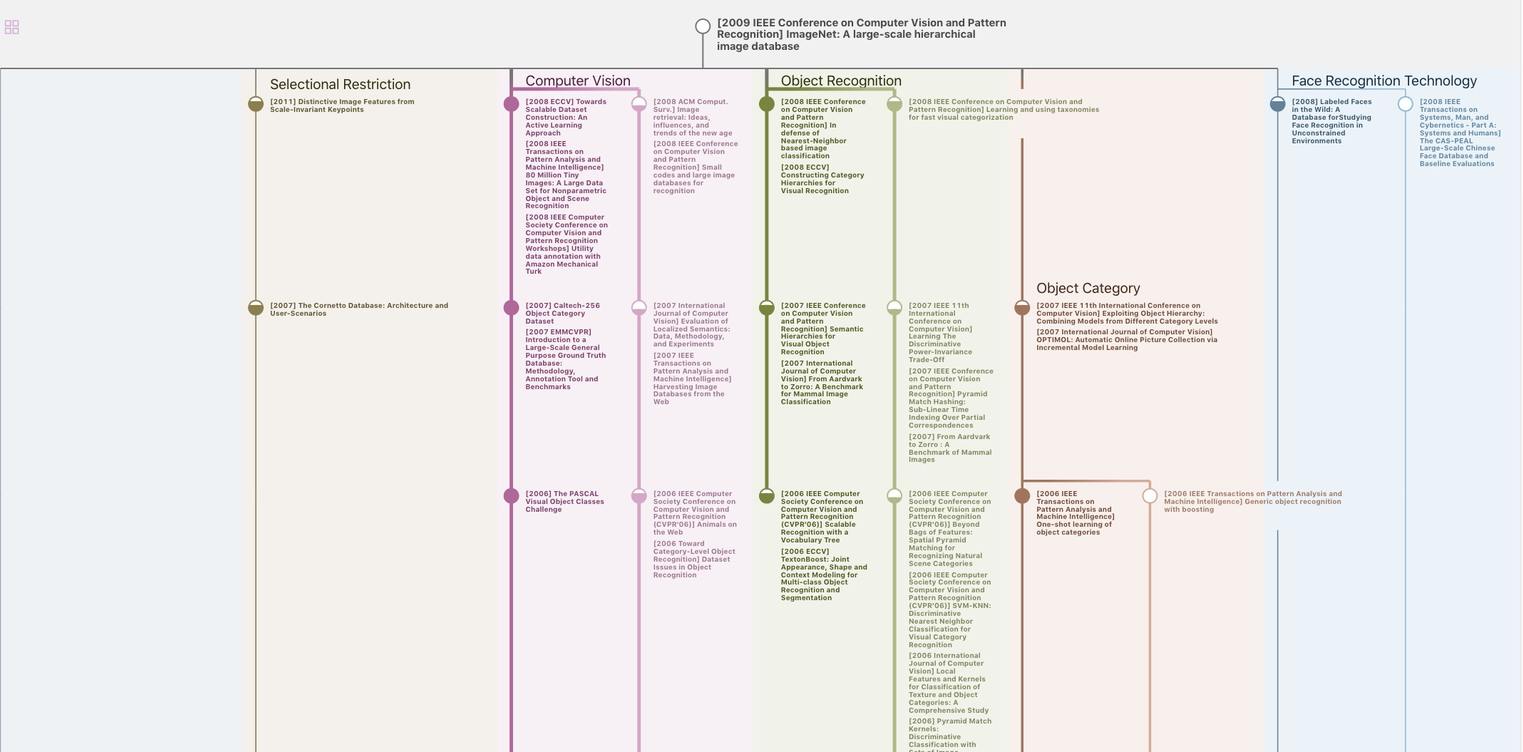
生成溯源树,研究论文发展脉络
Chat Paper
正在生成论文摘要