Improving Generalization of Pre-trained Language Models via Stochastic Weight Averaging
arxiv(2022)
摘要
Knowledge Distillation (KD) is a commonly used technique for improving the generalization of compact Pre-trained Language Models (PLMs) on downstream tasks. However, such methods impose the additional burden of training a separate teacher model for every new dataset. Alternatively, one may directly work on the improvement of the optimization procedure of the compact model toward better generalization. Recent works observe that the flatness of the local minimum correlates well with better generalization. In this work, we adapt Stochastic Weight Averaging (SWA), a method encouraging convergence to a flatter minimum, to fine-tuning PLMs. We conduct extensive experiments on various NLP tasks (text classification, question answering, and generation) and different model architectures and demonstrate that our adaptation improves the generalization without extra computation cost. Moreover, we observe that this simple optimization technique is able to outperform the state-of-the-art KD methods for compact models.
更多查看译文
关键词
language models,weight averaging,generalization,stochastic
AI 理解论文
溯源树
样例
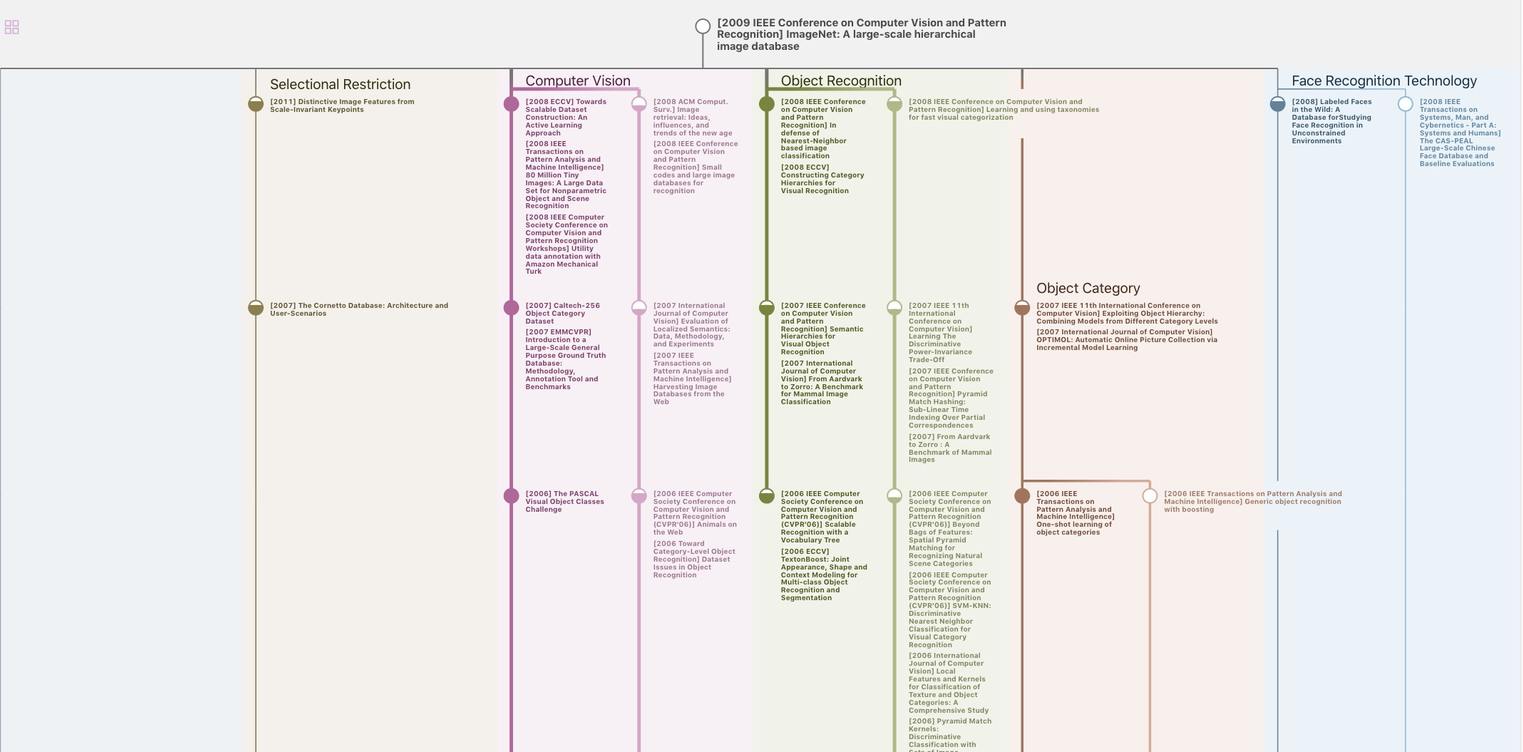
生成溯源树,研究论文发展脉络
Chat Paper
正在生成论文摘要