Learning on non-stationary data with re-weighting
arxiv(2022)
摘要
Many real-world learning scenarios face the challenge of slow concept drift, where data distributions change gradually over time. In this setting, we pose the problem of learning temporally sensitive importance weights for training data, in order to optimize predictive accuracy. We propose a class of temporal reweighting functions that can capture multiple timescales of change in the data, as well as instance-specific characteristics. We formulate a bi-level optimization criterion, and an associated meta-learning algorithm, by which these weights can be learned. In particular, our formulation trains an auxiliary network to output weights as a function of training instances, thereby compactly representing the instance weights. We validate our temporal reweighting scheme on a large real-world dataset of 39M images spread over a 9 year period. Our extensive experiments demonstrate the necessity of instance-based temporal reweighting in the dataset, and achieve significant improvements to classical batch-learning approaches. Further, our proposal easily generalizes to a streaming setting and shows significant gains compared to recent continual learning methods.
更多查看译文
关键词
learning,data,non-stationary,re-weighting
AI 理解论文
溯源树
样例
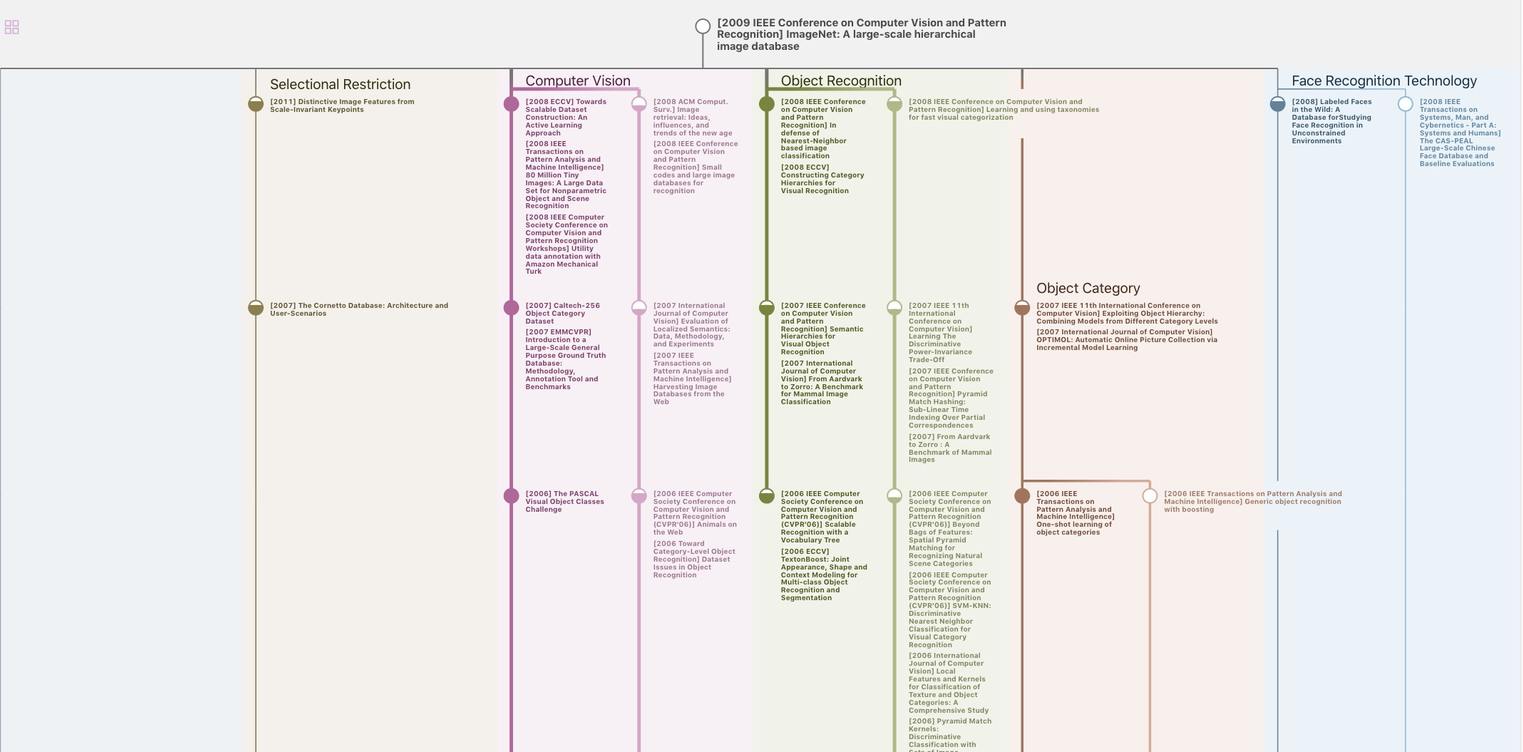
生成溯源树,研究论文发展脉络
Chat Paper
正在生成论文摘要