Multi-scale Feature Imitation for Unsupervised Anomaly Localization
CoRR(2022)
Abstract
The unsupervised anomaly localization task faces the challenge of missing anomaly sample training, detecting multiple types of anomalies, and dealing with the proportion of the area of multiple anomalies. A separate teacher-student feature imitation network structure and a multi-scale processing strategy combining an image and feature pyramid are proposed to solve these problems. A network module importance search method based on gradient descent optimization is proposed to simplify the network structure. The experimental results show that the proposed algorithm performs better than the feature modeling anomaly localization method on the real industrial product detection dataset in the same period. The multi-scale strategy can effectively improve the effect compared with the benchmark method.
MoreTranslated text
Key words
localization,multi-scale
AI Read Science
Must-Reading Tree
Example
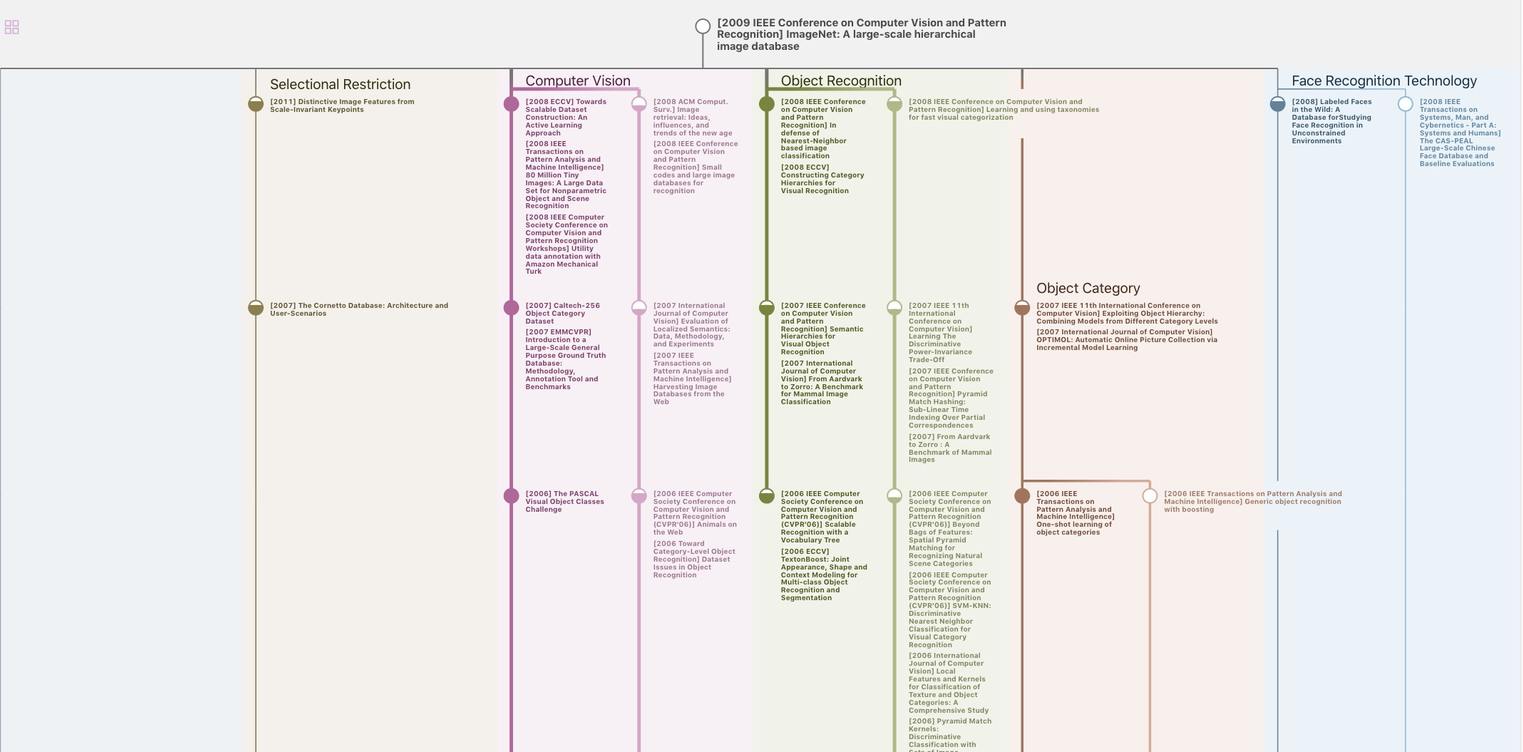
Generate MRT to find the research sequence of this paper
Chat Paper
Summary is being generated by the instructions you defined