Multi-Sem Fusion: Multimodal Semantic Fusion for 3D Object Detection
arxiv(2022)
摘要
LiDAR-based 3D Object detectors have achieved impressive performances in many benchmarks, however, multisensors fusion-based techniques are promising to further improve the results. PointPainting, as a recently proposed framework, can add the semantic information from the 2D image into the 3D LiDAR point by the painting operation to boost the detection performance. However, due to the limited resolution of 2D feature maps, severe boundary-blurring effect happens during re-projection of 2D semantic segmentation into the 3D point clouds. To well handle this limitation, a general multimodal fusion framework MSF has been proposed to fuse the semantic information from both the 2D image and 3D points scene parsing results. Specifically, MSF includes three main modules. First, SOTA off-the-shelf 2D/3D semantic segmentation approaches are employed to generate the parsing results for 2D images and 3D point clouds. The 2D semantic information is further re-projected into the 3D point clouds with calibrated parameters. To handle the misalignment between the 2D and 3D parsing results, an AAF module is proposed to fuse them by learning an adaptive fusion score. Then the point cloud with the fused semantic label is sent to the following 3D object detectors. Furthermore, we propose a DFF module to aggregate deep features in different levels to boost the final detection performance. The effectiveness of the framework has been verified on two public large-scale 3D object detection benchmarks by comparing with different baselines. The experimental results show that the proposed fusion strategies can significantly improve the detection performance compared to the methods using only point clouds and the methods using only 2D semantic information. Most importantly, the proposed approach significantly outperforms other approaches and sets new SOTA results on the nuScenes testing benchmark.
更多查看译文
AI 理解论文
溯源树
样例
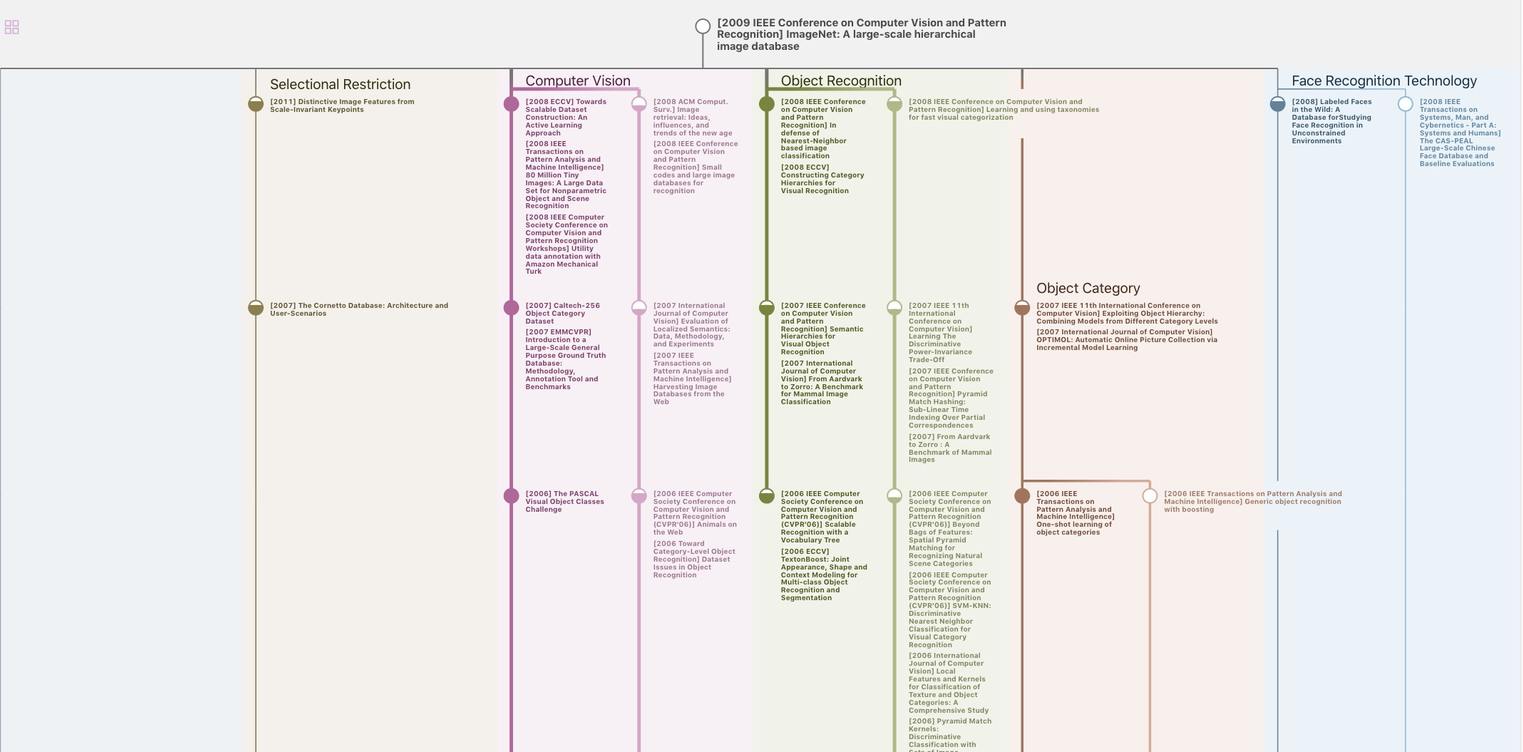
生成溯源树,研究论文发展脉络
Chat Paper
正在生成论文摘要