Mesh Neural Networks for SE(3)-Equivariant Hemodynamics Estimation on the Artery Wall
Computers in Biology and Medicine(2024)
Abstract
Computational fluid dynamics (CFD) is a valuable asset for patient-specificcardiovascular-disease diagnosis and prognosis, but its high computationaldemands hamper its adoption in practice. Machine-learning methods that estimateblood flow in individual patients could accelerate or replace CFD simulation toovercome these limitations. In this work, we consider the estimation ofvector-valued quantities on the wall of three-dimensional geometric arterymodels. We employ group equivariant graph convolution in an end-to-endSE(3)-equivariant neural network that operates directly on triangular surfacemeshes and makes efficient use of training data. We run experiments on a largedataset of synthetic coronary arteries and find that our method estimatesdirectional wall shear stress (WSS) with an approximation error of 7.6normalised mean absolute error (NMAE) of 0.4magnitude faster than CFD. Furthermore, we show that our method is powerfulenough to accurately predict transient, vector-valued WSS over the cardiaccycle while conditioned on a range of different inflow boundary conditions.These results demonstrate the potential of our proposed method as a pluginreplacement for CFD in the personalised prediction of hemodynamic vector andscalar fields.
MoreTranslated text
Key words
Graph convolutional networks,Group-equivariance,Computational fluid dynamics,Wall shear stress,Coronary arteries
AI Read Science
Must-Reading Tree
Example
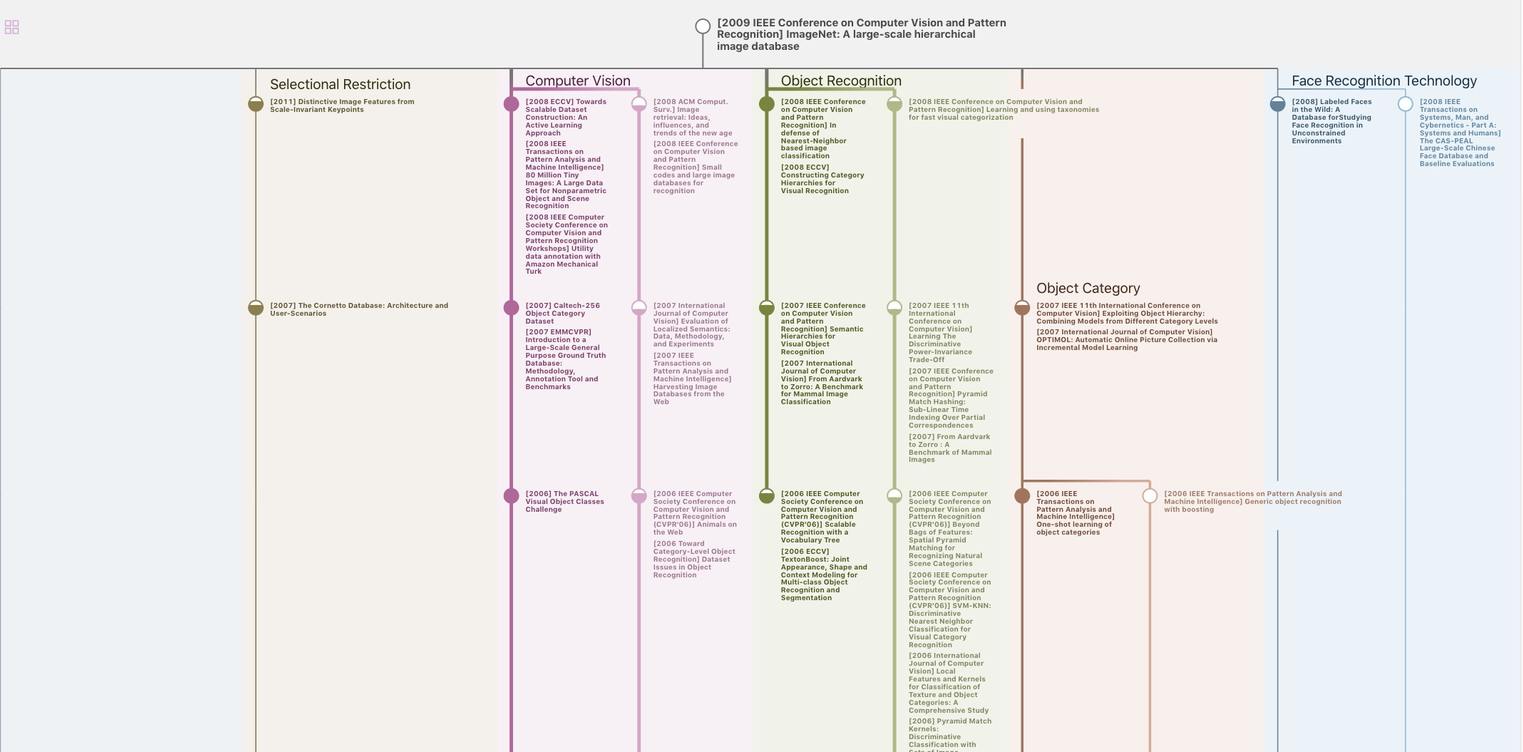
Generate MRT to find the research sequence of this paper
Chat Paper
Summary is being generated by the instructions you defined