Competency-Based Assessments: Leveraging Artificial Intelligence to Predict Subcompetency Content
Academic medicine : journal of the Association of American Medical Colleges(2023)
摘要
PurposeFaculty feedback on trainees is critical to guiding trainee progress in a competency-based medical education framework. The authors aimed to develop and evaluate a Natural Language Processing (NLP) algorithm that automatically categorizes narrative feedback into corresponding Accreditation Council for Graduate Medical Education Milestone 2.0 subcompetencies.MethodTen academic anesthesiologists analyzed 5,935 narrative evaluations on anesthesiology trainees at 4 graduate medical education (GME) programs between July 1, 2019, and June 30, 2021. Each sentence (n = 25,714) was labeled with the Milestone 2.0 subcompetency that best captured its content or was labeled as demographic or not useful. Inter-rater agreement was assessed by Fleiss' Kappa. The authors trained an NLP model to predict feedback subcompetencies using data from 3 sites and evaluated its performance at a fourth site. Performance metrics included area under the receiver operating characteristic curve (AUC), positive predictive value, sensitivity, F1, and calibration curves. The model was implemented at 1 site in a self-assessment exercise.ResultsFleiss' Kappa for subcompetency agreement was moderate (0.44). Model performance was good for professionalism, interpersonal and communication skills, and practice-based learning and improvement (AUC 0.79, 0.79, and 0.75, respectively). Subcompetencies within medical knowledge and patient care ranged from fair to excellent (AUC 0.66-0.84 and 0.63-0.88, respectively). Performance for systems-based practice was poor (AUC 0.59). Performances for demographic and not useful categories were excellent (AUC 0.87 for both). In approximately 1 minute, the model interpreted several hundred evaluations and produced individual trainee reports with organized feedback to guide a self-assessment exercise. The model was built into a web-based application.ConclusionsThe authors developed an NLP model that recognized the feedback language of anesthesiologists across multiple GME programs. The model was operationalized in a self-assessment exercise. It is a powerful tool which rapidly organizes large amounts of narrative feedback.
更多查看译文
关键词
artificial intelligence,assessments,predict,competency-based
AI 理解论文
溯源树
样例
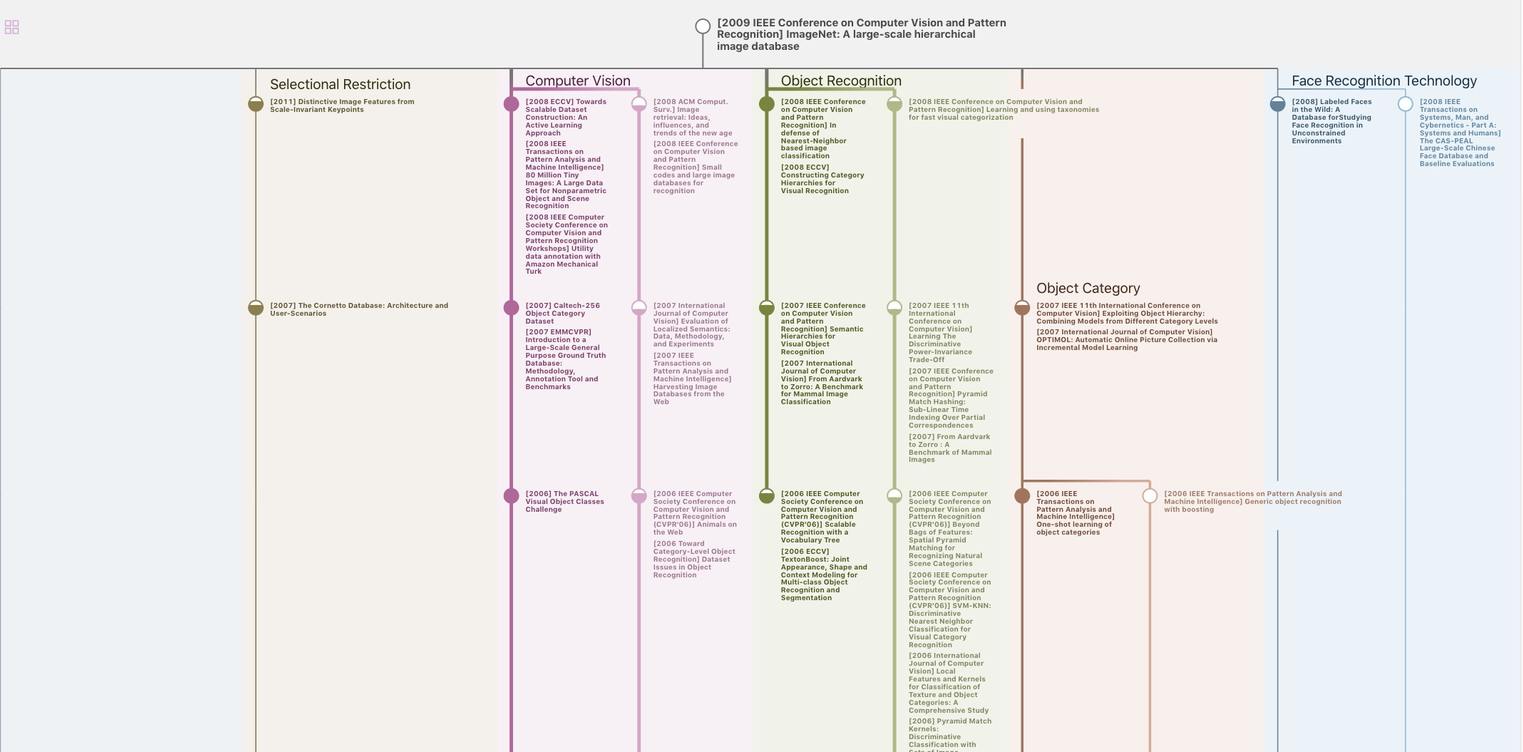
生成溯源树,研究论文发展脉络
Chat Paper
正在生成论文摘要