Deep-Learning Pipeline for Statistical Quantification of Amorphous Two-Dimensional Materials
ACS NANO(2022)
摘要
Aberration-corrected transmission electron microscopy enables imaging of two-dimensional (2D) materials with atomic resolution. However, dissecting the short-range-ordered structures in radiation-sensitive and amorphous 2D materials remains a significant challenge due to low atomic contrast and laborious manual evaluation. Here, we imaged carbon-based 2D materials with strong contrast, which is enabled by chromatic and spherical aberration correction at a low acceleration voltage. By constructing a deep-learning pipeline, atomic registry in amorphous 2D materials can be precisely determined, providing access to a full spectrum of quantitative data sets, including bond length/angle distribution, pair distribution function, and real-space polygon mapping. Accurate segmentation of micropores and surface contamination, together with robustness against background inhomogeneity, guaranteed the quantification validity in complex experimental images. The automated image analysis provides quantitative metrics with high efficiency and throughput, which may shed light on the structural understanding of short-range-ordered structures. In addition, the convolutional neural network can be readily generalized to crystalline materials, allowing for automatic defect identification and strain mapping.
更多查看译文
关键词
deep learning,neural networks,amorphous materials,two-dimensional materials,transmission electron microscopy,automated image evaluation
AI 理解论文
溯源树
样例
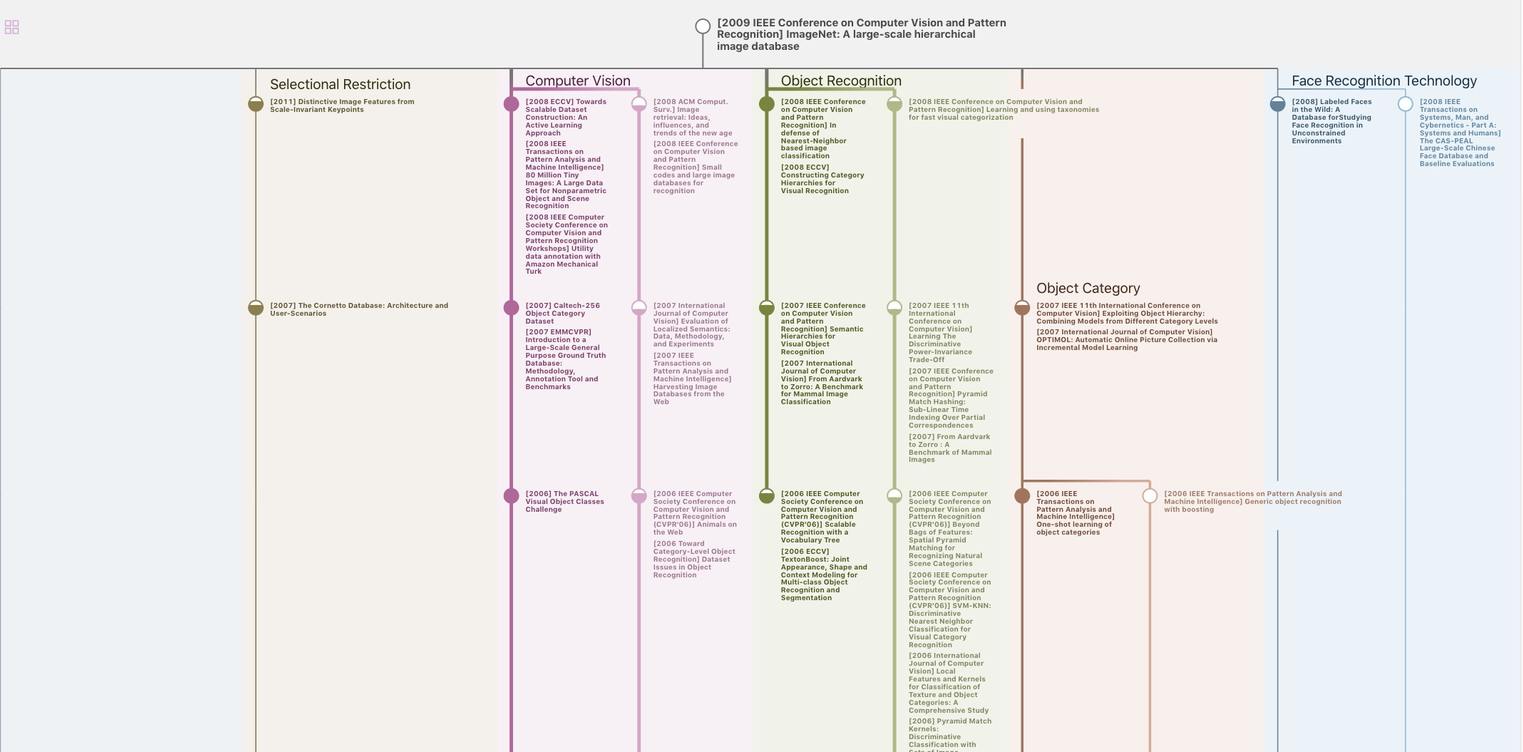
生成溯源树,研究论文发展脉络
Chat Paper
正在生成论文摘要