Exploiting Machine Learning for the Performance Analysis of a Mobile Hotspot with a Call Admission Control Mechanism
2022 IEEE 27th International Workshop on Computer Aided Modeling and Design of Communication Links and Networks (CAMAD)(2022)
摘要
Machine Learning (ML) algorithms can be efficiently employed to calculate various performance metrics in telecommunication systems showing comparable accuracy with analytical expressions while at the same time decreasing the computation time in several operational cases. In this paper, we examine the impact of six ML methods both on the accuracy of calculations and on the estimation time and benchmark them against an analytical formalism which solves a 2D Markov chain to estimate seven performance metrics in a vehicular system of a mobile hotspot. As a consequence, when using ML methods, we show that the computational complexity can be reduced, especially in cases where the system capacity is large and the computational complexity of the 2D Markov chain increases. More specifically, the proposed approach is applied in a dataset which comprises 100,000 operational cases, demonstrating a reduction of estimation time of more than two orders of magnitude while maintaining the average error less than 4.5%.
更多查看译文
关键词
machine learning,deep neural networks,machine learning aided performance estimation,vehicular communications
AI 理解论文
溯源树
样例
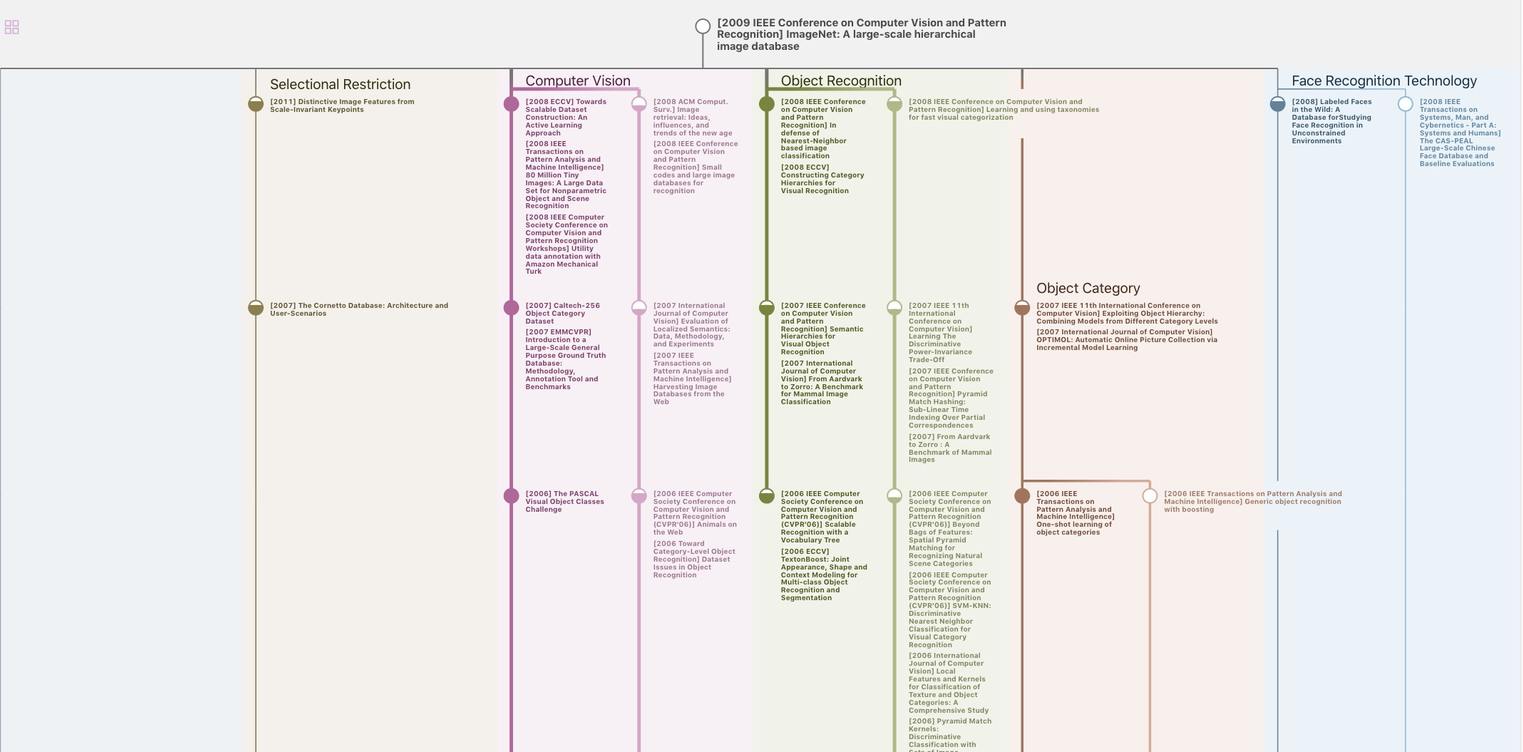
生成溯源树,研究论文发展脉络
Chat Paper
正在生成论文摘要