Strategies for Developing a Supervisory Controller with Deep Reinforcement Learning in a Production Context
2022 IEEE Conference on Control Technology and Applications (CCTA)(2022)
Abstract
Deep reinforcement learning (RL) algorithms are a promising optimisation tool in changing industrial production systems. We implement a supervisory controller using deep Q-learning and a Petri net simulation model. Furthermore, we identify challenges for using RL in a production context and propose three generally applicable strategies for using deep RL with production systems. Firstly, reward shaping may be used to deal with multiple goals and constraints, by allowing the RL agent to slowly adapt to the constraints. Secondly, an existing RL agent can be adapted to a different task using transfer learning and thus reducing training times. Lastly, including varying starting conditions increases the number of states the RL agent encounters during training. This increases the generalisation capabilities of the deep-Rlagent and allows the agent to react to unseen states more robustly. We present a setup for solving a sorting task using deep Q-learning and conduct several experiments to evaluate the proposed strategies.
MoreTranslated text
AI Read Science
Must-Reading Tree
Example
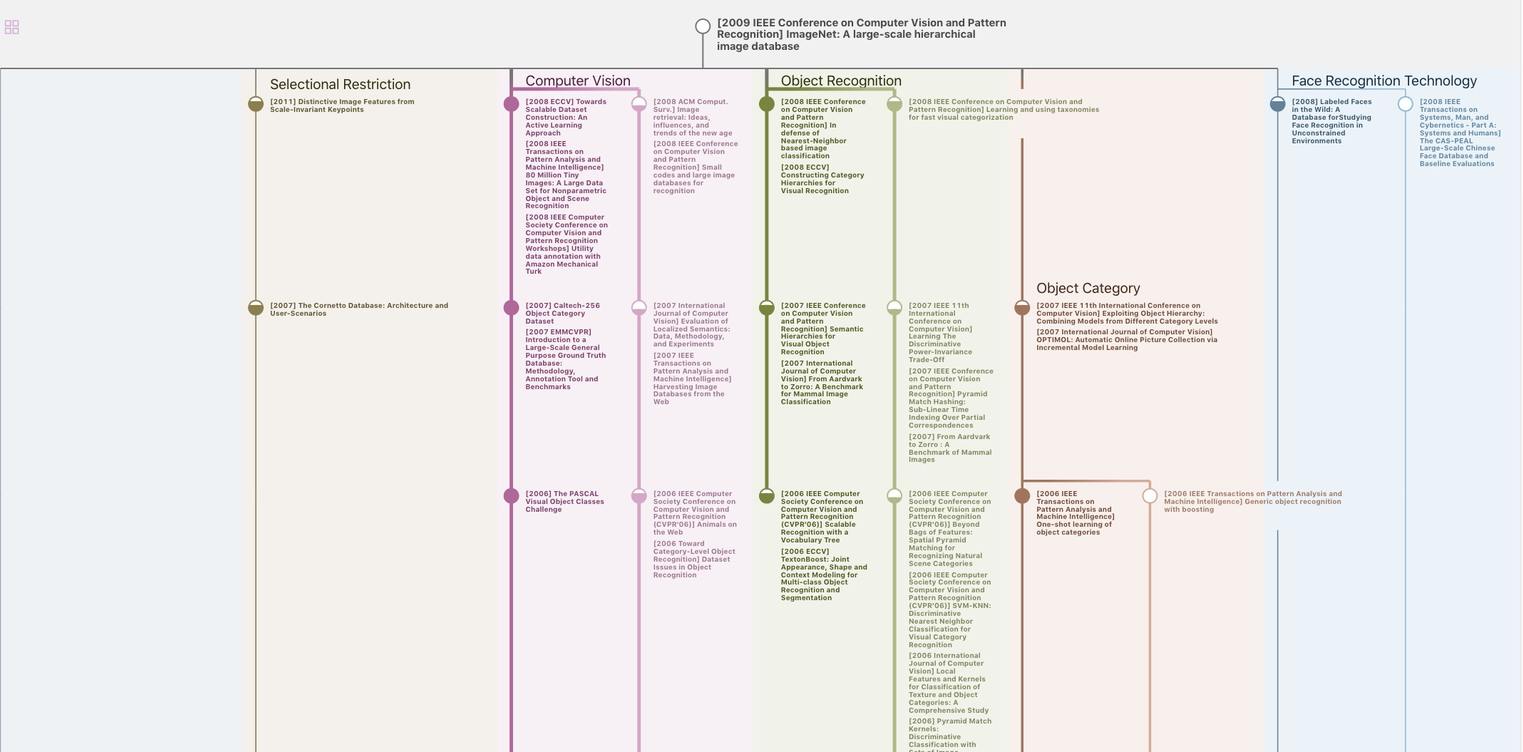
Generate MRT to find the research sequence of this paper
Chat Paper
Summary is being generated by the instructions you defined