Entropy minimizing distributions are worst-case optimal importance proposals
CoRR(2022)
摘要
Importance sampling of target probability distributions belonging to a given convex class is considered. Motivated by previous results, the cost of importance sampling is quantified using the relative entropy of the target with respect to proposal distributions. Using a reference measure as a reference for cost, we prove under some general conditions that the worst-case optimal proposal is precisely given by the distribution minimizing entropy with respect to the reference within the considered convex class of distributions. The latter conditions are in particular satisfied when the convex class is defined using a push-forward map defining atomless conditional measures. Applications in which the optimal proposal is Gibbsian and can be practically sampled using Monte Carlo methods are discussed.
更多查看译文
关键词
optimal importance
AI 理解论文
溯源树
样例
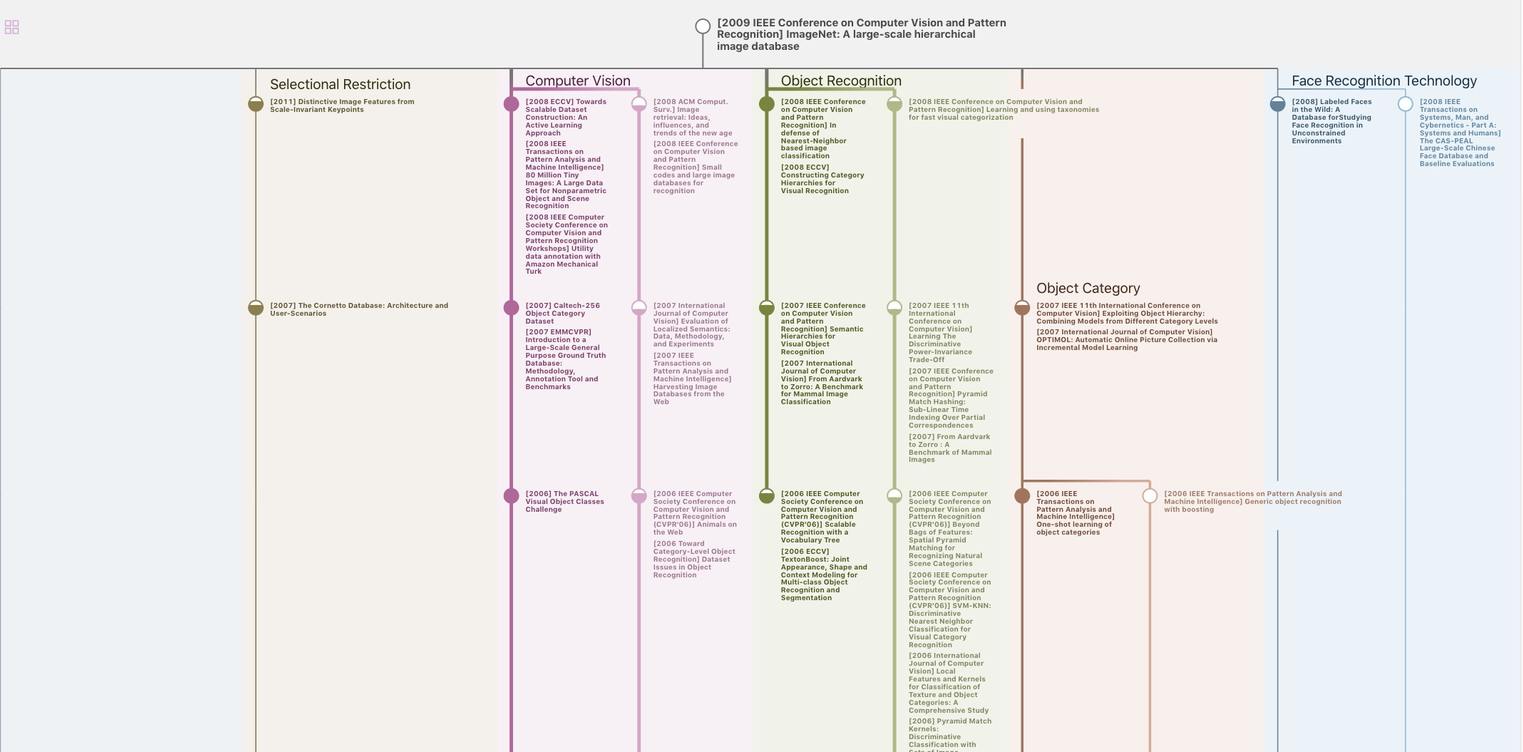
生成溯源树,研究论文发展脉络
Chat Paper
正在生成论文摘要