Talking Head Generation with Probabilistic Audio-to-Visual Diffusion Priors.
CoRR(2022)
摘要
We introduce a novel framework for one-shot audio-driven talking head generation. Unlike prior works that require additional driving sources for controlled synthesis in a deterministic manner, we instead sample all holistic lip-irrelevant facial motions (i.e. pose, expression, blink, gaze, etc.) to semantically match the input audio while still maintaining both the photo-realism of audio-lip synchronization and overall naturalness. This is achieved by our newly proposed audio-to-visual diffusion prior trained on top of the mapping between audio and non-lip representations. Thanks to the probabilistic nature of the diffusion prior, one big advantage of our framework is it can synthesize diverse facial motion sequences given the same audio clip, which is quite user-friendly for many real applications. Through comprehensive evaluations of public benchmarks, we conclude that (1) our diffusion prior outperforms auto-regressive prior significantly on all the concerned metrics; (2) our overall system is competitive with prior works in terms of audio-lip synchronization but can effectively sample rich and natural-looking lip-irrelevant facial motions while still semantically harmonized with the audio input.
更多查看译文
AI 理解论文
溯源树
样例
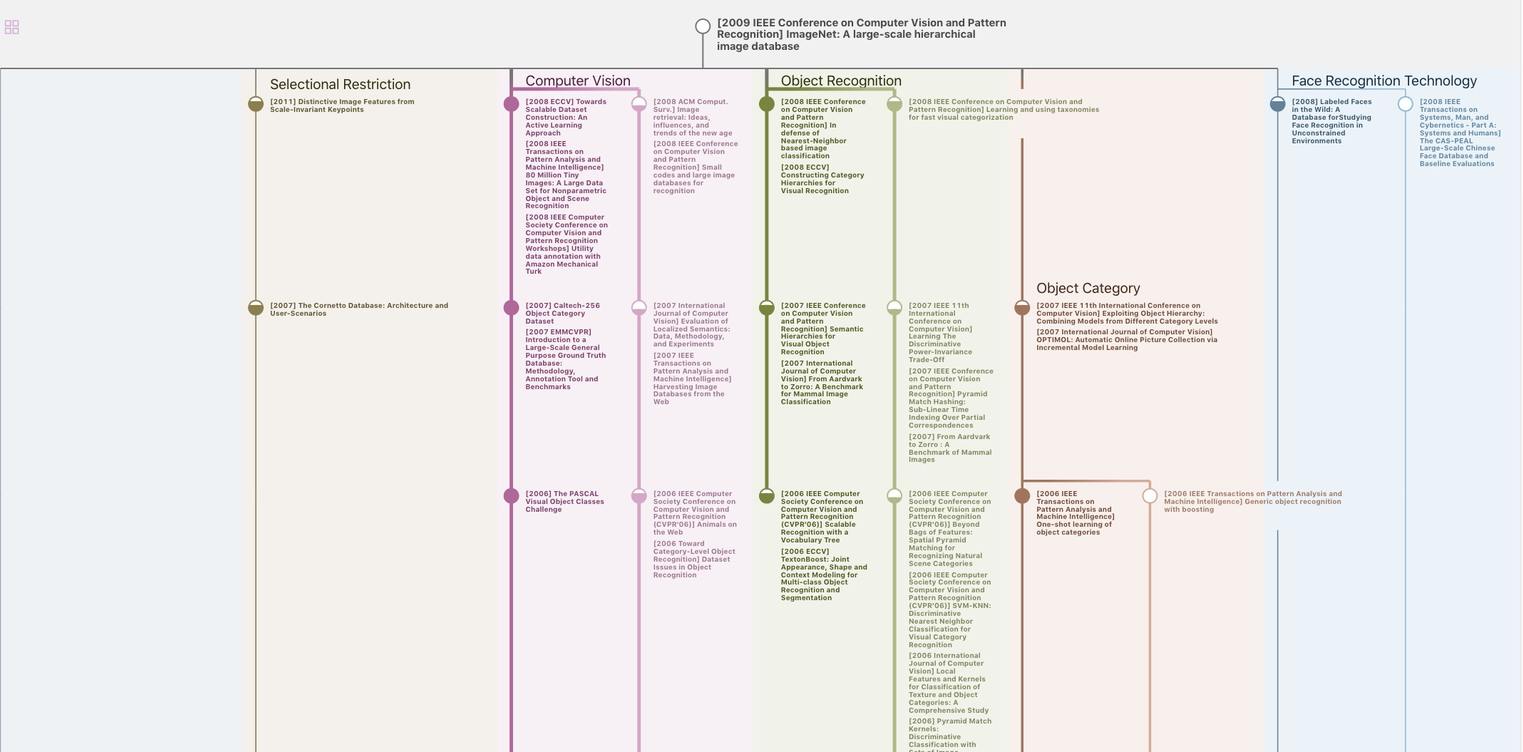
生成溯源树,研究论文发展脉络
Chat Paper
正在生成论文摘要