Considerations in Bayesian agent-based modeling for the analysis of COVID-19 data
STATISTICAL ANALYSIS AND DATA MINING(2024)
摘要
Agent-based model (ABM) has been widely used to study infectious disease transmission by simulating behaviors and interactions of autonomous individuals called agents. In the ABM, agent states, for example infected or susceptible, are assigned according to a set of simple rules, and a complex dynamics of disease transmission is described by the collective states of agents over time. Despite the flexibility in real-world modeling, ABMs have received less attention by statisticians because of the intractable likelihood functions which lead to difficulty in estimating parameters and quantifying uncertainty around model outputs. To overcome this limitation, a Bayesian framework that treats the entire ABM as a Hidden Markov Model has been previously proposed. However, existing approach is limited due to computational inefficiency and unidentifiability of parameters. We extend the ABM approach within Bayesian framework to study infectious disease transmission addressing these limitations. We estimate the hidden states, represented by individual agent's states over time, and the model parameters by applying an improved particle Markov Chain Monte Carlo algorithm, that accounts for computing efficiency. We further evaluate the performance of the approach for parameter recovery and prediction, along with sensitivity to prior assumptions under various simulation conditions. Finally, we apply the proposed approach to the study of COVID-19 outbreak on Diamond Princess cruise ship. We examine the differences in transmission by key demographic characteristics, while considering two different networks and limited COVID-19 testing in the cruise.
更多查看译文
关键词
agent-based model,Bayesian inference,compartmental model,hidden Markov model,particle filter
AI 理解论文
溯源树
样例
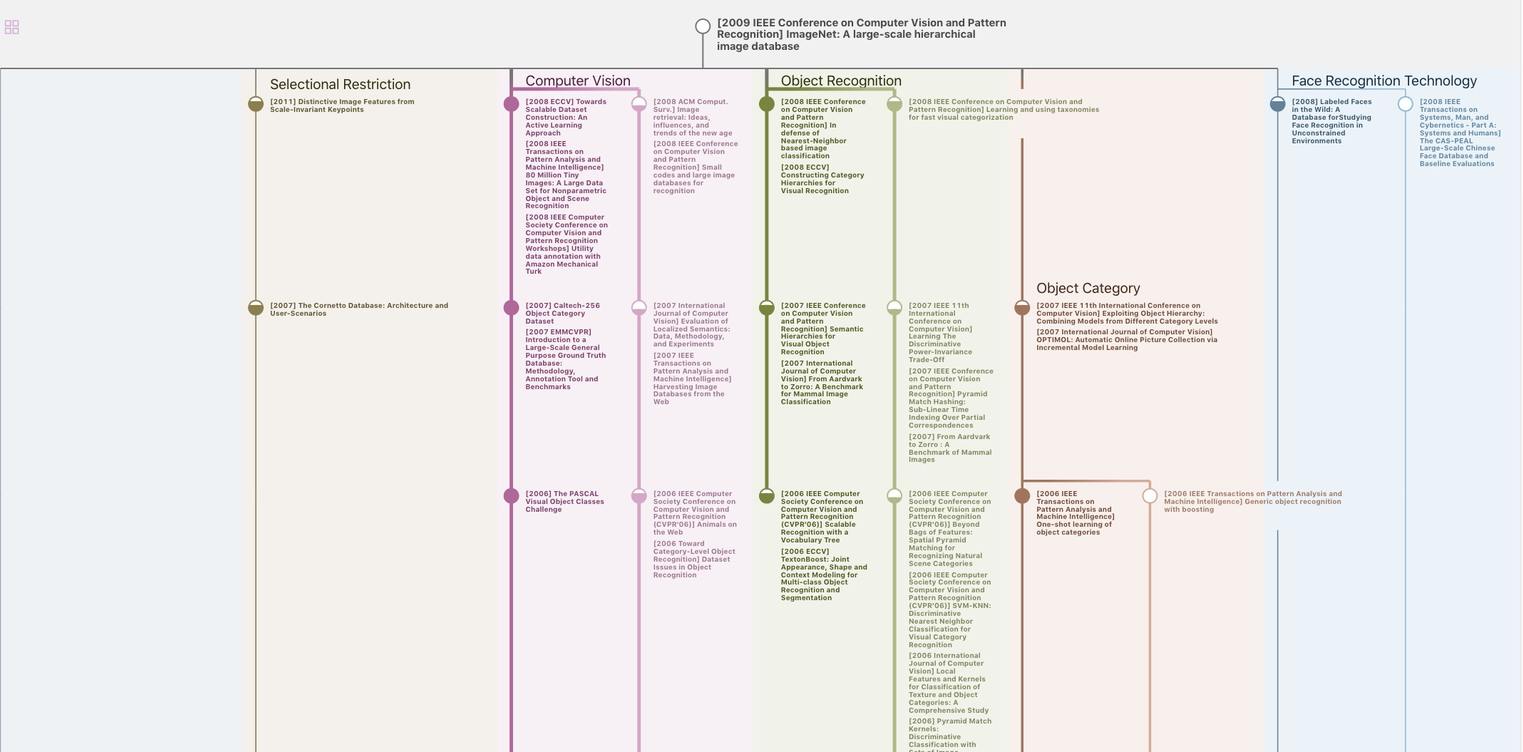
生成溯源树,研究论文发展脉络
Chat Paper
正在生成论文摘要