Spatial-Temporal Separable Attention for Video Action Recognition
2022 International Conference on Frontiers of Artificial Intelligence and Machine Learning (FAIML)(2022)
Abstract
Convolutional neural networks (CNNs) have been proved as a efficient method for various of visual recognition tasks. However, it is more difficult for CNNs to capture long-range spatial-temporal cues in dynamic videos than in static images. Recent nonlocal neural networks attempt to overcome this problem by a self-attention mechanism, where pair-wise affinities for all the spatial-temporal positions are calculated. However, this introduces a substantial computational burden. In this paper, we propose a spatial-temporal separable attention module (STSAM) to reduce the computational complexity. The experimental results, based on the Kinetics 400 benchmark, show that our model achieves better performance but introduces less extra FLOPs than nonlocal neural networks.
MoreTranslated text
Key words
video action recognition,attention mechanism,nonlocal neural networks,convolutional neural network
AI Read Science
Must-Reading Tree
Example
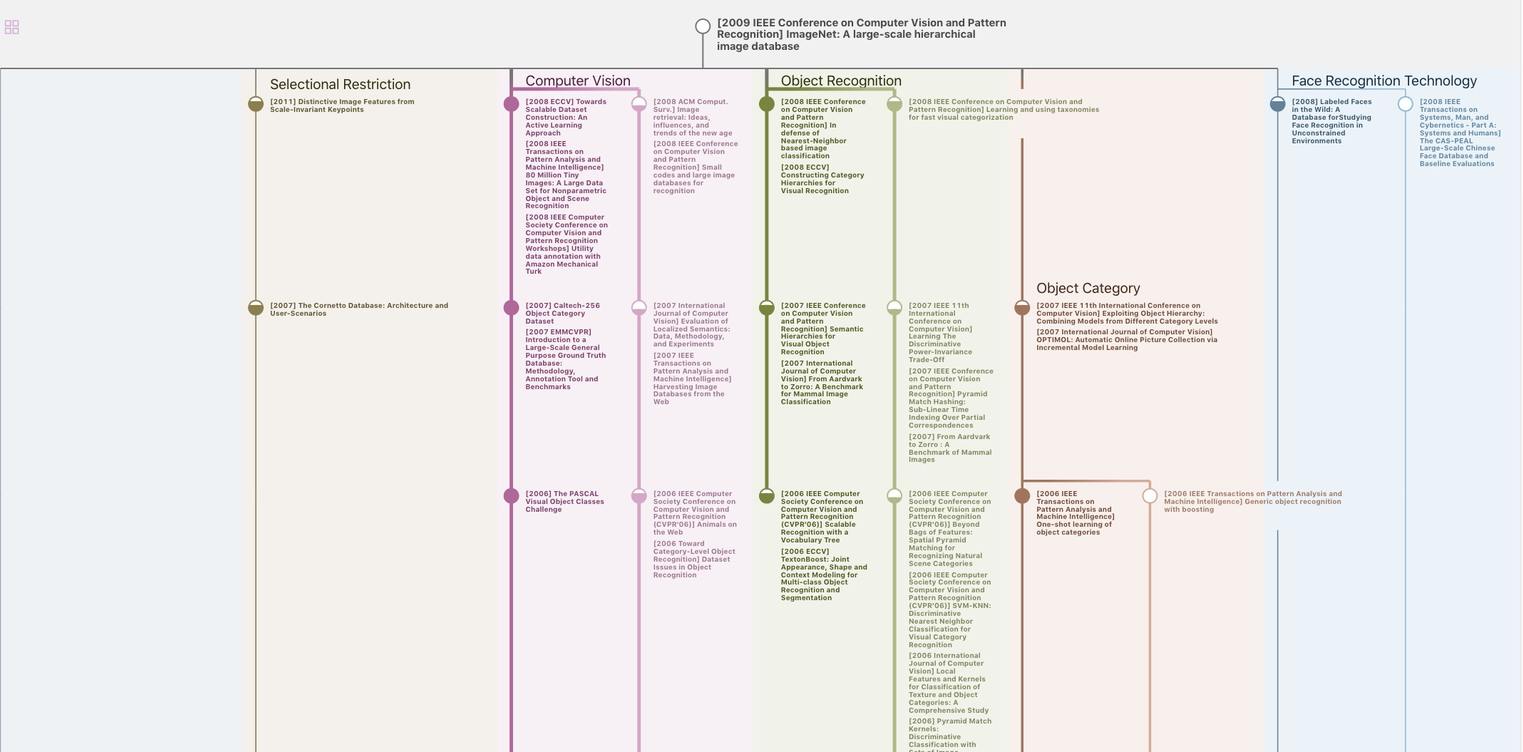
Generate MRT to find the research sequence of this paper
Chat Paper
Summary is being generated by the instructions you defined