A short-term building energy consumption prediction and diagnosis using deep learning algorithms
JOURNAL OF INTELLIGENT & FUZZY SYSTEMS(2022)
Abstract
Short-term energy consumption prediction of buildings is crucial for developing model-based predictive control, fault detection, and diagnosis methods. This study takes a university library in Xi'an as the research object. First, a time-by-time energy consumption prediction model is established under the supervised learning approach, which uses a long short-term memory (LSTM) network and a Multi-Input Multi-Output (MIMO) strategy. The experimental results validate the model's validity, which is close enough to physical reality for engineering purposes. Second, the potential of the people flows factor in energy consumption prediction models is explored. The results show that people flow has great potential in predicting building energy consumption and can effectively improve the prediction model performance. Third, a diagnostic method, which can recognize abnormal energy consumption data is used to diagnose the unreasonable use of the building during each hour of operation. The method is based on differences between actual and predicted energy consumption data derived from a short-term energy consumption prediction model. Based on actual building operation data, this work is enlightening and can serve as a reference for building energy efficiency management and operation.
MoreTranslated text
Key words
Deep learning,energy consumption prediction,energy consumption diagnosis,people flows
AI Read Science
Must-Reading Tree
Example
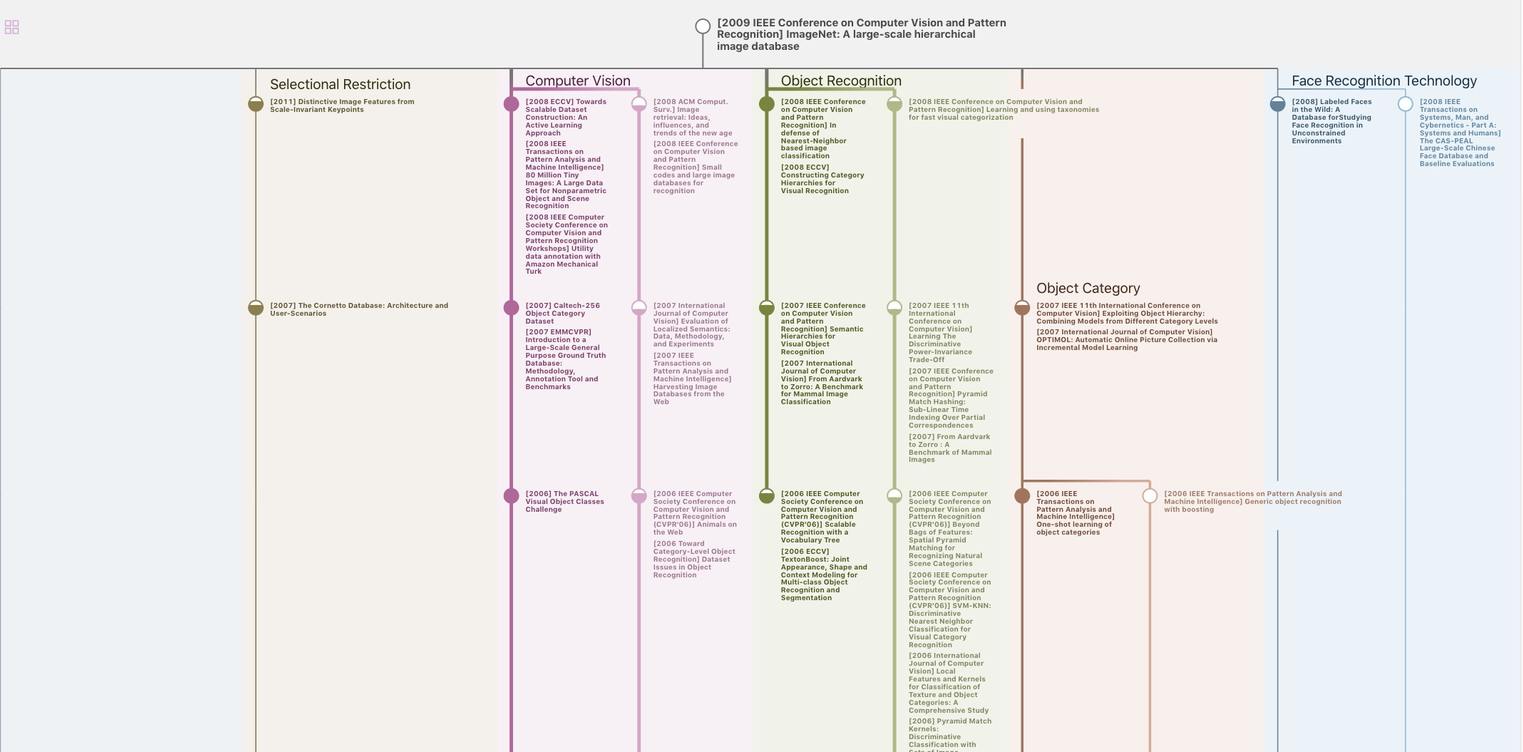
Generate MRT to find the research sequence of this paper
Chat Paper
Summary is being generated by the instructions you defined