Annular-Graph Attention Model for Personalized Sequential Recommendation
IEEE Transactions on Multimedia(2021)
摘要
Sequential recommendations aim to predict the user's next behaviors items based on their successive historical behaviors sequence. It has been widely applied in lots of online services. However, current sequential recommendations use the adjacent behaviors to capture the features of the sequence, ignoring the features among nonadjacent sequential items and the summarized features of the sequence. To address the above problems, in this paper, we propose an annular-graph attention based sequential recommendation (AGSR) model by exploring user's long-term and short-term preferences for the personalized sequential recommendation. For user's short-term preferences, AGSR builds an annular-graph on the sequence of user behavior. Then, AGSR proposes an annular-graph attention applying on the sub annular-graph to explore local features and applying annular-graph attention on entire annular-graph to explore the global features and the skip features. For user's long-term preferences, the latent factor model are introduced in AGSR. The experimental results on two public datasets show that our model outperforms the state-of-the-art methods.
更多查看译文
关键词
Recommender systems,Collaboration,Recurrent neural networks,Deep learning,Computational modeling,Measurement,Markov processes,Attention mechanism,graph attention,personalized recommendation,sequential recommendation,user preferences
AI 理解论文
溯源树
样例
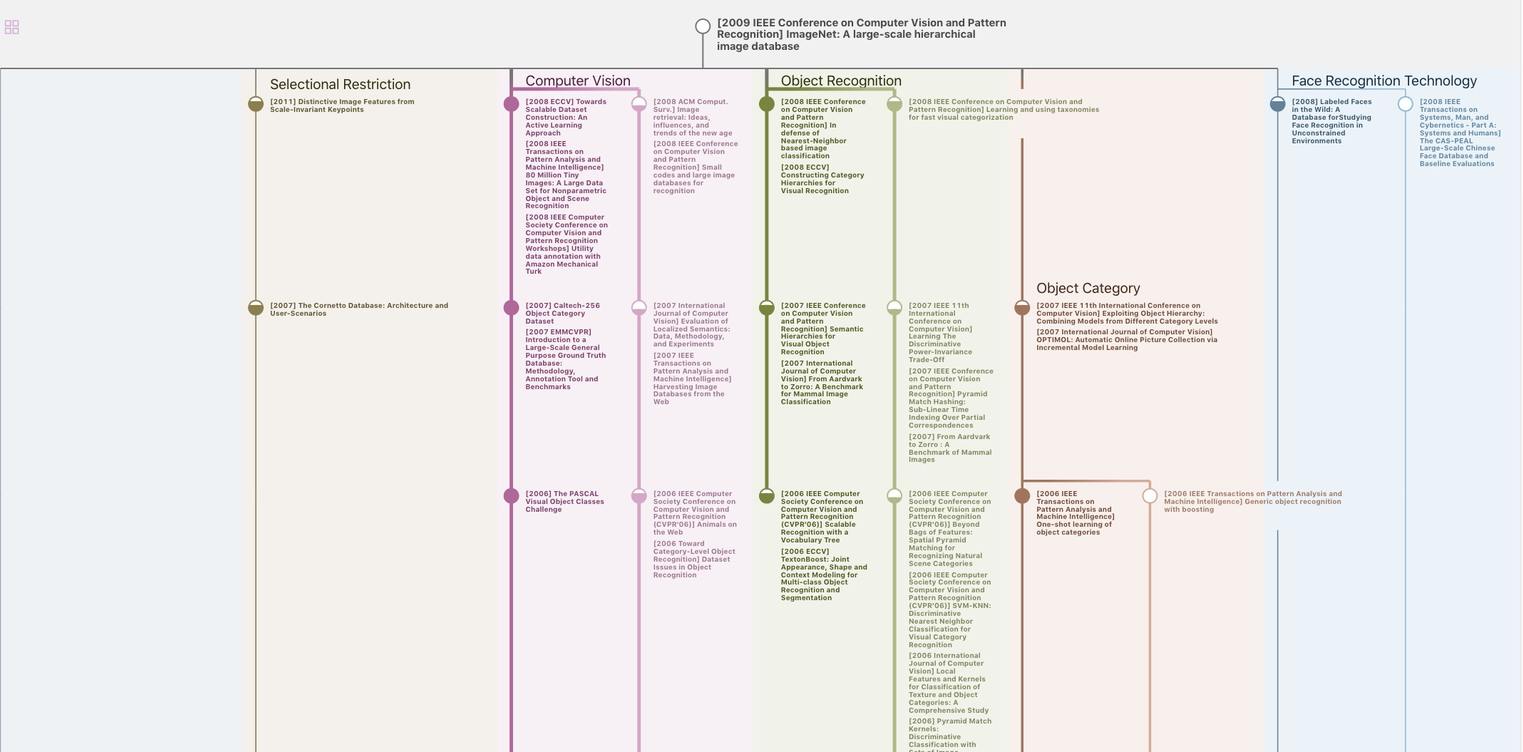
生成溯源树,研究论文发展脉络
Chat Paper
正在生成论文摘要