Deep Encoder With Kernel-Wise Taylor Series for Hyperspectral Image Classification.
IEEE Transactions on Geoscience and Remote Sensing(2022)
摘要
Deep learning is a popular and effective technique for hyperspectral image (HSI) classification. Current deep-learning-based methods have numerous free parameters to be trained. They may be unavailable once lacking training samples. In addition, these approaches only use features from the deepest layer and exclude shallow features, which is a kind of information loss. To remedy such deficiencies, in this work, we construct a novel deep encoder with kernel-wise Taylor series (EKTS) for HSI classification. More specifically, we introduce the Taylor series to approximate the role of deep networks for feature extraction. Because the original Taylor series is linear, the kernel theory is used to build EKTS to encode the HSI data and extract deep nonlinear features. Furthermore, an alternating iterative optimization strategy is developed to obtain the outputs of all the layers of the built deep encoder. Subsequently, we stack the outputs of all the layers to obtain the final features that integrate both shallow and deep features. Finally, support vector machine (SVM) is adopted to deal with the obtained final features so that label results can be predicted. Unlike current deep learning methods, our EKTS has no free parameters to be trained and combines the advantages of both shallow and deep features to predict labels. Sufficient experimental analysis has been performed to verify greater classification performance of our EKTS method compared with many state-of-the-art approaches.
更多查看译文
关键词
Taylor series,Feature extraction,Deep learning,Task analysis,Kernel,Matrix decomposition,Support vector machines,Deep encoder,hyperspectral image (HSI) classification,kernel,Taylor series
AI 理解论文
溯源树
样例
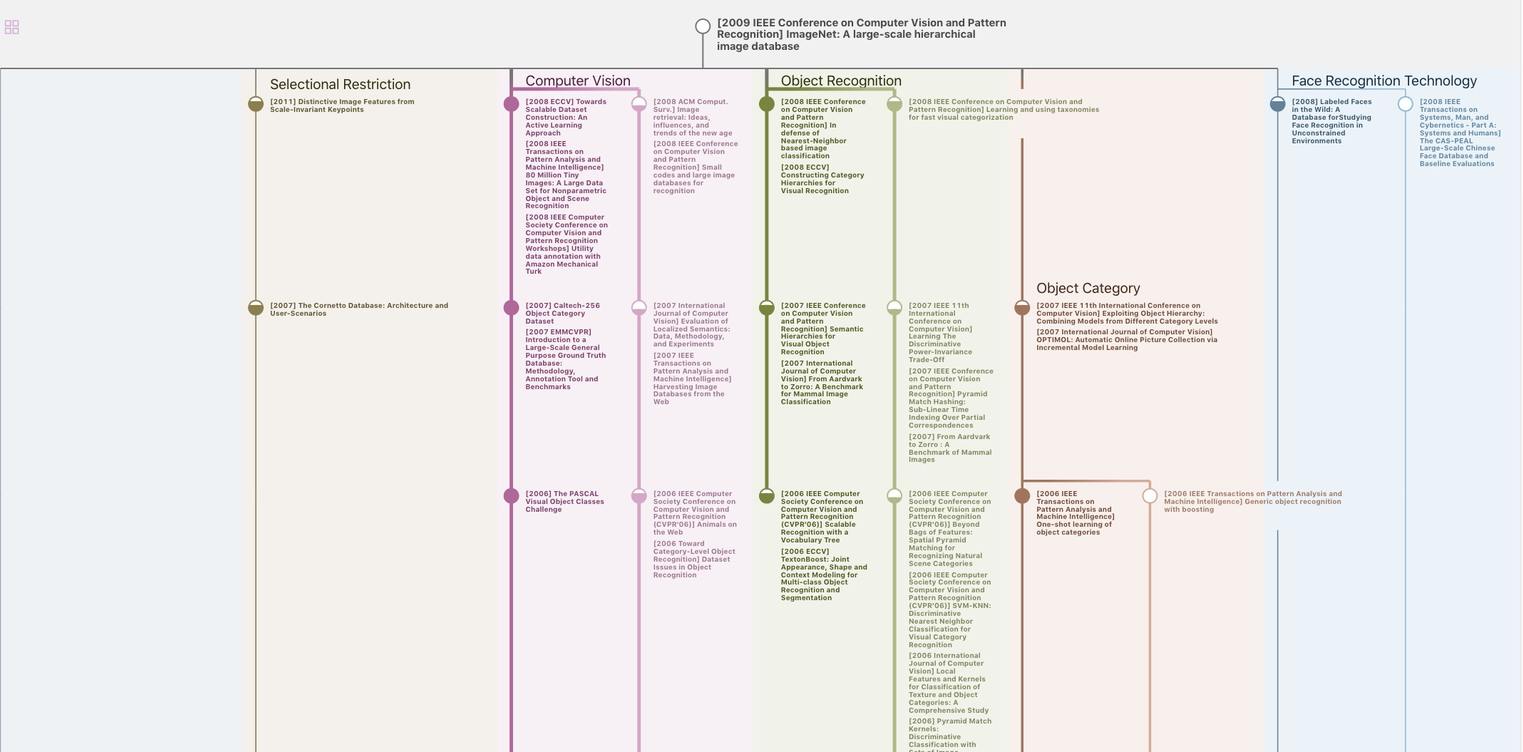
生成溯源树,研究论文发展脉络
Chat Paper
正在生成论文摘要