Privacy-Preserving Contrastive Explanations with Local Foil Trees.
IACR Cryptology ePrint Archive(2022)
摘要
We present the first algorithm that combines privacy-preserving technologies and state-of-the-art explainable AI to enable privacy-friendly explanations of black-box AI models. We provide a secure algorithm for contrastive explanations of black-box machine learning models that securely trains and uses local foil trees. Our work shows that the quality of these explanations can be upheld whilst ensuring the privacy of both the training data, and the model itself. An extended version of this paper is found at Cryptology ePrint Archive [16].
更多查看译文
关键词
Explainable AI,Secure multi-party computation,Decision tree,Foil tree
AI 理解论文
溯源树
样例
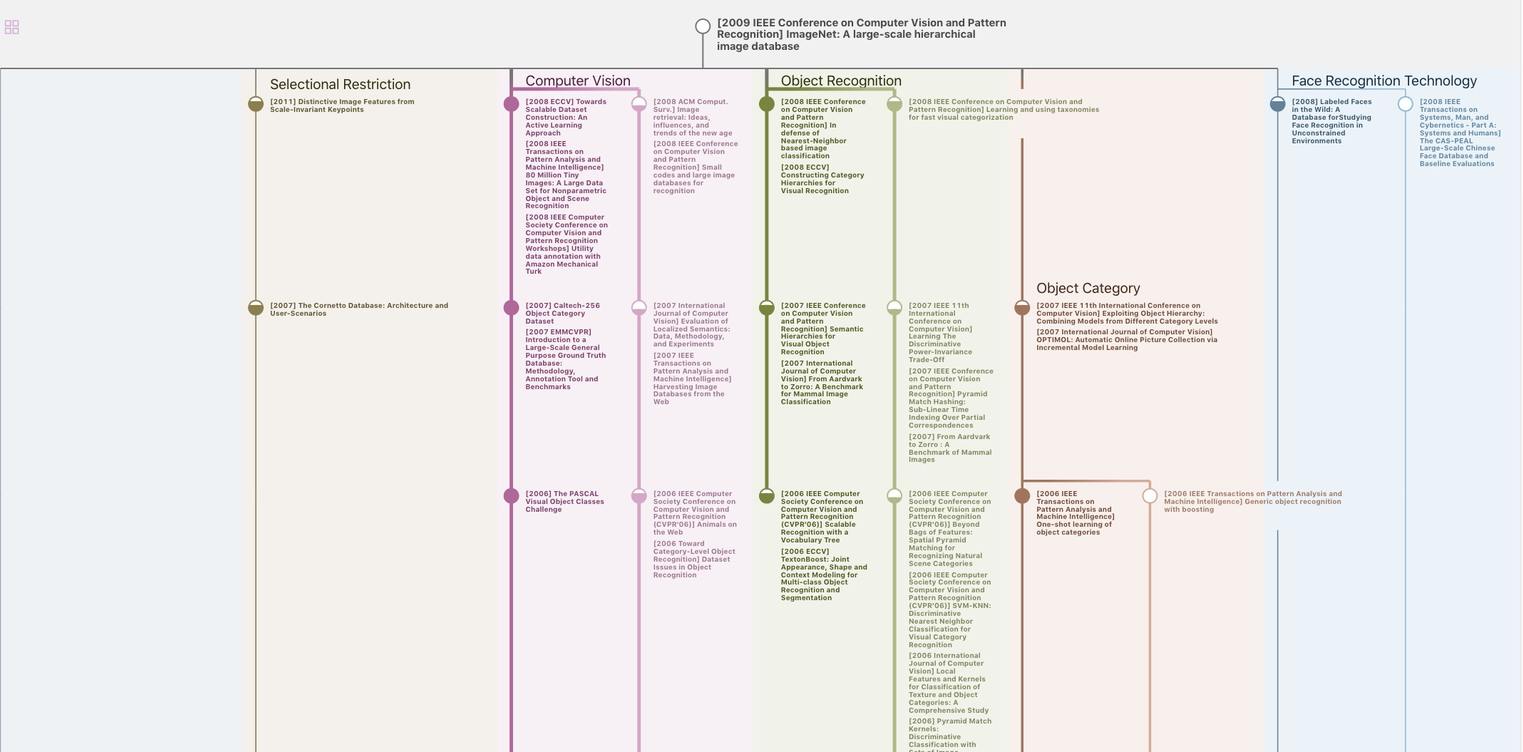
生成溯源树,研究论文发展脉络
Chat Paper
正在生成论文摘要