Leveraging Readability and Sentiment in Spam Review Filtering Using Transformer Models.
Computer Systems: Science & Engineering(2023)
摘要
Online reviews significantly influence decision-making in many aspects of society. The integrity of internet evaluations is crucial for both consumers and vendors. This concern necessitates the development of effective fake review detection techniques. The goal of this study is to identify fraudulent text reviews. A comparison is made on shill reviews vs. genuine reviews over sentiment and readability features using semi-supervised language processing methods with a labeled and balanced Deceptive Opinion dataset. We analyze textual features accessible in internet reviews by merging sentiment mining approaches with readability. Overall, the research improves fake review screening by using various transformer models such as Bidirectional Encoder Representation from Transformers (BERT), Robustly Optimized BERT (Roberta), XLNET (Transformer-XL) and XLM-Roberta (Cross-lingual Language model–Roberta). This proposed research extracts and classifies features from product reviews to increase the effectiveness of review filtering. As evidenced by the investigation, the application of transformer models improves the performance of spam review filtering when related to existing machine learning and deep learning models.
更多查看译文
关键词
spam review filtering,readability,sentiment
AI 理解论文
溯源树
样例
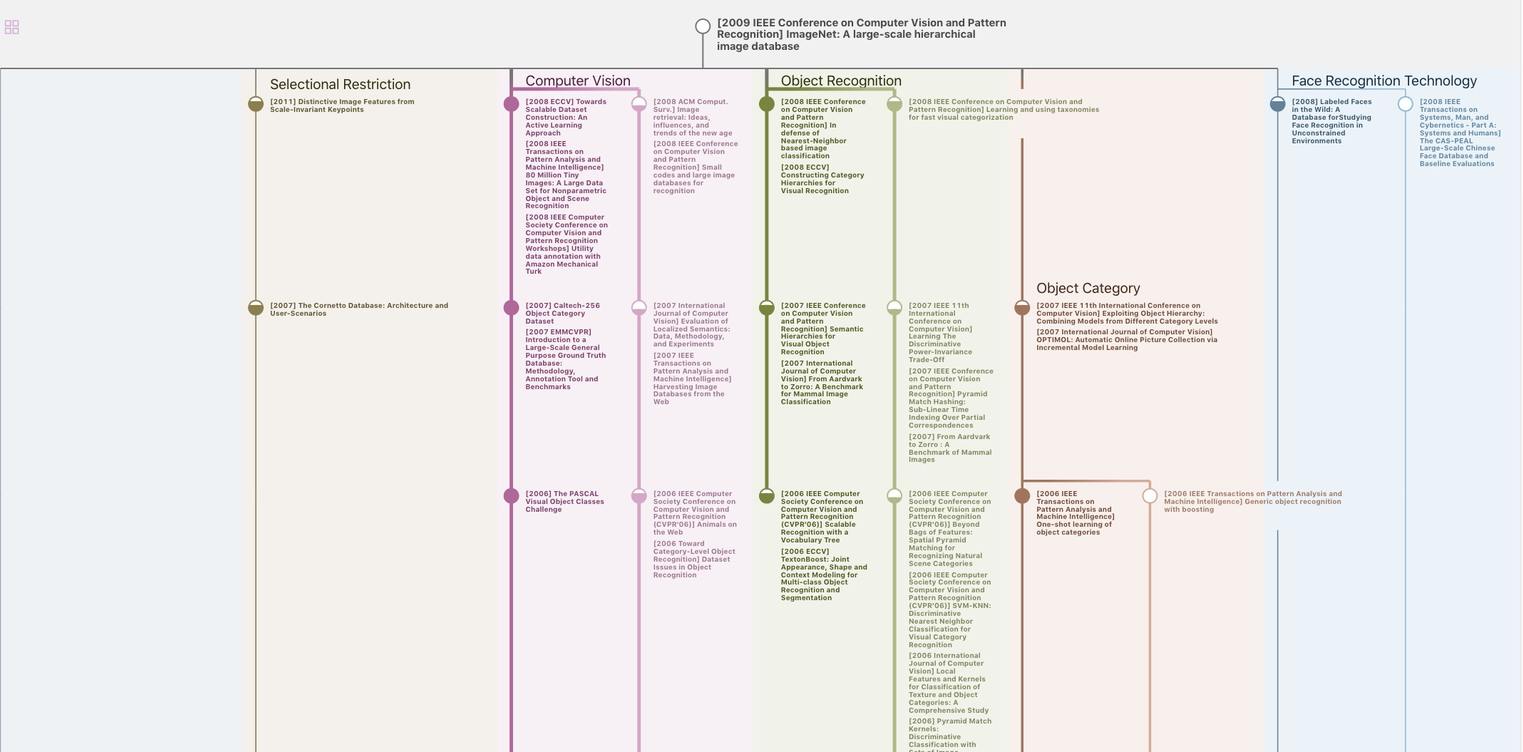
生成溯源树,研究论文发展脉络
Chat Paper
正在生成论文摘要