Sea-Land Segmentation of Remote Sensing Images Based on SDW-UNet.
Computer Systems: Science & Engineering(2023)
摘要
Image segmentation of sea-land remote sensing images is of great importance for downstream applications including shoreline extraction, the monitoring of near-shore marine environment, and near-shore target recognition. To mitigate large number of parameters and improve the segmentation accuracy, we propose a new Squeeze-Depth-Wise UNet (SDW-UNet) deep learning model for sea-land remote sensing image segmentation. The proposed SDW-UNet model leverages the squeeze-excitation and depth-wise separable convolution to construct new convolution modules, which enhance the model capacity in combining multiple channels and reduces the model parameters. We further explore the effect of position-encoded information in NLP (Natural Language Processing) domain on sea-land segmentation task. We have conducted extensive experiments to compare the proposed network with the mainstream segmentation network in terms of accuracy, the number of parameters and the time cost for prediction. The test results on remote sensing data sets of Guam, Okinawa, Taiwan, San Diego, and Diego Garcia demonstrate the effectiveness of SDW-UNet in recognizing different types of sea-land areas with a smaller number of parameters, reduces prediction time cost and improves performance over other mainstream segmentation models. We also show that the position encoding can further improve the accuracy of model segmentation.
更多查看译文
关键词
remote sensing images,remote sensing,sea-land,sdw-unet
AI 理解论文
溯源树
样例
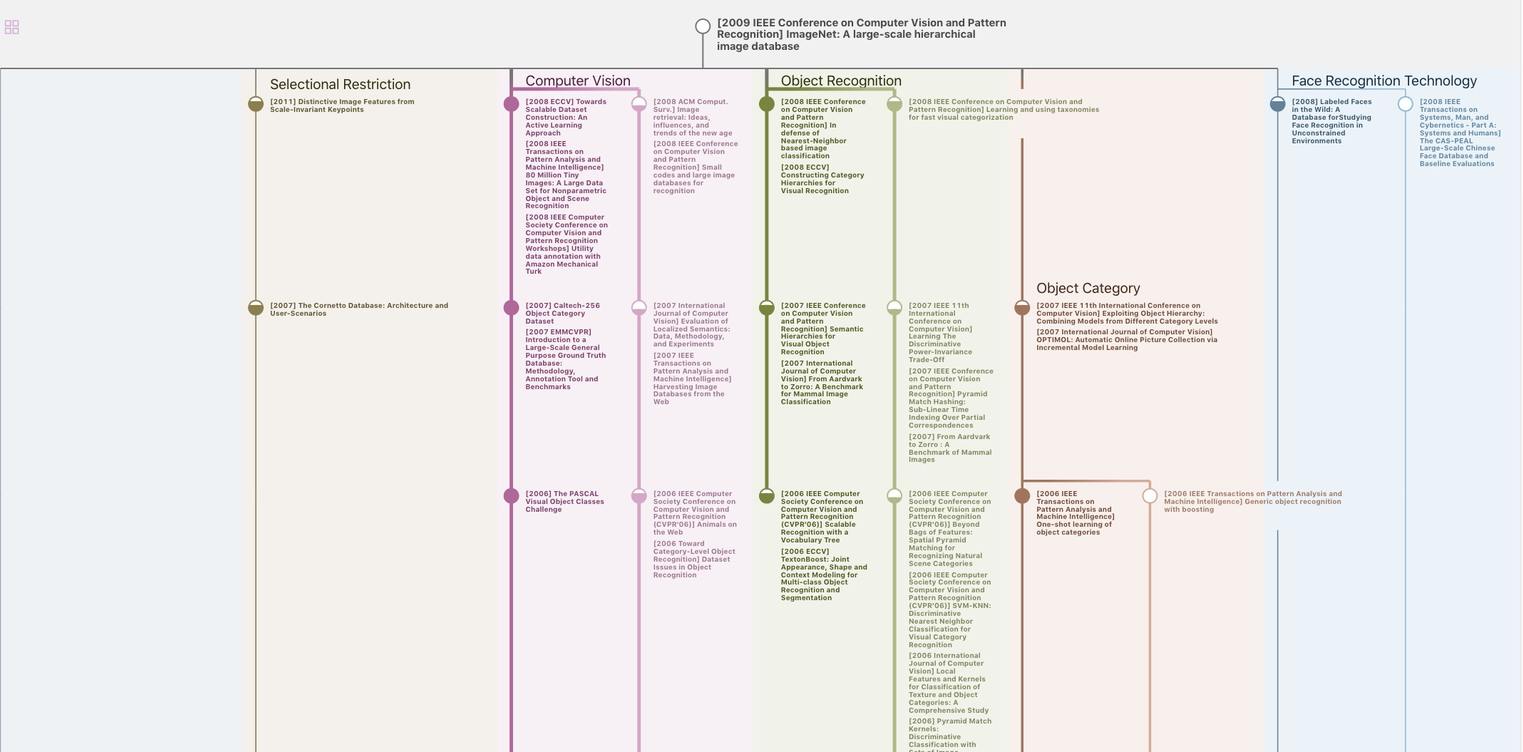
生成溯源树,研究论文发展脉络
Chat Paper
正在生成论文摘要