A Huanglongbing Detection Method for Orange Trees Based on Deep Neural Networks and Transfer Learning.
IEEE Access(2022)
摘要
Huanglongbing (HLB) is one of the most threatening diseases for citrus production and it has caused significant economic damage worldwide. Hence, computer-vision systems that are based on convolutional neural networks (CNNs) can detect HLB accurately. Moreover, the detection system should be able to discriminate between HLB and other citrus abnormalities to ensure that any treatments are effective. Besides, the causal pathogen of HLB is usually detected and diagnosed by the quantitative real-time polymerase chain reaction (qPCR) test, which is costly. Consequently, it is difficult to collect large datasets to train CNN-based systems. In this case, transfer learning from pre-trained CNNs is a solution for building an HLB-detection system using small-sized datasets. This paper evaluates two kinds of CNN architectures: series network (represented by AlexNet, VGG16, and VGG19 models) and directed acyclic graph (DAG) network (represented by ResNet18, GoogLeNet, and Inception-V3 models). These pre-trained CNNs are fine-tuned to distinguish HLB, healthy cases, and 10 kinds of abnormalities of the Citrus sinensis species, which is commonly known as sweet orange. The dataset includes 953 color images, where the leaf samples were collected from orange groves in north Mexico. The 10-fold cross-validation results show that all the CNNs present a 95% or higher HLB sensitivity. However, the number of trainable parameters impacts HLB detection more than the network's depth. Specifically, VGG19, with 19 layers and 144 M parameters, reached a perfect sensitivity for all cross-validation experiments; whereas Inception-V3, with 48 layers and 24 M parameters, reached 95% sensitivity to HLB detection. This outcome happens because a higher number of parameters compensates for the limited number of HLB cases, so VGG19 can successfully transfer the learned characteristics to new cases. This study gives guidance when choosing an adequate CNN to efficiently detect HLB and other orange abnormalities. Besides, a detection scheme is proposed to be further implemented in a portable system to detect HLB in situ, potentially helping to reduce economic losses for small growers from low-income regions.
更多查看译文
关键词
Diseases,Image color analysis,Feature extraction,Cameras,Support vector machines,Convolutional neural networks,Transfer learning,Data models,Crops,Agriculture,Pathogens,Directed acyclic graph,Biological system modeling,Economics,Huanglongbing detection,Citrus sinensis orange,convolutional neural networks,transfer learning,hand-crafted features
AI 理解论文
溯源树
样例
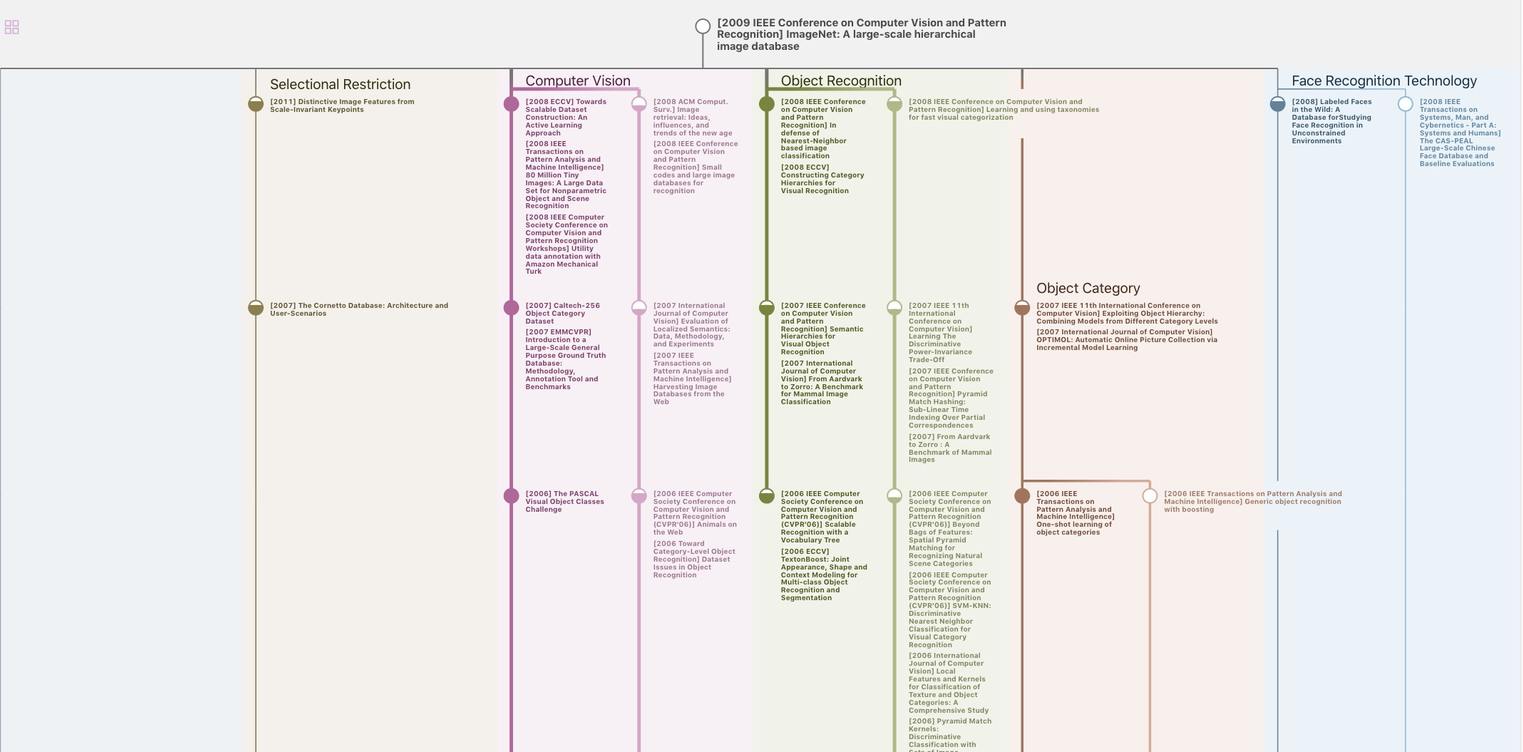
生成溯源树,研究论文发展脉络
Chat Paper
正在生成论文摘要