Lightweight and Effective Tensor Sensitivity for Atomistic Neural Networks
arxiv(2023)
摘要
Atomistic machine learning focuses on the creation of models which obey fundamental symmetries of atomistic configurations, such as permutation, translation, and rotation invariances. In many of these schemes, translation and rotation invariance are achieved by building on scalar invariants, e.g., distances between atom pairs. There is growing interest in molecular representations that work internally with higher rank rotational tensors, e.g., vector displacements between atoms, and tensor products thereof. Here we present a framework for extending the Hierarchically Interacting Particle Neural Network (HIP-NN) with Tensor Sensitivity information (HIP-NN-TS) from each local atomic environment. Crucially, the method employs a weight tying strategy that allows direct incorporation of many-body information while adding very few model parameters. We show that HIP-NN-TS is more accurate than HIP-NN, with negligible increase in parameter count, for several datasets and network sizes. As the dataset becomes more complex, tensor sensitivities provide greater improvements to model accuracy. In particular, HIP-NN-TS achieves a record mean absolute error of 0.927 kcal/mol for conformational energy variation on the challenging COMP6 benchmark, which includes a broad set of organic molecules. We also compare the computational performance of HIP-NN-TS to HIP-NN and other models in the literature.
更多查看译文
关键词
effective tensor sensitivity,networks
AI 理解论文
溯源树
样例
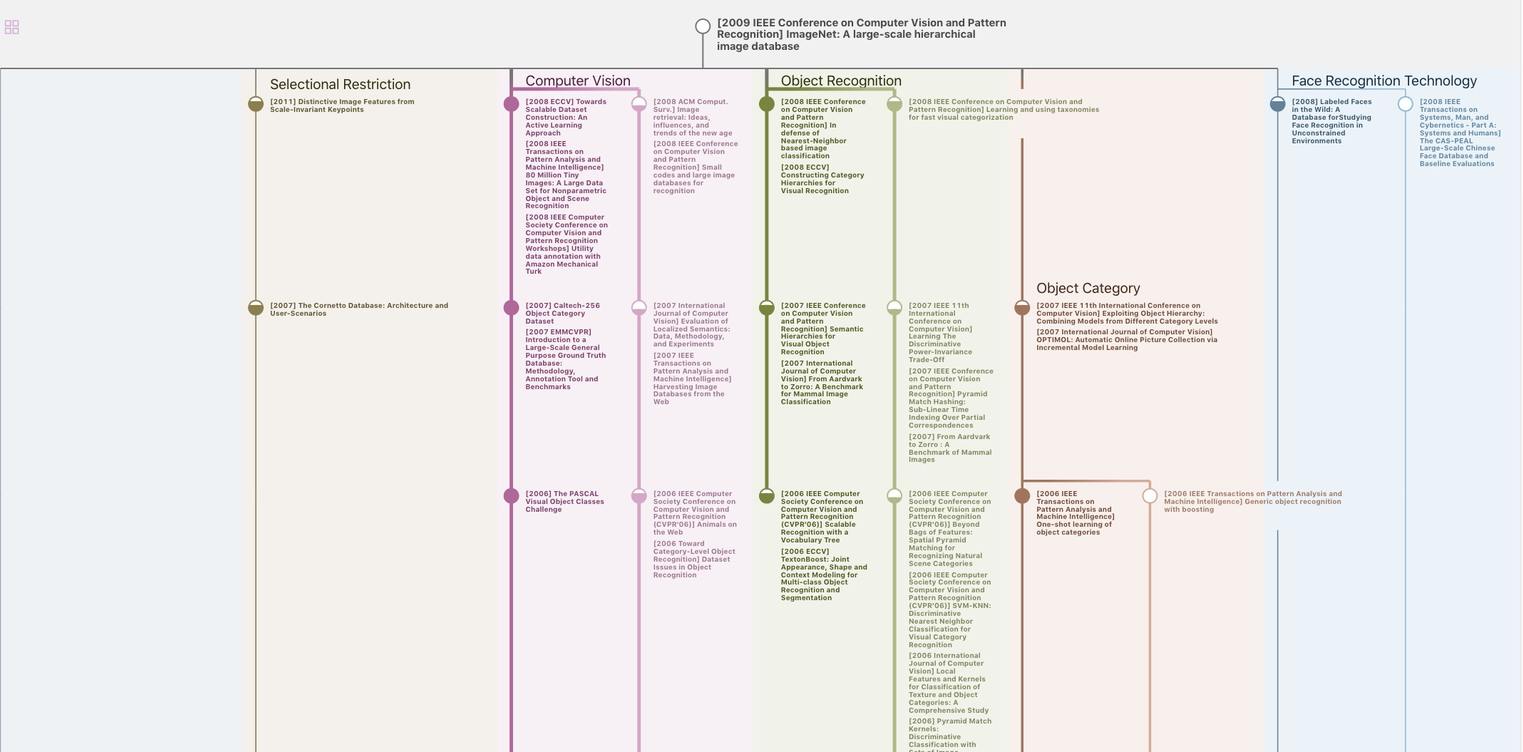
生成溯源树,研究论文发展脉络
Chat Paper
正在生成论文摘要