Benchmarking AutoML algorithms on a collection of synthetic classification problems
CoRR(2023)
Abstract
Automated machine learning (AutoML) algorithms have grown in popularity due to their high performance and flexibility to adapt to different problems and data sets. With the increasing number of AutoML algorithms, deciding which would best suit a given problem becomes increasingly more work. Therefore, it is essential to use complex and challenging benchmarks which would be able to differentiate the AutoML algorithms from each other. This paper compares the performance of four different AutoML algorithms: Tree-based Pipeline Optimization Tool (TPOT), Auto-Sklearn, Auto-Sklearn 2, and H2O AutoML. We use the Diverse and Generative ML benchmark (DIGEN), a diverse set of synthetic datasets derived from generative functions designed to highlight the strengths and weaknesses of the performance of common machine learning algorithms. We confirm that AutoML can identify pipelines that perform well on all included datasets. Most AutoML algorithms performed similarly; however, there were some differences depending on the specific dataset and metric used.
MoreTranslated text
Key words
automl algorithms,benchmarking,classification
AI Read Science
Must-Reading Tree
Example
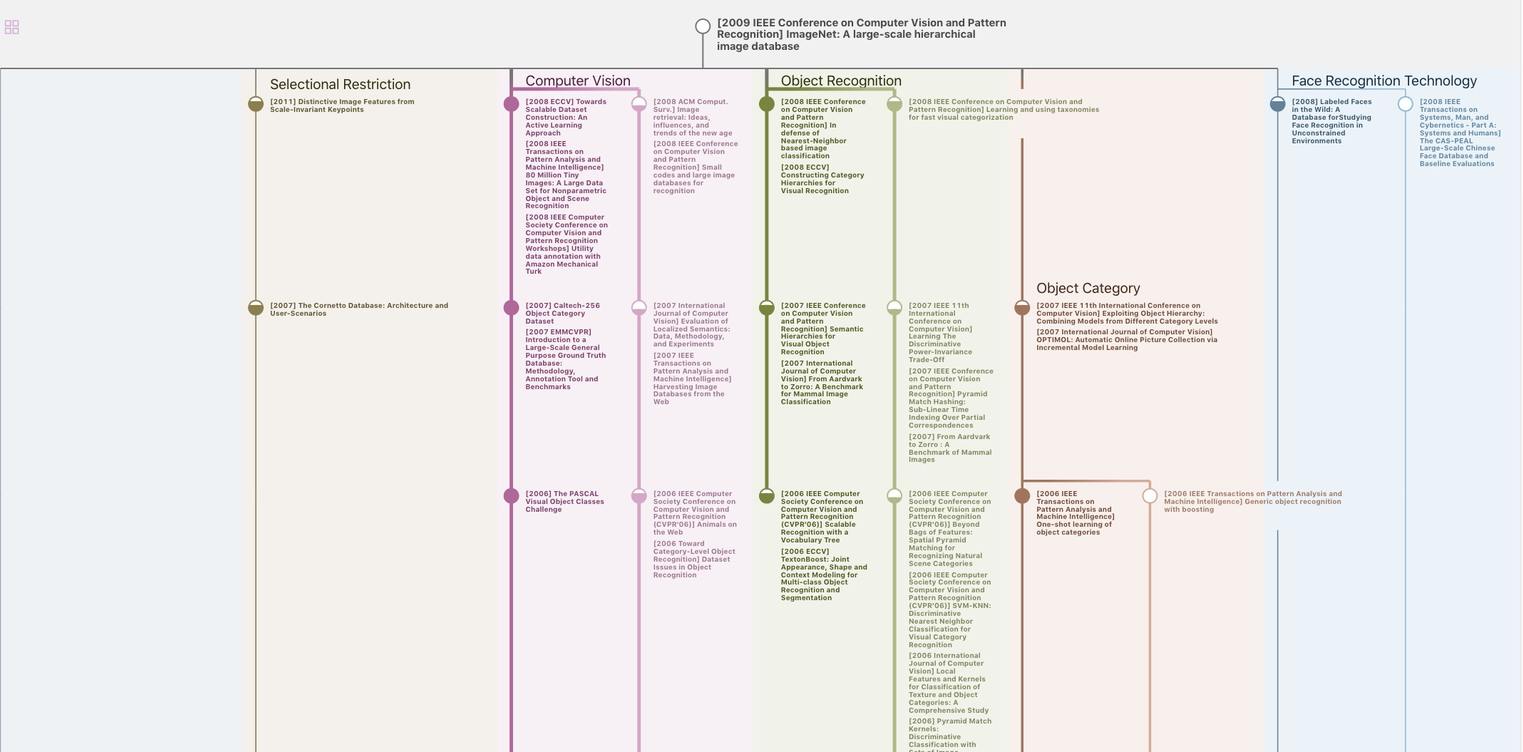
Generate MRT to find the research sequence of this paper
Chat Paper
Summary is being generated by the instructions you defined